Scientists detect 8 strange alien signals originating from nearby stars
New machine learning techniques reveal potential extraterrestrial signals, advancing the search for intelligent life in the universe.
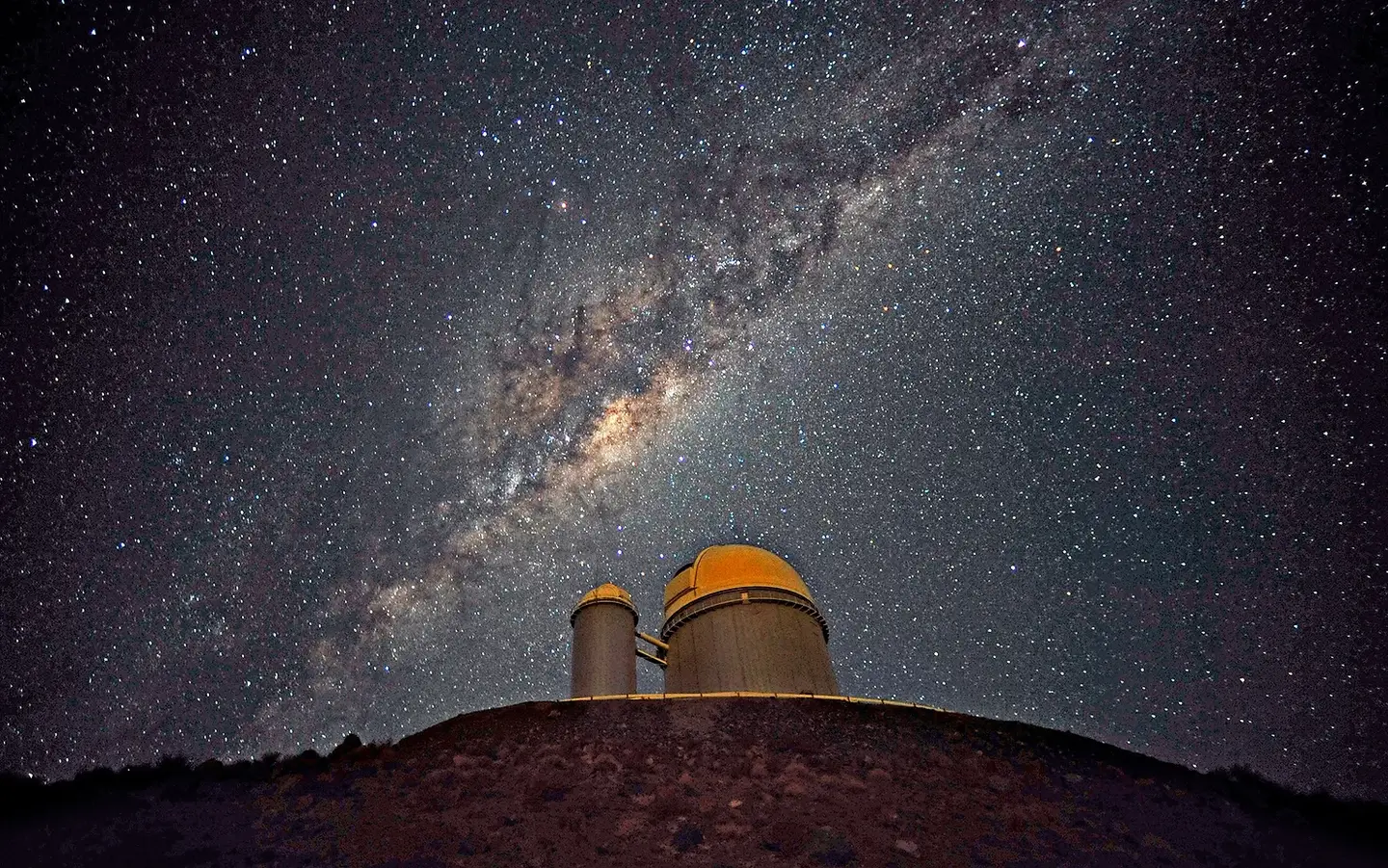
Scientists have harnessed machine learning to uncover potential extraterrestrial signals, transforming the search for intelligent life beyond Earth. (CREDIT: CC BY-SA 4.0)
Are we alone in the universe? This timeless question has driven scientists to explore the cosmos for decades. The search for extraterrestrial intelligence (SETI) focuses on finding evidence of advanced civilizations by identifying "technosignatures"—signals or artifacts produced by alien technologies.
The discovery of such signatures would confirm the existence of intelligent extraterrestrial life, a revelation of profound scientific and philosophical significance.
Historically, radio frequencies have been the primary focus of SETI. Radio waves travel efficiently through space, and the construction of powerful radio transmitters and receivers is feasible.
The most promising technosignatures are narrow-band signals—those confined to a bandwidth of about 1 Hz—and signals exhibiting Doppler shifts caused by relative motion between the transmitter and receiver. These characteristics distinguish potential alien signals from natural astrophysical phenomena. Despite extensive efforts, no definitive technosignature has been identified.
One of the most ambitious efforts in SETI is the Breakthrough Listen (BL) initiative, launched in 2016. Using the Green Bank Telescope (GBT) in the United States and the Parkes "Murriyang" Telescope in Australia, BL has scanned thousands of stars and hundreds of galaxies across multiple frequency bands.
Although these observatories are located in radio-quiet zones, human-generated radio frequency interference (RFI) presents a significant challenge. RFI can mimic potential technosignatures, complicating the task of identifying genuine extraterrestrial signals.
To mitigate RFI, scientists employ spatial filtering techniques such as “cadence” observations or position switching. Genuine extraterrestrial signals should only appear in "on-source" scans, while RFI tends to persist across multiple observations.
Despite these measures, false positives remain common. Previous searches using similar GBT datasets reported tens of millions of “hits”—candidate signals—most of which were ultimately dismissed as interference.
Related Stories
In recent years, advances in machine learning (ML) have revolutionized data analysis in astronomy. ML algorithms can identify patterns and relationships in large datasets, making them ideal for SETI, where data volumes are immense. Researchers have developed ML tools for tasks such as signal classification and anomaly detection. However, a fully ML-driven SETI pipeline had not been achieved until now.
A team of researchers, led by scientists at the University of Toronto, recently unveiled a groundbreaking approach to SETI using advanced ML techniques. Their method combines a type of neural network called β-Variational Autoencoders (β-VAE) with Random Forest decision trees.
The β-VAE framework forces the neural network to learn interpretable features from the data, addressing the "black box" problem often associated with ML models. This design enables the system to identify features such as cadence filtering and narrowband Doppler drifting signals while simultaneously learning the characteristics of RFI.
The researchers applied their ML model to data from the BL initiative, specifically the 1.1–1.9 GHz frequency range observed by the GBT. Analyzing 150 terabytes of data from 820 nearby stars, they examined 57 million unique data snippets, each containing 4096 frequency bins and 16 time bins.
This comprehensive search revealed eight previously undetected signals exhibiting characteristics consistent with technosignatures. These signals were present only when the telescope was directed at the stars and absent when pointed away, suggesting an extraterrestrial origin. Additionally, the signals showed frequency drifts indicative of a source located far from Earth.
The study, published in Nature Astronomy, does not claim to have found evidence of intelligent extraterrestrial life. Instead, it demonstrates the potential of ML to enhance SETI efforts. "I am impressed by how well this approach has performed," said co-author Cherry Ng, an astronomer at the University of Toronto. "With the help of artificial intelligence, I’m optimistic that we’ll be able to better quantify the likelihood of extraterrestrial signals."
The key innovation lies in the use of semi-unsupervised learning, a hybrid approach combining supervised and unsupervised techniques. Supervised learning trains the algorithm using labeled data, while unsupervised learning identifies hidden patterns without explicit guidance. This combination allows the model to generalize from known data and detect novel patterns, improving its ability to distinguish between genuine signals and interference.
The algorithm was developed by Peter Ma, an undergraduate researcher at the University of Toronto. Remarkably, Ma’s interest in SETI began as a high school project.
"I only told my team after the paper’s publication that this all started as a high-school project that wasn’t really appreciated by my teachers," Ma said. His innovative approach has garnered praise from SETI scientists. "New ideas are vital in this field," noted Dr. Ng. "By poking the data with every technique, we might discover exciting signals."
The eight newly identified signals originated from five stars located 30 to 90 light-years from Earth. These stars are relatively close in cosmic terms, making them prime candidates for further investigation. However, the researchers caution that these signals could still have natural or human-made explanations.
"Further observations are necessary to make any claims about extraterrestrial life," said Steve Croft, a project scientist for Breakthrough Listen. "These signals exhibit characteristics we would expect from intelligent origins, but chance cannot be ruled out."
The success of this ML approach has inspired plans to expand its application. The research team aims to analyze data from more powerful telescopes, such as MeerKAT in South Africa and the planned Next Generation Very Large Array. These advanced facilities will allow astronomers to search millions of stars instead of hundreds, significantly increasing the scope of SETI.
"With our new technique, combined with the next generation of telescopes, we hope that machine learning can take us from searching hundreds of stars to searching millions," Ma said. This ambition underscores the transformative potential of ML in SETI, offering a scalable solution to the challenges posed by vast datasets and pervasive interference.
Despite the lack of definitive evidence for extraterrestrial life, the use of AI in SETI represents a significant step forward. The study’s authors remain optimistic that continued advancements in technology and methodology will bring humanity closer to answering one of its most profound questions.
As Dr. Ng put it, "With every new tool we develop, we’re expanding our ability to explore the universe and uncover its mysteries."
Note: Materials provided above by The Brighter Side of News. Content may be edited for style and length.
Like these kind of feel good stories? Get The Brighter Side of News' newsletter.
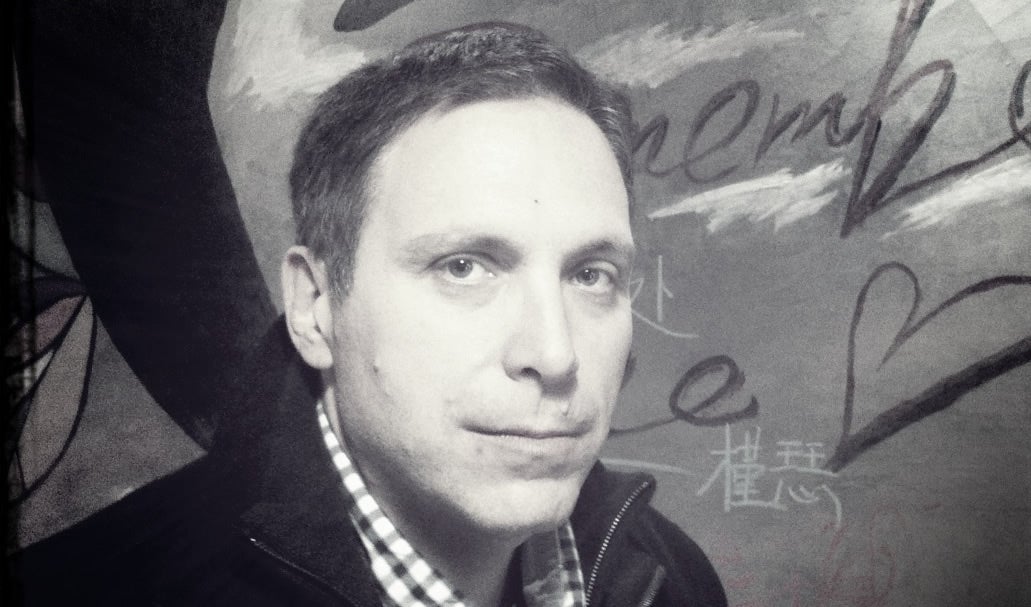