Researchers reveal the fascinating ways AI can mimic the human brain
Not only is the brain great at solving complex problems, it does so while using very little energy.
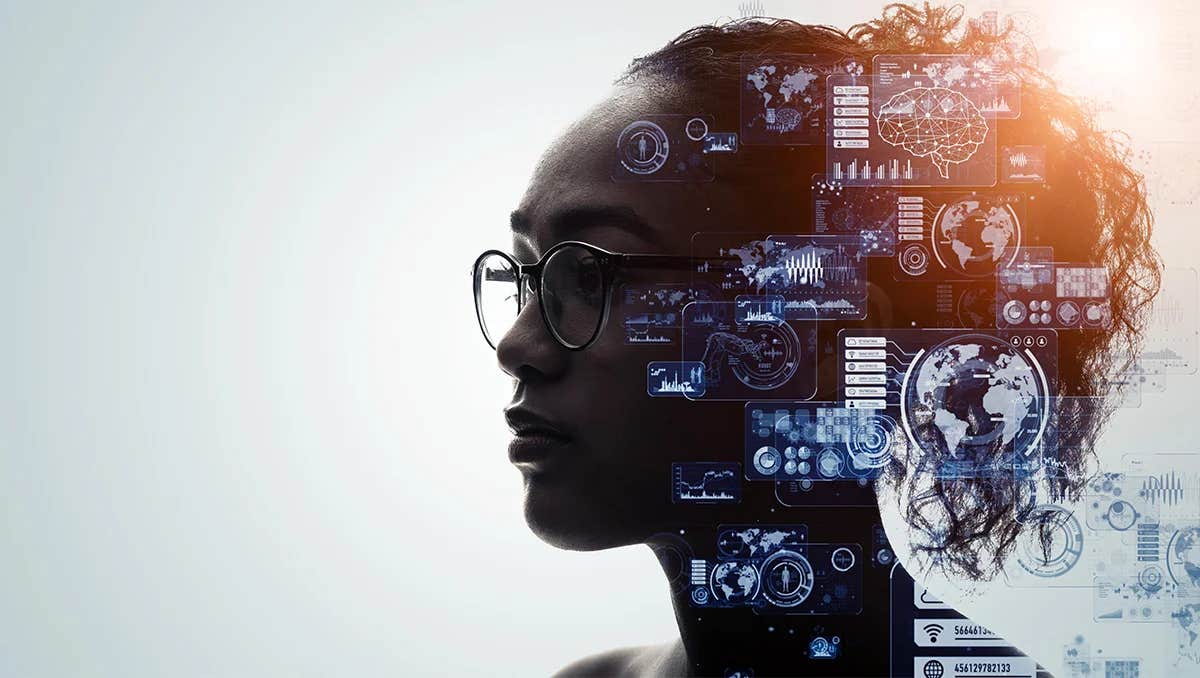
[Nov. 26, 2023: JJ Shavit, The Brighter Side of News]
Not only is the brain great at solving complex problems, it does so while using very little energy. (CREDIT: Creative Commons)
In the intricate dance of evolution, the development of neural systems, such as the human brain, involves a delicate balancing act. These remarkable biological networks must allocate energy and resources efficiently to grow and maintain their physical presence while optimizing their capabilities for information processing.
This inherent trade-off is a fundamental aspect that shapes the structure and function of brains across various species. New research led by Jascha Achterberg and Dr. Danyal Akarca from the Medical Research Council Cognition and Brain Sciences Unit (MRC CBU) at the University of Cambridge explores this intricate interplay between energy conservation and cognitive prowess.
Achterberg, a Gates Scholar, highlights the brain's remarkable abilities, stating, "Not only is the brain great at solving complex problems, it does so while using very little energy. In our new work, we show that considering the brain's problem-solving abilities alongside its goal of spending as few resources as possible can help us understand why brains look like they do."
Dr. Akarca, co-lead author of the study, adds, "This stems from a broad principle, which is that biological systems commonly evolve to make the most of what energetic resources they have available to them. The solutions they come to are often very elegant and reflect the trade-offs between various forces imposed on them."
Related Stories
Published in the prestigious journal Nature Machine Intelligence, the study by Achterberg, Akarca, and their colleagues introduces an artificial neural system designed to model a simplified version of the human brain while imposing physical constraints. In this pioneering study, the researchers set out to uncover whether these constraints would lead the artificial system to develop characteristics and strategies akin to those found in biological brains.
Unlike the biological neurons in the human brain, the artificial system employed computational nodes with similar input-output functionalities. Neurons and nodes share the common purpose of processing information, with each taking input, transforming it, and producing an output. Both neurons and nodes can establish connections with multiple others, facilitating the flow of information within the network.
In this artificial system, the researchers introduced a unique "physical" constraint. Each computational node was assigned a specific location within a virtual space, and, crucially, the distance between two nodes influenced the ease of communication between them. This mimicked the organizational principles seen in the human brain, where proximity plays a role in neural connectivity.
Graphic representing an artificially intelligent brain. (CREDIT: DeltaWorks)
To put their artificial system to the test, the researchers assigned it a seemingly straightforward task—a simplified maze navigation problem, akin to challenges given to animals such as rats and macaques in neuroscience research. This task required the system to integrate multiple pieces of information to determine the shortest path from a starting point to the end.
Initially, the artificial system was unfamiliar with the task and made errors. However, it learned and improved over time through feedback, much like the way real brains adapt and strengthen connections as they learn. The key distinction here was the imposed physical constraint, which made it more challenging for nodes that were physically distant from each other to form connections. In the human brain, establishing connections over long distances is energetically costly.
We use regularization to influence network structure during training to promote smaller network weights and hence a sparser connectome. (CREDIT: Nature Machine Intelligence)
Remarkably, the artificial system, when confronted with these constraints, exhibited behaviors akin to those seen in human brains. It began to develop hubs, highly connected nodes that acted as central channels for information transmission across the network. Furthermore, individual nodes demonstrated flexible coding schemes, meaning they could encode multiple aspects of the maze task simultaneously, a characteristic observed in the brains of complex organisms.
Co-author Professor Duncan Astle from Cambridge's Department of Psychiatry remarks, "This simple constraint—it's harder to wire nodes that are far apart—forces artificial systems to produce some quite complicated characteristics. Interestingly, they are characteristics shared by biological systems like the human brain. I think that tells us something fundamental about why our brains are organized the way they are."
An example of a representative seRNN network. The colour of the nodes relates to the decoding preference of that neuron, where a preference for goal information is represented by green and choices information by brown. (CREDIT: Nature Machine Intelligence)
The study's implications extend beyond a mere understanding of brain organization. The researchers hope that their artificial intelligence system can provide insights into how such constraints contribute to individual differences in human brains and may play a role in cognitive and mental health disorders.
Co-author Professor John Duncan from the MRC CBU notes, "These artificial brains give us a way to understand the rich and bewildering data we see when the activity of real neurons is recorded in real brains."
Artificial neural systems, such as the one created in this study, have the potential to answer questions that would be impossible to address in biological systems. By training these systems to perform tasks and experimenting with the constraints imposed upon them, researchers can gain valuable insights into the intricacies of neural evolution.
The white borders within the regularization-training parameter space delineate the conditions where seRNNs achieve robust accuracy (left), sparse connectivity (middle left), modular networks (middle right) and small-worldness (right). The pink box shows where all these findings can be found simultaneously. The colour of the matrix corresponds to the relative magnitude of the statistic compared with the maximum. (CREDIT: Nature Machine Intelligence)
The findings also hold significant promise for the field of artificial intelligence (AI). Dr. Akarca emphasizes, "AI researchers are constantly trying to work out how to make complex, neural systems that can encode and perform in a flexible way that is efficient. To achieve this, we think that neurobiology will give us a lot of inspiration. For example, the overall wiring cost of the system we've created is much lower than you would find in a typical AI system."
In the world of AI, many solutions utilize architectures that bear only a superficial resemblance to the human brain. However, this research suggests that the specific problem a given AI system is tasked with solving could influence the most effective architecture to employ.
Achterberg concludes, "If you want to build an artificially-intelligent system that solves similar problems to humans, then ultimately the system will end up looking much closer to an actual brain than systems running on large compute clusters that specialize in very different tasks to those carried out by humans. The architecture and structure we see in our artificial 'brain' is there because it is beneficial for handling the specific brain-like challenges it faces."
These insights could lead to the development of more efficient AI systems, particularly in scenarios where physical constraints come into play. Robots operating in dynamic environments with limited energy resources, for instance, may benefit from brain-like structures that optimize the balance between information processing and energetic constraints.
Achterberg adds, "Brains of robots that are deployed in the real physical world are probably going to look more like our brains because they might face the same challenges as us. They need to constantly process new information coming in through their sensors while controlling their bodies to move through space towards a goal. Many systems will need to run all their computations with a limited supply of electric energy, and so, to balance these energetic constraints with the amount of information it needs to process, it will probably need a brain structure similar to ours."
In the ever-evolving landscape of artificial intelligence, this study underscores the importance of drawing inspiration from the elegant solutions that have evolved in biological systems over millennia. As researchers continue to uncover the secrets of the brain, the potential for more efficient and brain-inspired AI systems continues to expand, offering exciting prospects for the future of technology and cognition.
Note: Materials provided above by The Brighter Side of News. Content may be edited for style and length.
Like these kind of feel good stories? Get the Brighter Side of News' newsletter.
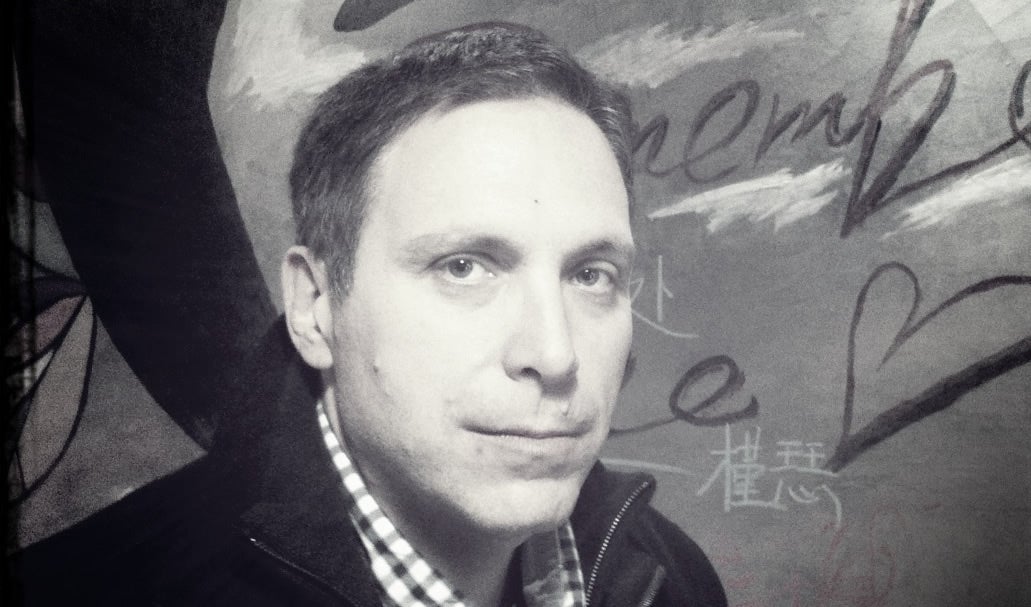
Joseph Shavit
Head Science News Writer | Communicating Innovation & Discovery
Based in Los Angeles, Joseph Shavit is an accomplished science journalist, head science news writer and co-founder at The Brighter Side of News, where he translates cutting-edge discoveries into compelling stories for a broad audience. With a strong background spanning science, business, product management, media leadership, and entrepreneurship, Joseph brings a unique perspective to science communication. His expertise allows him to uncover the intersection of technological advancements and market potential, shedding light on how groundbreaking research evolves into transformative products and industries.