Powerful new AI tool helps fact-checkers battle election misinformation
Researchers develop AI-powered tool to help fact-checkers combat election misinformation, reducing costs and improving accuracy.
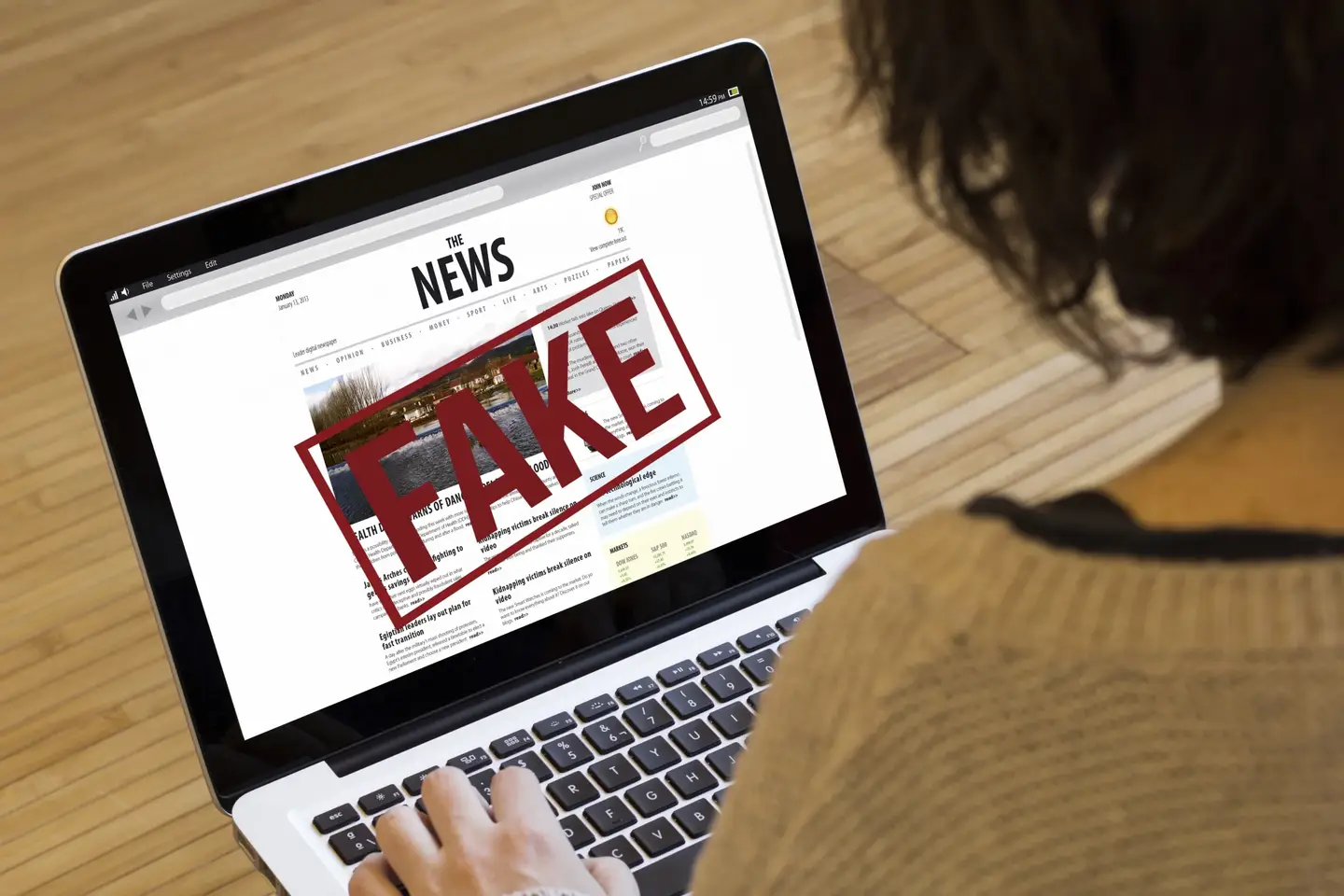
AI system helps fact-checkers tackle election misinformation, boosting efficiency and cutting costs. (CREDIT: CC BY-SA 3.0)
In the midst of an intense election season, the rising tide of misinformation and conspiracy theories poses a significant challenge. With fake news aiming to sway public opinion and confuse voters, the demand for efficient fact-checking is greater than ever.
Researchers at Ben-Gurion University of the Negev (BGU) are addressing this issue with a new tool designed to assist fact-checkers by automating the identification of fake news sources. This approach, developed by a team led by Dr. Nir Grinberg and Prof. Rami Puzis, has the potential to alleviate the overwhelming burden on human fact-checkers, particularly as misinformation spreads rapidly on social media.
In a study recently published in Proceedings of the 30th ACM SIGKDD Conference on Knowledge Discovery and Data Mining, the BGU researchers propose a machine learning-based system that tracks patterns across fake news sources, rather than focusing on individual articles or social media posts.
Dr. Grinberg explains, “The problem today with the proliferation of fake news is that fact-checkers are overwhelmed. They cannot fact-check everything, but the breadth of their coverage amidst a sea of social media content and user flags is unclear. Moreover, we know little about how successful fact-checkers are in getting to the most important content to fact-check.”
Current methods in fact-checking generally target users sharing misinformation or specific flagged posts, which limits the scope and effectiveness of spotting emerging fake news sources. However, fake news sites often disappear and reappear in new forms, making it challenging to track misinformation at the source level.
The BGU team’s model seeks to overcome this by identifying clusters of fake news sources and focusing on the flow of information and social media users’ interactions with these sources. The system is designed to evolve with shifting patterns in misinformation, allowing it to adapt to new sites and platforms more reliably over time.
Related Stories
The researchers’ findings reveal that their audience-based model outperforms conventional methods significantly. By observing the behavior of audiences who frequently engage with fake news, the team achieved a 33% improvement in identifying fake news sources when analyzing historical data and a 69% improvement when tracking sources in real time.
The model can also maintain similar accuracy levels with only a fraction of the costs associated with traditional fact-checking approaches. This breakthrough could mark a turning point in the fight against misinformation, particularly during election seasons.
While this system shows promise, Dr. Grinberg is clear that it should complement—not replace—human fact-checkers. “It can greatly expand the coverage of today’s fact checkers,” he says, noting that a collaborative approach would optimize the system’s effectiveness.
The team includes additional expertise from Maor Reuben, also from BGU’s Department of Software and Information Systems Engineering, and independent researcher Lisa Friedland, whose collective efforts highlight the interdisciplinary nature of this innovation.
One remaining question is whether social media platforms will support this initiative by providing the necessary access to data. Without cooperation from these platforms, deploying this technology broadly remains challenging.
Social media companies hold key data that could amplify this system’s impact and make it easier for fact-checkers to tackle misinformation head-on. The research team hopes this project will encourage platforms to work alongside academic researchers, policymakers, and fact-checking organizations to bring this tool to fruition.
The BGU team’s approach represents a proactive step toward minimizing the impact of misinformation on public discourse. Their machine learning model doesn’t just identify fake news sources more effectively but also significantly reduces the cost and time required to do so.
In a landscape where misinformation threatens the democratic process, innovations like this could empower fact-checkers to work more efficiently, ultimately helping voters make more informed choices.
Note: Materials provided above by The Brighter Side of News. Content may be edited for style and length.
Like these kind of feel good stories? Get The Brighter Side of News' newsletter.
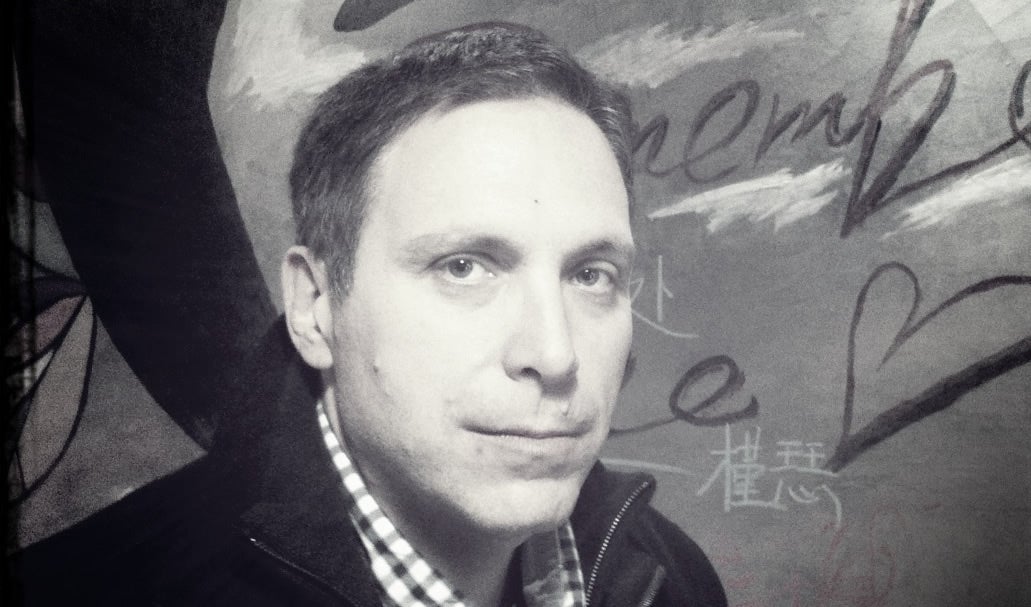