Powerful AI tongue can assess food safety with over 80% accuracy
Learn how graphene-based ISFETs and machine learning are transforming chemical sensing for food safety, healthcare, and environmental monitoring.
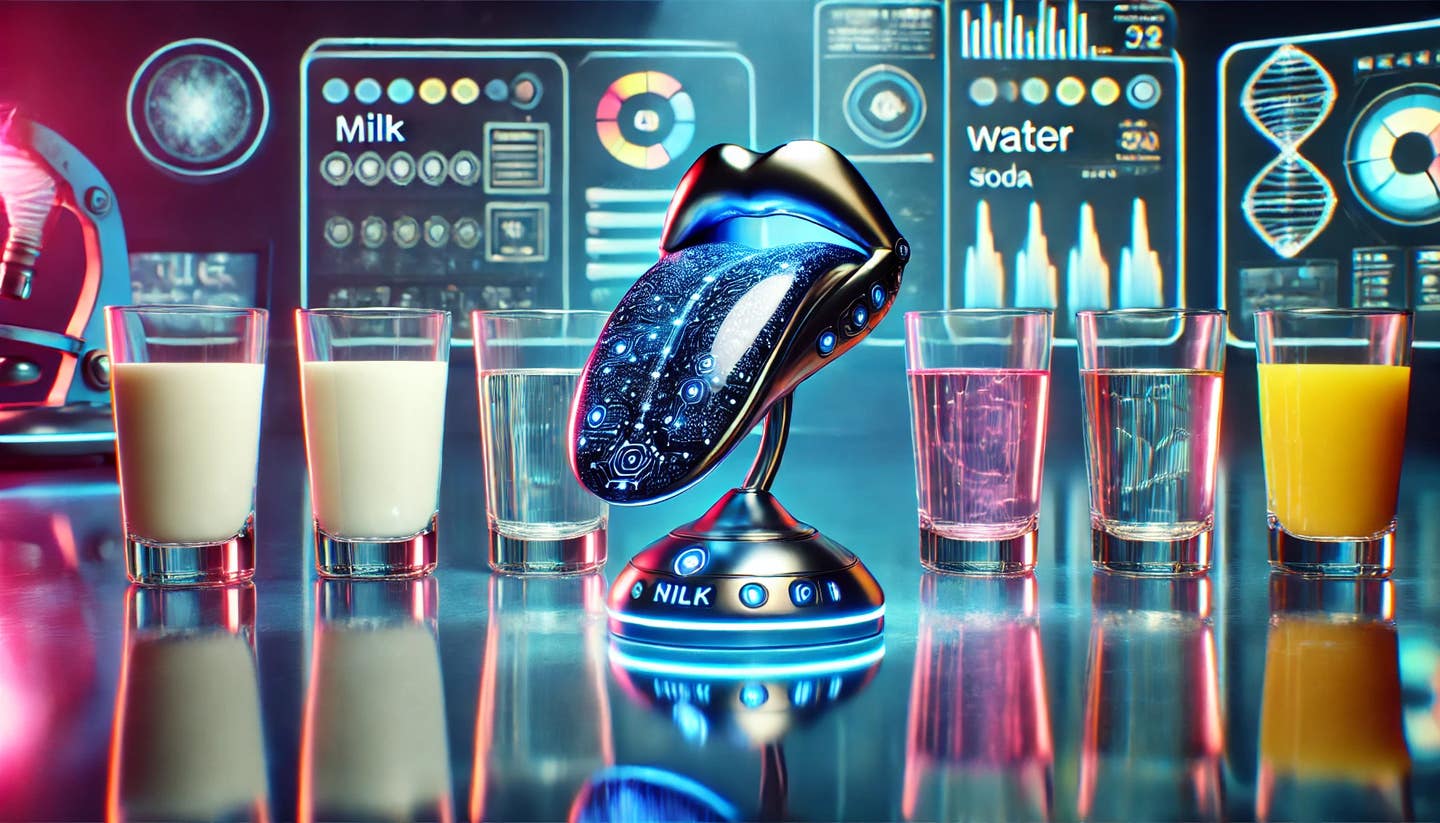
Researchers successfully applied this system to identify different types of milk, sodas, coffee blends, and fruit juices. (CREDIT: The Brighter Side of News / AI-generated)
Chemosensors, which translate the chemical composition of liquids into electrical signals, play a pivotal role in diverse fields. They are critical in environmental monitoring, healthcare diagnostics, and industrial process control. Among these, ion-sensitive field-effect transistors (ISFETs) have emerged as highly sensitive and scalable tools.
However, challenges like sensor variability and manufacturing inconsistencies hinder their widespread adoption. Recent innovations in graphene-based ISFETs and machine learning (ML) have shown promise in overcoming these barriers, unlocking potential applications in areas such as food safety and medical diagnostics.
Since their inception in the 1970s, ISFETs have seen remarkable evolution. Initially demonstrated using silicon to detect sodium ions, ISFETs have incorporated advanced materials like carbon nanotubes and graphene. Graphene stands out due to its high carrier mobility, chemical inertness, and compatibility with functionalization techniques.
These attributes make it an ideal candidate for building highly sensitive and selective ISFET sensors. Arrays of graphene ISFETs have been developed to detect ions like potassium, sodium, calcium, and ammonium, demonstrating their potential for multiplexed chemical sensing.
However, the reliability of ISFETs has been compromised by factors such as sensor-to-sensor and chip-to-chip variations, stemming from material inconsistencies and environmental influences. Addressing these issues is critical for advancing ISFET technology beyond research laboratories and into commercial applications.
Incorporating machine learning into ISFET data analysis offers a novel approach to mitigate these challenges. Unlike traditional methods that rely on manual calibration, ML models can adapt to variations across different sensors.
Published in the journal Nature, researchers have demonstrated this by using non-functionalized graphene-based ISFETs as testbeds for ML integration. By training artificial neural networks (ANNs) on pH data, the team identified patterns in sensor responses that human-derived metrics could not discern.
The study revealed that ANNs can recognize subtle features in graphene ISFET characteristics, distinguishing between classes of chemical data with high accuracy. This capability eliminates the need for extensive sensor calibration, enabling a single sensor design to function across diverse applications. Moreover, ML enhances quantification tasks by employing gradient-based training, expanding its utility beyond simple classification.
Related Stories
One compelling application of ML-assisted graphene ISFETs lies in the food industry. The technology has been used to authenticate products, detect adulteration, and assess food safety.
For instance, researchers successfully applied this system to identify different types of milk, sodas, coffee blends, and fruit juices. Notably, the sensors could determine both the identity and spoilage status of these liquids with over 80% accuracy in under a minute.
By allowing the neural network to define its own evaluation metrics, accuracy improved further. When raw sensor data were processed directly by the ML model, inference accuracy exceeded 95%.
The neural network's decision-making process was analyzed using Shapley additive explanations, a method rooted in game theory. This provided insights into how the AI prioritized different data inputs, revealing a holistic approach to classification and quantification.
For example, the system could differentiate milk types and detect spoilage by analyzing subtle variations in chemical composition. This holistic assessment mirrors human sensory processes, where nuances in taste and smell guide judgments.
The researchers’ findings highlight the potential of ML-enhanced ISFETs to revolutionize food safety, offering rapid, accurate, and cost-effective solutions.
The benefits of this technology extend beyond food safety. With appropriate training data, graphene-based ISFETs could be adapted for medical diagnostics, environmental monitoring, and industrial applications. Their robustness and adaptability make them suitable for real-world deployment, where conditions often deviate from ideal laboratory settings.
Unlike traditional sensors, these devices do not require identical manufacturing. The ML models compensate for imperfections, producing reliable outputs even with variability in sensor fabrication. This reduces production costs while maintaining performance standards, paving the way for scalable manufacturing.
“We figured out that we can live with imperfection,” said Saptarshi Das, the study’s lead author. “Nature is full of imperfections, yet it makes robust decisions. Our electronic tongue follows a similar principle.”
By mimicking human sensory systems, these sensors offer a glimpse into how AI can bridge the gap between machine precision and human intuition. The integration of compact, energy-efficient graphene ISFETs with robust ML algorithms promises to enhance our ability to detect subtle chemical and environmental changes, providing actionable insights in real time.
As researchers continue to refine this technology, its applications are expected to expand. From ensuring food quality to diagnosing diseases, the fusion of graphene-based ISFETs and machine learning represents a transformative leap forward. This innovation underscores the potential of interdisciplinary approaches in addressing complex challenges, heralding a new era in chemical sensing and beyond.
Note: Materials provided above by The Brighter Side of News. Content may be edited for style and length.
Like these kind of feel good stories? Get The Brighter Side of News' newsletter.
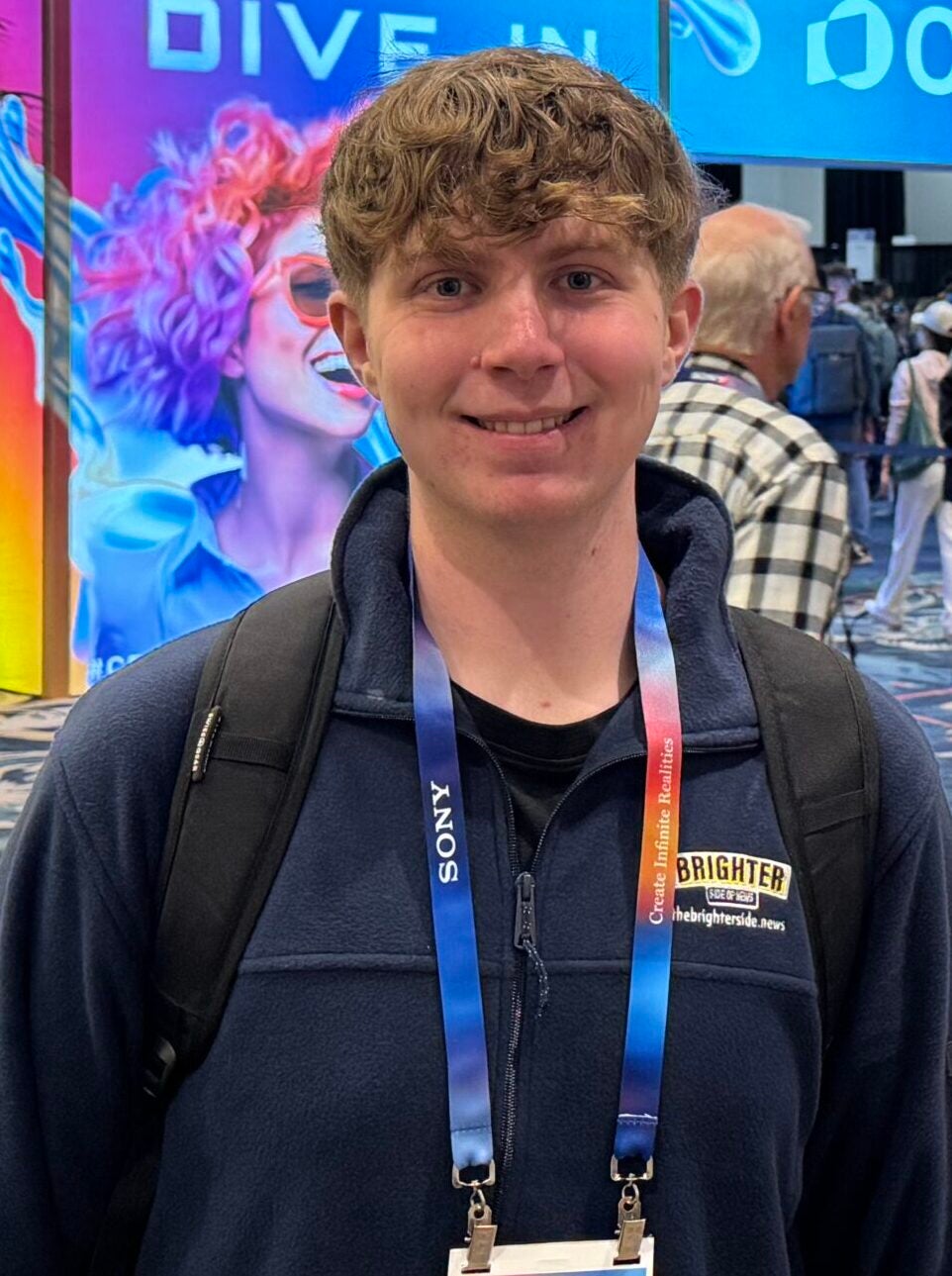
Joshua Shavit
Science & Technology Writer | AI and Robotics Reporter
Joshua Shavit is a Los Angeles-based science and technology writer with a passion for exploring the breakthroughs shaping the future. As a contributor to The Brighter Side of News, he focuses on positive and transformative advancements in AI, technology, physics, engineering, robotics and space science. Joshua is currently working towards a Bachelor of Science in Business Administration at the University of California, Berkeley. He combines his academic background with a talent for storytelling, making complex scientific discoveries engaging and accessible. His work highlights the innovators behind the ideas, bringing readers closer to the people driving progress.