Parkinson’s disease can now be detected with near-perfect accuracy
EEG scans and AI achieve near-perfect accuracy in Parkinson’s detection, unveiling new insights into emotional brain activity.
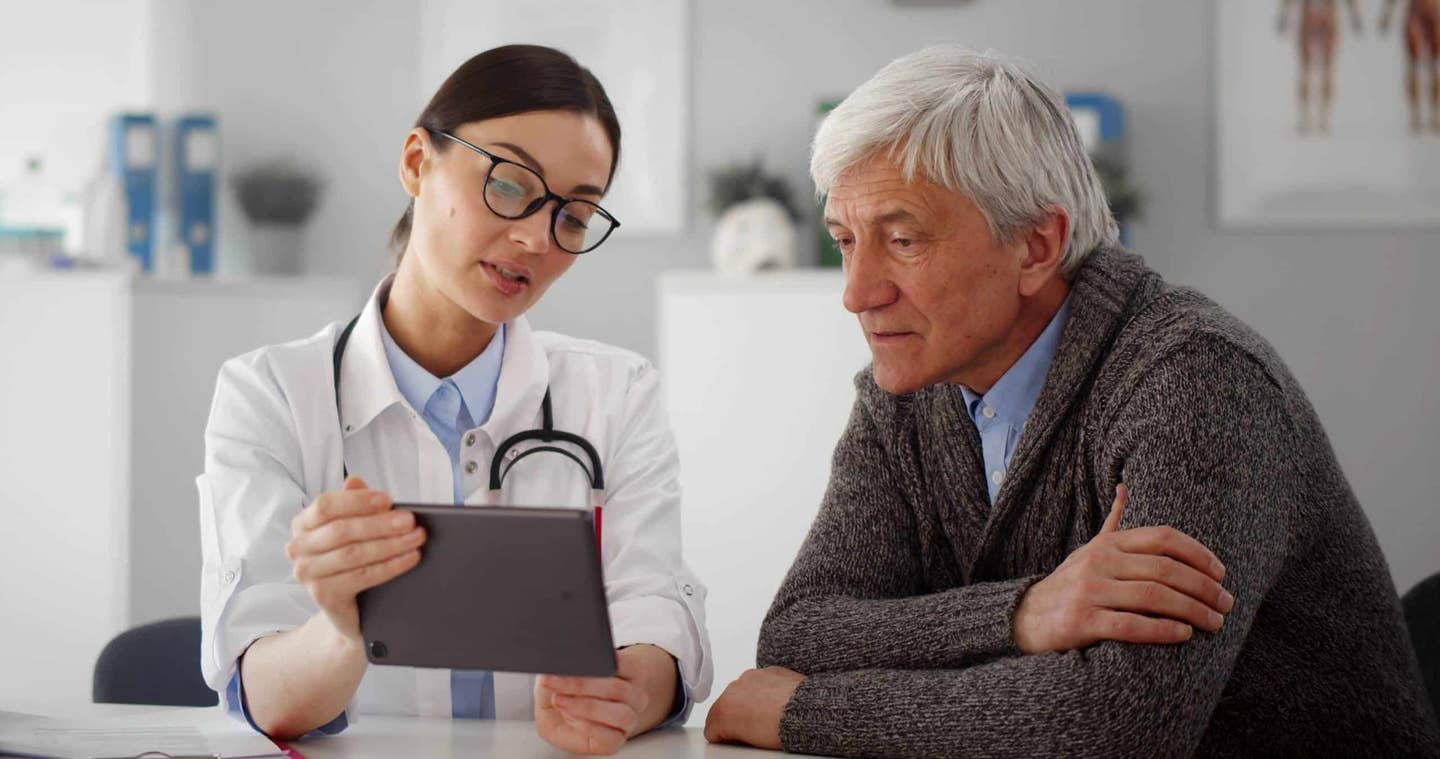
EEG and AI deciphers emotional brain activity, achieving near-perfect accuracy in Parkinson’s diagnosis. (CREDIT: CC BY-SA 4.0)
Parkinson’s disease (PD) is a progressive neurodegenerative disorder affecting over 10 million people worldwide. Its hallmark is the loss of dopamine-producing neurons in the brain’s substantia nigra, leading to tremors, rigidity, and other motor impairments.
Beyond movement issues, PD often disrupts emotional and cognitive functions, complicating patients' ability to recognize and respond to emotions. Addressing these challenges, a recent study unveils a novel diagnostic method leveraging brain responses to emotional stimuli.
A joint research team from the University of Canberra and Kuwait College of Science and Technology has pioneered a way to detect PD with near-perfect accuracy.
By analyzing electroencephalography (EEG) data recorded as participants viewed emotion-inducing video clips and images, researchers achieved diagnostic performance with an F1 score exceeding 0.97. This score—a metric combining precision and recall—signals a breakthrough in non-invasive, objective diagnostics.
Traditional PD diagnoses rely heavily on clinical observations and patient self-reports, which can be subjective. The new EEG-based method bypasses this subjectivity by focusing on implicit emotional brain activity. Unlike explicit responses such as verbal feedback, implicit signals cannot be consciously controlled, providing reliable insights into central nervous system function.
Emotions play a crucial role in communication and social interaction. However, PD patients often struggle to process emotions, particularly those linked to specific valence dimensions (pleasantness or unpleasantness) and arousal levels (emotional intensity).
The study confirmed prior findings that individuals with PD have difficulty recognizing emotions such as fear, disgust, and surprise. They may also confuse emotions of opposite valences, for example, mistaking sadness for happiness.
EEG recordings of 20 PD patients and 20 healthy controls captured brain activity as participants reacted to emotionally evocative stimuli. Researchers extracted key EEG descriptors, including spectral power vectors (SPVs) and common spatial patterns (CSPs).
Related Stories
SPVs measure the power distribution across different frequency bands, which are closely linked to emotional states. CSPs, on the other hand, enhance class discriminability by maximizing variance differences, facilitating precise classification of EEG signals.
These descriptors were converted into visual representations, such as topographical maps and temporal activity patterns. Advanced machine learning frameworks, including convolutional neural networks (CNNs), analyzed these representations to decode emotional responses.
This combination of neurotechnology and artificial intelligence allowed researchers to distinguish PD patients from healthy individuals with unprecedented accuracy.
The study also explored how PD patients perceive emotions. While they showed reduced sensitivity to valence, they exhibited better comprehension of emotional arousal. This indicates a heightened awareness of emotional intensity over the pleasantness or aversion associated with emotions. Such insights highlight the complexity of emotional processing deficits in PD and underscore the potential of EEG-based tools in clinical settings.
Emotion-eliciting stimuli like movies and music have previously proven useful in studying brain activity. However, many earlier approaches relied on resting-state EEG, which requires patients to remain still in controlled environments. This limits ecological validity. By incorporating dynamic, real-world stimuli, the current research achieves more accurate reflections of everyday emotional processing.
The integration of SPVs, CSPs, and CNNs also advances the field of affective computing. Hand-coded EEG descriptors like SPVs have long been used to detect emotions, but CNNs offer automatic learning of cognitive and emotional patterns, enhancing the precision of emotion recognition. For instance, 1D-, 2D-, and 3D-CNNs enable dimensional emotion classification, such as identifying binary valence and arousal states.
One notable finding from the study is the potential to differentiate PD patients based solely on their brain’s emotional activity patterns. This aligns with growing evidence that EEG frequency bands—such as alpha, beta, and gamma waves—correlate with distinct emotional states. By decoding these signals, researchers have uncovered specific neural markers associated with PD, offering a deeper understanding of how the disease alters brain function.
As this technology develops, emotional brain monitoring could transform clinical practice. EEG’s non-invasive nature and high temporal resolution make it an ideal tool for early diagnosis and monitoring of neurological conditions. Moreover, combining EEG data with AI-driven analysis enhances its scalability and applicability in diverse healthcare settings.
The study also underscores the broader implications of emotion-focused diagnostics. Recognizing emotional deficits in PD patients could lead to targeted interventions, improving social interactions and quality of life. Furthermore, the use of machine learning frameworks to analyze neural data represents a significant step forward in personalized medicine, paving the way for tailored treatment strategies.
While the current research involved a relatively small sample size, its findings lay the groundwork for larger-scale studies. Future research could refine these methods, expanding their use to other neurodegenerative diseases and mood disorders. By mapping the neural underpinnings of emotional processing, scientists can better understand how various conditions impact the brain.
This groundbreaking study, published in Intelligent Computing, demonstrates the potential of merging neuroscience, AI, and affective computing to enhance PD diagnostics.
As researchers continue to innovate, the hope is to bring these advancements from the lab to clinical practice, benefiting millions worldwide.
Note: Materials provided above by The Brighter Side of News. Content may be edited for style and length.
Like these kind of feel good stories? Get The Brighter Side of News' newsletter.