Optical deep neural networks are revolutionizing AI computation
Discover how photonic deep neural networks achieve light-speed AI processing with ultra-efficiency, transforming machine learning hardware.
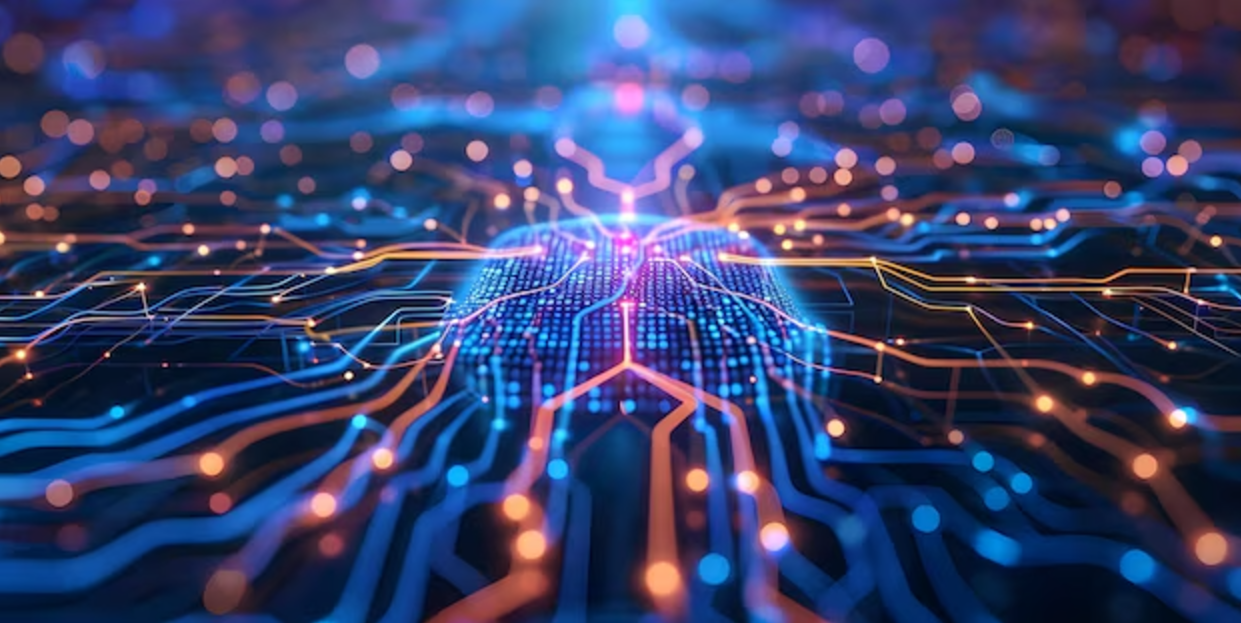
Photonic deep neural networks revolutionize AI hardware with light-speed computations and unmatched energy efficiency. (CREDIT: Freepik)
Modern artificial intelligence systems rely on deep neural networks (DNNs) that demand immense computational resources.
Traditional electronic processors often struggle to meet the growing needs of machine learning tasks, especially in terms of energy efficiency and processing speed.
Photonic hardware, which uses light for computation, offers a transformative solution, and recent advancements in this technology are setting new benchmarks for AI hardware.
Linear algebra underpins most computations in DNNs. These calculations are pivotal for applications ranging from self-driving cars to scientific research. However, the computational and thermal limitations of traditional electronic systems necessitate innovative hardware solutions.
Photonic systems can process information by manipulating light, inherently offering lower latency and higher energy efficiency. Unlike their electronic counterparts, they avoid the need for repeated optical-to-electrical conversions, preserving the integrity of data and reducing power consumption.
Such capabilities are critical in applications where speed and precision are paramount, such as lidar systems for autonomous vehicles, particle physics experiments, and high-speed optical telecommunications.
A Breakthrough in Photonic Hardware
Scientists have now demonstrated a fully integrated photonic processor capable of performing all computations required by a deep neural network.
Related Stories
This device achieves remarkable accuracy and speed, completing machine-learning classification tasks with over 92% accuracy in less than half a nanosecond. These results are comparable to those of traditional hardware but with significantly enhanced efficiency.
This breakthrough leverages these key innovations:
Coherent Programmable Optical Nonlinearities
Nonlinear operations enable DNNs to recognize intricate patterns in data. Historically, integrating such operations into photonic systems has been challenging due to the high energy demands of optical nonlinearities.
The research team resolved this by designing nonlinear optical function units (NOFUs) that combine electronics and optics, enabling efficient and reconfigurable nonlinear computations directly on the chip.
Coherent Matrix Multiplication Units
Matrix multiplication is central to DNNs. Photonic systems traditionally faced bottlenecks due to the need for optical-to-electronic conversions.
By integrating coherent matrix multiplication units (CMXUs) that utilize light's amplitude and phase, the researchers eliminated these bottlenecks, achieving faster and more energy-efficient computations.
In Situ Training
Training DNNs requires evaluating large datasets to optimize model parameters, a process that consumes substantial computational resources.
This photonic processor enables in situ training by performing rapid, low-energy inference directly on optical signals. Such capabilities are especially beneficial for real-time applications, including edge devices and optical communication systems.
Realizing a Fully Integrated Photonic System
The new system encodes neural network parameters into light and performs computations using programmable beam splitters and NOFUs. This design maintains data in the optical domain throughout the process, significantly reducing latency and energy consumption.
During tests, the system achieved 96% accuracy during training and over 92% during inference. These results match the performance of traditional hardware, but the photonic approach completed computations in a fraction of the time.
The chip integrates 132 tunable parameters on a compact 6 × 5.7 mm² silicon photonic platform. Fabricated using commercial foundry processes, the device is scalable and compatible with existing CMOS manufacturing infrastructure, making large-scale production feasible.
Applications and Future Directions
This technology has broad implications for fields requiring rapid and energy-efficient computations. Autonomous systems, scientific instrumentation, and telecommunications stand to benefit immensely. The chip’s ability to perform real-time training further expands its potential, particularly in adaptive systems that require continuous learning.
Saumil Bandyopadhyay, a key researcher on the project, highlights the potential for practical applications: "Now that we have an end-to-end system that can run a neural network in optics, at a nanosecond time scale, we can start thinking at a higher level about applications and algorithms."
The team plans to scale the device and integrate it with real-world systems like cameras and telecommunications networks. They are also exploring new algorithms to leverage optical advantages for faster and more energy-efficient training.
Dirk Englund, a senior researcher, emphasizes the significance of this work: "This demonstrates that computing can be compiled onto new architectures of linear and nonlinear physics, enabling fundamentally different scaling laws of computation."
This breakthrough represents a critical step toward realizing the full potential of photonic deep neural networks. By addressing longstanding challenges in photonic integration and energy efficiency, the researchers have paved the way for a new era of AI hardware.
Note: Materials provided above by The Brighter Side of News. Content may be edited for style and length.
Like these kind of feel good stories? Get The Brighter Side of News' newsletter.
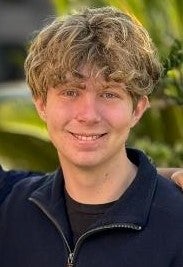