New AI system detects common sleep disorder with 92% accuracy – helping millions
AI analyzes sleep videos to diagnose REM sleep behavior disorder with 92% accuracy, offering an early warning sign for Parkinson’s.
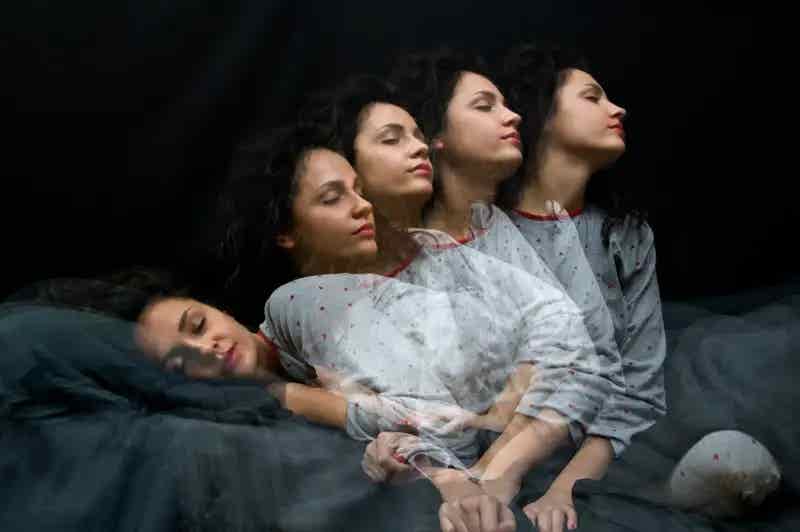
Scientists have trained AI to spot REM sleep behavior disorder using sleep lab videos. (CREDIT: CC BY-SA 4.0)
In the stillness of the night, when the body should lie calm in sleep, something else may be happening beneath the surface. For some people, the dreaming mind turns the body into a stage for sudden kicks, jerks, or even violent thrashes. These are not restless movements from bad dreams, but signs of a complex and often misunderstood sleep disorder—REM sleep behavior disorder, or RBD.
The Early Sign of Something More
RBD is a condition where people physically act out their dreams during REM (rapid eye movement) sleep. That’s the part of the sleep cycle when the brain is most active and dreaming is at its peak. Normally, the body becomes temporarily paralyzed during REM sleep to keep dreams from being physically acted out. But in those with RBD, this natural muscle “off switch” fails.
The disorder most often shows up in otherwise healthy people over age 40. At that stage, it’s called isolated RBD (iRBD). Though the word “isolated” might suggest the issue is minor or temporary, iRBD is usually the first visible clue of a deeper condition. In almost all cases, it becomes an early marker for brain diseases like Parkinson’s or certain types of dementia.
Up to 1.5% of middle-aged and older adults are believed to have iRBD, yet many don’t know they have it. The condition can be easy to miss, especially when the symptoms are mild. Sometimes, a partner might notice odd movements during the night. Other times, people only learn of the disorder after an injury from a more extreme episode.
Why Diagnosis Is So Difficult
Getting an accurate diagnosis of iRBD has never been easy. That’s part of why many cases slip through the cracks. While dream-enacting behaviors (DEBs) like punching, yelling, or kicking can strongly suggest the disorder, these behaviors don’t happen every night. Sometimes, only brief movements of the head, limbs, or torso occur. And not all of these are tied to dreams.
Screening surveys exist, but they often lack precision. They may misidentify other sleep disorders like sleep apnea or restless leg syndrome as iRBD. The only way to truly confirm the diagnosis is through an overnight test called video-polysomnography (vPSG). This involves sleeping in a lab while a team collects data on muscle activity, eye movements, and brain waves. But even this “gold standard” test has its flaws.
Related Stories
Reviewing the data from a vPSG takes time. Experts must go through every 30-second clip of REM sleep, filtering out false signals like twitches caused by arousals or noise. This process isn’t just slow—it’s complex and prone to human error. And even with a perfect setup, many patients don’t show dramatic dream enactments during a single night. That makes catching the disorder in action unlikely.
The video footage recorded during the test, while rich in information, is often ignored or thrown out after the exam is scored. That’s a missed opportunity, according to researchers.
Technology That Sees What Others Miss
In a study led by Mount Sinai’s Icahn School of Medicine, a team of researchers found a better way to make use of that video data. They developed an artificial intelligence (AI) system to scan video recordings from standard 2D cameras used in most sleep labs. The study was published in Annals of Neurology.
“This automated approach could be integrated into clinical workflow during the interpretation of sleep tests to enhance and facilitate diagnosis, and avoid missed diagnoses,” said Dr. Emmanuel During, who led the research. He’s a professor of neurology and medicine at Mount Sinai.
The researchers weren’t the first to try this. Scientists at the Medical University of Innsbruck in Austria had already built an AI model using 3D cameras to spot movement patterns linked to RBD. Those cameras were considered necessary because they could capture depth and detect motion even under blankets. But they are expensive and rare in real-world clinics.
The Mount Sinai team, along with computer vision experts from Switzerland’s École Polytechnique Fédérale de Lausanne, wanted to show that regular 2D cameras could do the job just as well. These are the same kinds of cameras already installed in most sleep labs. Using data collected from 172 people tested at the Stanford Sleep Center between 2016 and 2022, the team trained their AI to tell the difference between RBD and other sleep conditions.
The dataset included 81 people with confirmed iRBD and 91 others with different sleep problems. All patients were over age 40 and had spent at least four hours asleep with five minutes or more of REM sleep recorded.
To measure movement, the AI looked at how pixels in each video frame changed from one second to the next. It then pulled out five features from those motion patterns: how often movements occurred, how long they lasted, how strong they were, how fast they were, and how still the body remained overall.
The results were striking. With only short movements—lasting 0.1 to 2 seconds—the algorithm reached an accuracy of nearly 92% in identifying iRBD. That’s the best result reported for this method so far. The model even outperformed previous systems that relied on expensive 3D cameras.
Better Tools Could Mean Safer Nights
This research has major implications. Not only does it lower the cost of reliable diagnosis, but it also improves patient safety. Knowing whether a person has iRBD early on allows for preventive steps. These might include locking away sharp objects or moving furniture to avoid injuries during sleep. In more serious cases, doctors might begin close monitoring for signs of Parkinson’s or dementia.
Even more importantly, a tool like this could fit right into the existing systems at sleep labs. That means clinicians could start using the technology without having to buy new equipment or retrain staff.
Dr. During believes the system could also help tailor treatment. “This method could also be used to inform treatment decisions based on the severity of movements displayed during the sleep tests and, ultimately, help doctors personalize care plans for individual patients,” he explained.
Looking Toward the Future
The study also opens the door for further research. Could home video systems detect iRBD without the need for a lab? Could movement data help predict who will go on to develop Parkinson’s disease? The answers remain to be seen, but this work marks a big leap forward.
By using smart tools to unlock hidden insights from old data, scientists have found a better way to understand a silent and early warning sign of neurodegeneration. For patients and doctors alike, that could mean better care, faster answers, and safer sleep.
Note: The article above provided above by The Brighter Side of News.
Like these kind of feel good stories? Get The Brighter Side of News' newsletter.
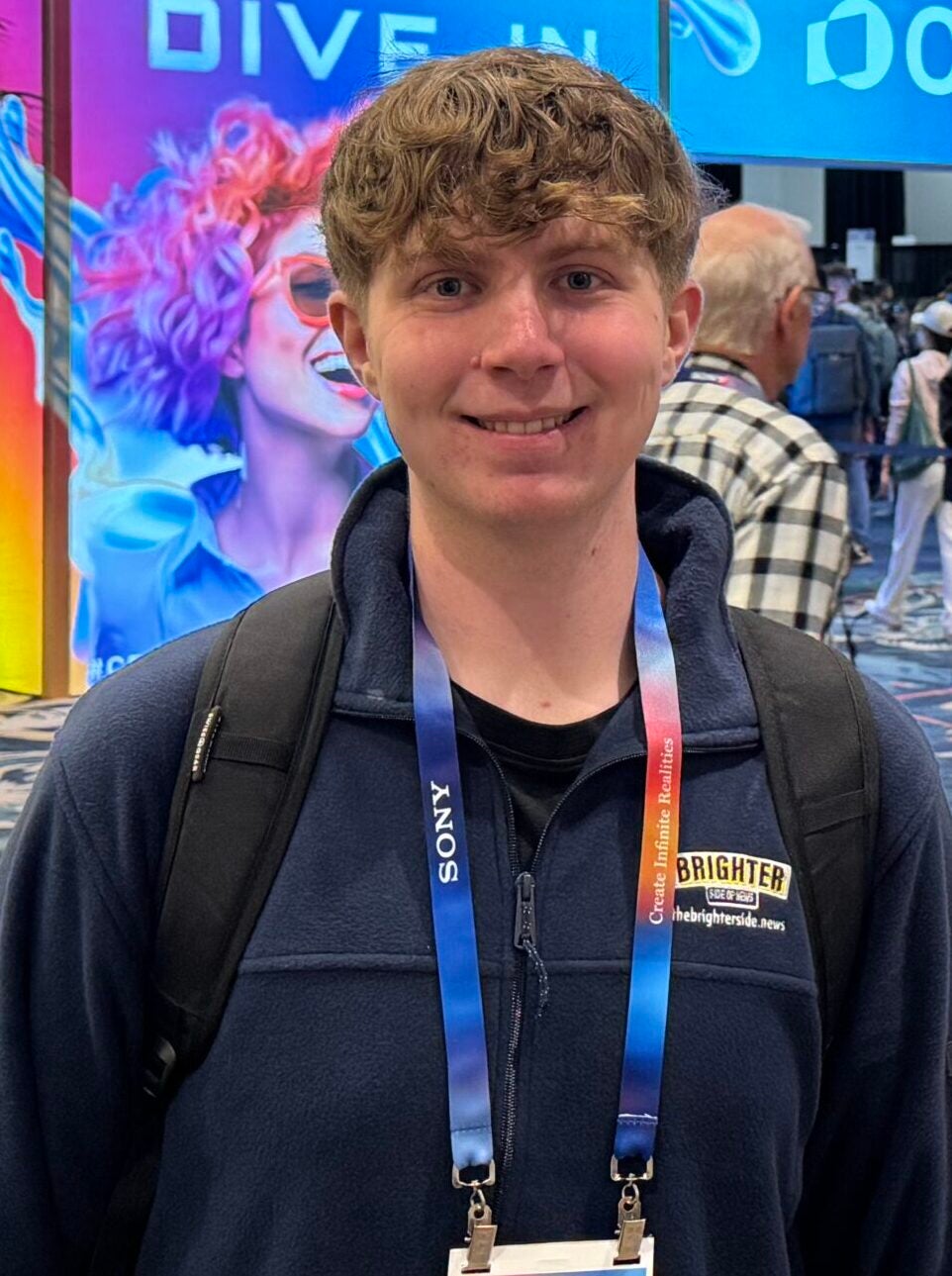
Joshua Shavit
Science & Technology Writer | AI and Robotics Reporter
Joshua Shavit is a Los Angeles-based science and technology writer with a passion for exploring the breakthroughs shaping the future. As a contributor to The Brighter Side of News, he focuses on positive and transformative advancements in AI, technology, physics, engineering, robotics and space science. Joshua is currently working towards a Bachelor of Science in Business Administration at the University of California, Berkeley. He combines his academic background with a talent for storytelling, making complex scientific discoveries engaging and accessible. His work highlights the innovators behind the ideas, bringing readers closer to the people driving progress.