Lifesaving AI technology tracks how cells respond to drug treatments
A new AI tool called scNET uses gene and cell networks to reveal hidden patterns in noisy single-cell RNA sequencing data.
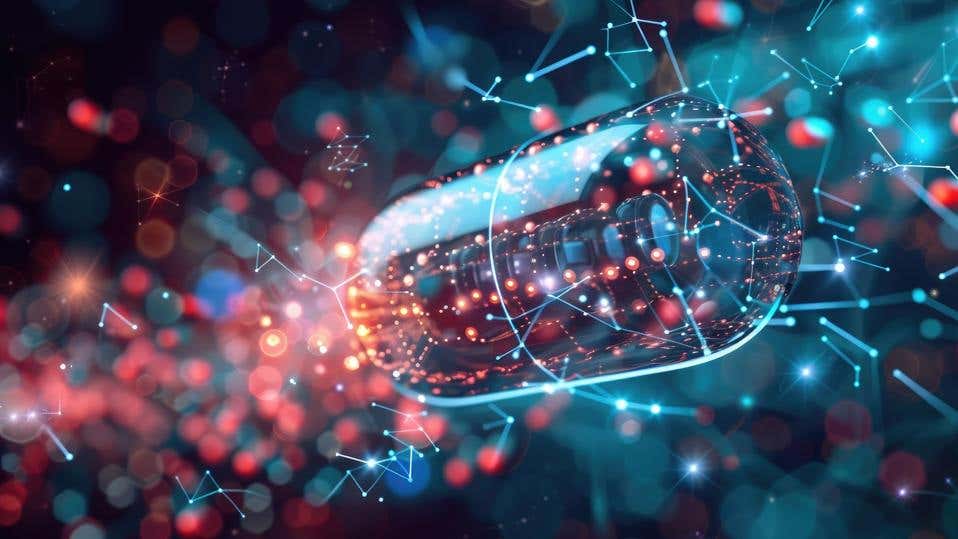
AI-powered tool scNET links gene and cell networks to reveal hidden patterns in single-cell data. (CREDIT: Adobe Stock)
Single-cell gene sequencing has changed the way scientists understand life at the smallest level. It lets researchers study how each cell behaves, especially when cells face challenges like disease or drug treatments. But while the technology provides great detail, it also creates a huge amount of noise in the data. This noise often hides important biological signals, making it hard to draw accurate conclusions.
One of the most promising solutions to this problem, published in the scientific journal Nature Methods, comes from a new method called scNET. Developed by researchers at a university in Israel, this system blends two powerful tools: single-cell RNA sequencing and protein–protein interaction networks. By combining these, scNET can give you a much clearer picture of how genes and cells interact across different conditions.
The noise problem in single-cell data
Single-cell RNA sequencing, or scRNA-seq, lets you examine gene activity inside individual cells. Unlike older methods that average gene activity across millions of cells, this newer approach allows scientists to spot unique behaviors of different cells—even when they belong to the same tissue or organ.
However, there’s a catch. A large number of the recorded gene counts are zeros. Sometimes, this is because the gene truly isn’t active in a cell. But often, it’s just a technical problem—a gene was active, but the sequencing method didn’t detect it. This issue is called dropout, and it creates a lot of false zero values in the data.
When so many gene activities go missing or unrecorded, it becomes tough to study how genes work together or how pathways get activated in response to changes. Researchers have tried to fix this using many statistical and computational tricks.
Some use complex models to estimate the missing values, while others try to group similar cells and guess what genes they should express. These approaches have helped, but they usually focus on either fixing the numbers or grouping the cells—not both at once.
A smarter approach: connecting the dots with networks
To go beyond patching up the numbers, scientists started to use protein–protein interaction (PPI) networks. These maps show how proteins—products of genes—interact inside the cell. PPIs capture more than just numbers. They provide real context: how genes might function together as part of larger processes like cell division or immune responses.
Related Stories
But PPIs come with their own limits. Most are built using large datasets that combine information from many different tissues and conditions. So while they’re useful, they don’t reflect what’s going on in a specific sample—like a tumor or a group of immune cells in a sick patient.
That’s where combining PPIs with scRNA-seq comes in. When you bring the dynamic data of gene activity from single cells into these static PPI maps, you get something much more useful: a way to see how gene relationships shift in real time across different biological states.
Previous research had already shown that mixing these two sources of information can boost tasks like finding key genes, predicting survival rates, and improving cell clustering. But until recently, there wasn’t a method that could combine all this power in one place—especially for small datasets where labeled cell types might not even be known.
scNET: Seeing cells and genes in a new way
scNET changes the game by doing something simple yet powerful. It doesn’t just look at genes. It also looks at cells. This dual view allows it to learn how cells are similar to one another and how genes work together—all at the same time.
At the core of scNET is a graph neural network, or GNN. This type of artificial intelligence model is great at working with networks—just like a social network can show how people are connected, GNNs can reveal how genes and cells connect. In this model, one graph represents gene interactions (based on PPIs), and another represents cell-to-cell similarities (based on how similar their gene activity profiles are).
Rather than fixing the number of neighbors each cell has—something most models do—scNET introduces a flexible edge-attention mechanism. This lets it learn which cells are truly related, instead of forcing each cell to be linked to a set number of others. This better reflects how biology actually works, where some cells may be deeply connected and others might stand apart.
By learning from both the gene and cell networks together, scNET smooths out the noise and learns accurate gene and cell embeddings—compact representations that hold key information. These embeddings can then be used to improve downstream analysis tasks, like clustering cells, spotting gene relationships, and identifying important biological pathways.
Breakthroughs in cancer and immune cell research
To test scNET’s abilities, the research team focused on immune cells called T cells. These cells play a key role in fighting tumors. But understanding how they change in response to treatment is tough, especially when the data is messy.
Using scNET, the researchers could finally see which T cells became more active after treatment. They noticed an increase in cytotoxic behavior—where T cells attack and kill tumor cells. This insight was buried in the original noisy data but became clear with scNET’s improved analysis.
Ron Sheinin, the lead PhD student on the project at Tel Aviv University, explained: “scNET integrates single-cell sequencing data with networks that describe possible gene interactions, much like a social network, providing a map of how different genes might influence and interact with each other.”
With this better view, it became possible to detect how different treatments affected not just the tumor but also the immune cells around it—something that’s key for improving cancer therapies.
Prof. Asaf Madi, one of the supervising scientists, added: “We focused on a population of T cells, immune cells known for their power to fight cancerous tumors. scNET revealed the effects of treatments on these T cells and how they became more active in their cytotoxic activity against the tumor—something that was not possible to discover before due to the high level of noise in the original data.”
A wider impact in biology and medicine
The promise of scNET goes far beyond cancer. By making it easier to understand how genes behave under different conditions, it could help with a wide range of diseases—from autoimmune conditions to neurological disorders. It also opens the door to more targeted drug development.
Professor Roded Sharan, who co-led the research, emphasized the bigger picture: “This is an excellent example of how artificial intelligence tools can help decipher biological and medical data, allowing us to gain new and significant insights. The idea is to provide biomedical researchers with computational tools that will aid in understanding how the body’s cells function, thereby identifying new ways to improve our health.”
In tests, scNET outperformed older methods in identifying gene–gene interactions and clustering similar cells. It also uncovered more biologically meaningful pathways and gave better insight into how different treatments influence cell behavior.
What sets it apart is its ability to work without needing labeled data—a major advantage for new experiments where the researchers don’t yet know what cell types are present. It’s this flexibility that makes scNET a strong tool for scientists studying complex biological systems.
As researchers continue to expand their use of AI in biology, tools like scNET will become central to making sense of the flood of new data. They offer a way to cut through the noise, see patterns more clearly, and bring us closer to more effective and personalized medicine.
Note: The article above provided above by The Brighter Side of News.
Like these kind of feel good stories? Get The Brighter Side of News' newsletter.
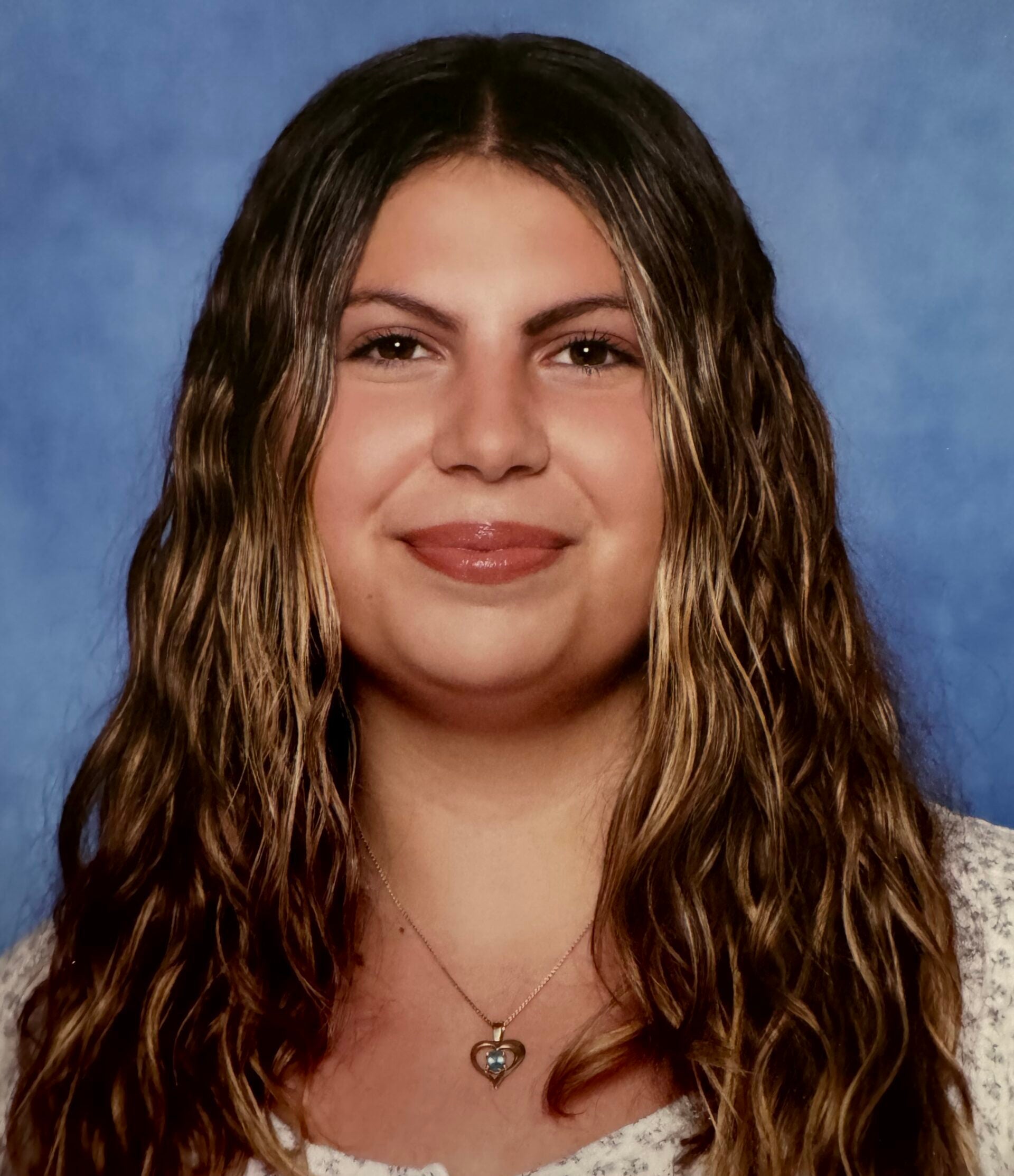
Rebecca Shavit
Science & Technology Journalist | Innovation Storyteller
Based in Los Angeles, Rebecca Shavit is a dedicated science and technology journalist who writes for The Brighter Side of News, an online publication committed to highlighting positive and transformative stories from around the world. With a passion for uncovering groundbreaking discoveries and innovations, she brings to light the scientific advancements shaping a better future. Her reporting spans a wide range of topics, from cutting-edge medical breakthroughs and artificial intelligence to green technology and space exploration. With a keen ability to translate complex concepts into engaging and accessible stories, she makes science and innovation relatable to a broad audience.