Lifechanging brain research reveals four different types of Autism
Groundbreaking research identifies four autism subtypes through brain activity and genetics, paving the way for personalized treatments.
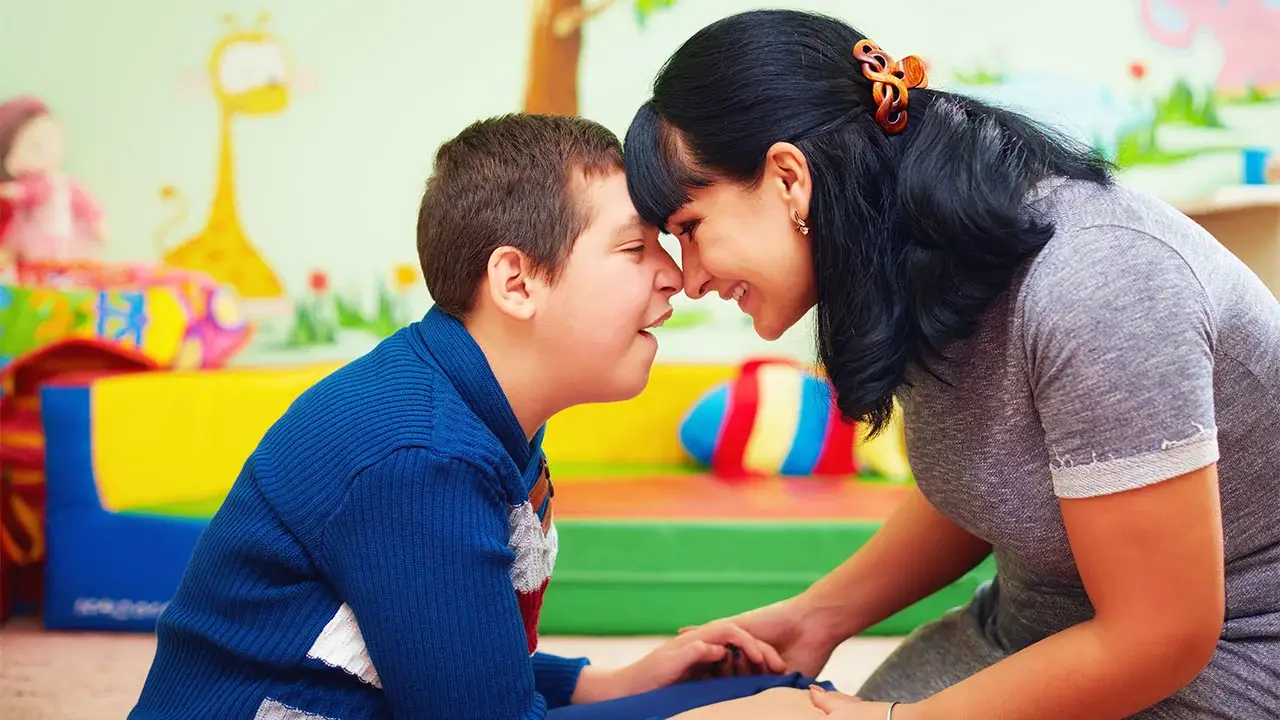
Groundbreaking research identifies four autism subtypes using brain activity and genetics. (CREDIT: CC BY-SA 4.0)
Autism spectrum disorder (ASD) encompasses a wide array of challenges in social interaction, communication, and repetitive behaviors. It’s not a single entity but a diverse condition with varying intellectual abilities and medical complications. This heterogeneity complicates identifying the root causes and crafting effective treatments.
Neuroimaging has shed light on ASD’s complexity. Functional magnetic resonance imaging (fMRI) studies have revealed atypical activity in key brain areas tied to impaired social cognition and language processing. The thalamus, visual regions, and salience network often exhibit unusual connectivity.
Moreover, repetitive behaviors correlate with abnormalities in the frontostriatal circuits that regulate inhibitory control. By analyzing large-scale resting-state fMRI (rsfMRI) datasets, researchers have found significant differences in functional connectivity across brain networks in individuals with ASD compared to neurotypical controls. These variations highlight the complex interplay between brain activity and the diverse symptoms seen in ASD.
A groundbreaking study from Weill Cornell Medicine further advanced understanding by identifying four distinct ASD subtypes based on brain activity and behavior. Using machine learning, the team analyzed neuroimaging data from nearly 300 individuals with autism and over 900 neurotypical participants.
Their findings were published in Nature Neuroscience. Dr. Amanda Buch, the study’s lead author, developed novel methods for integrating neuroimaging with gene expression data, uncovering new insights into how genetic risk factors manifest in different autism subgroups.
Dr. Conor Liston, co-senior author and a professor at Weill Cornell Medicine, emphasized the study’s significance. “Autism is associated with diverse presentations and limited therapeutic options. Our work provides a framework to better understand this variability,” he said. The team’s research not only highlights the biological diversity within ASD but also offers a potential path toward more tailored diagnostic and therapeutic strategies.
Machine learning enabled the researchers to identify patterns of brain connectivity linked to distinct behavioral traits. Two subgroups exhibited above-average verbal intelligence, but their challenges differed. One had severe social communication deficits with limited repetitive behaviors, while the other showed the opposite pattern.
The remaining two groups shared significant impairments in social interactions and repetitive behaviors but had opposite verbal abilities. Despite these behavioral overlaps, the neuroimaging data revealed entirely distinct patterns of brain connectivity.
Related Stories
The team’s findings also included a detailed analysis of the underlying genetics. They integrated rsfMRI data with normative gene expression information, identifying regional differences in gene activity linked to atypical brain connectivity in each subgroup. Many implicated genes had been previously associated with autism, providing further validation of the results.
For instance, the study highlighted oxytocin’s role—a protein linked to social interactions—as a hub protein in one subgroup characterized by social impairments but relatively limited repetitive behaviors. These findings could inform future therapeutic approaches, such as testing whether oxytocin therapy is more effective for specific subgroups.
This research builds on earlier studies, including a 2017 investigation that identified biologically distinct subtypes of depression using similar machine-learning methods. Those findings have since guided the development of targeted therapies for depression, underscoring the potential of this approach for autism as well.
Dr. Logan Grosenick, another co-lead author, noted the transformative potential of these discoveries. “One of the reasons it’s so difficult to develop therapies for autism is the variability within the population. By identifying subgroups, we might create targeted treatments rather than relying on a one-size-fits-all approach,” he said.
This perspective resonates with the broader scientific community. Dr. Kevin Pelphrey of George Washington University called the study “groundbreaking,” emphasizing its potential to refine diagnostic criteria and treatment strategies.
The team’s methodology relied on advanced statistical tools like regularized canonical correlation analysis (RCCA) to reduce overfitting and improve generalizability. By analyzing two large rsfMRI datasets from the Autism Brain Imaging Data Exchange (ABIDE I and II), they identified three dimensions of brain-behavior relationships.
These dimensions corresponded to verbal ability, social affect, and repetitive behaviors. Hierarchical clustering along these axes revealed four distinct ASD subgroups, validated using independent data.
The study also benefited from a vast collaborative effort. Data from 17 institutions across the United States, Europe, and Australia were crucial in creating the ABIDE dataset. Dr. Adriana Di Martino, research director at the Autism Center at the Child Mind Institute, played a pivotal role in assembling this resource.
Dr. Buch highlighted the importance of these contributions, stating that such large datasets enable robust machine-learning studies that were previously impossible.
While the study offers valuable insights, the authors caution that more research is needed. Dr. Buch and her team plan to explore the underlying biology of the identified subgroups further, including studies in animal models to test potential therapies. Their goal is to translate these findings into personalized medical approaches that improve outcomes for individuals with autism.
The implications extend beyond treatment. Refined diagnostic criteria based on biological subtypes could enhance the accuracy of ASD diagnoses. Dr. Liston noted, “Current diagnostic criteria are broad and apply to a diverse group of people. Identifying subtypes could help refine these criteria, improving diagnosis and treatment.”
The study has garnered widespread attention among researchers and autism advocates. Ari Ne’eman, co-founder of the Autistic Self Advocacy Network, praised the work for advancing understanding and shifting the focus away from a “cure” toward a nuanced view of autism. He remarked, “This research underscores autism’s diversity. Understanding subtypes can lead to better support and therapies tailored to individual needs.”
Funding from the National Institutes of Health and the Simons Foundation Autism Research Initiative supported the study. The researchers expressed deep gratitude to the families who participated, acknowledging their vital role in advancing scientific knowledge. Dr. Liston emphasized, “Without the generosity of these families, this research wouldn’t have been possible.”
This pioneering study marks a significant step forward in unraveling the complexities of autism. By identifying distinct subtypes and their underlying biology, it lays the groundwork for a future where personalized medicine transforms how autism is understood, diagnosed, and treated.
Note: Materials provided above by The Brighter Side of News. Content may be edited for style and length.
Like these kind of feel good stories? Get The Brighter Side of News' newsletter.
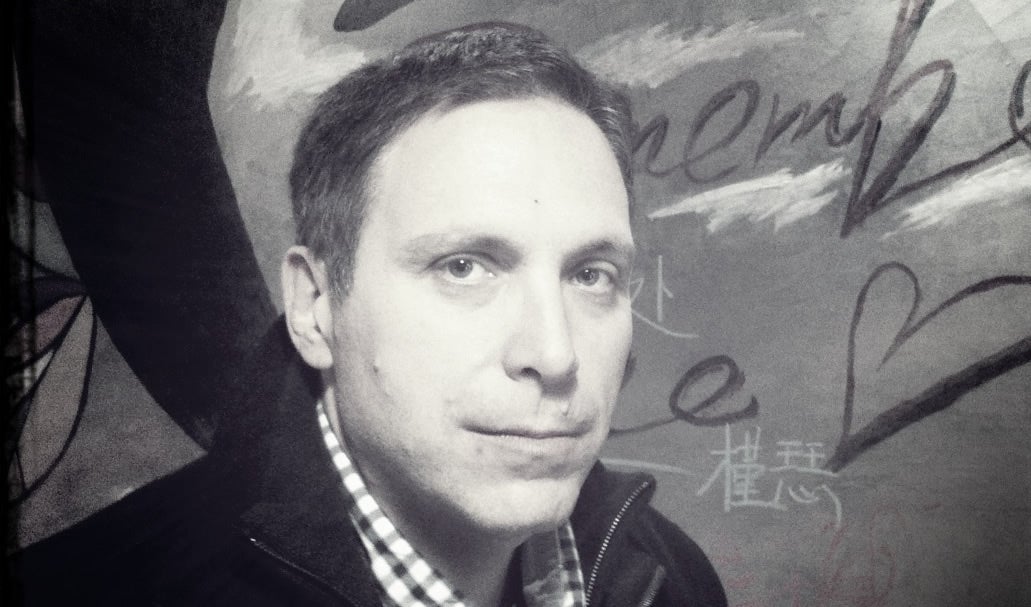