Groundbreaking new AI precisely calculates the universe’s ‘initial settings’
Researchers harness AI to dramatically improve cosmological parameter estimates. This breakthrough could resolve the Hubble tension and deepen our understanding of the universe’s dark energy and matter.
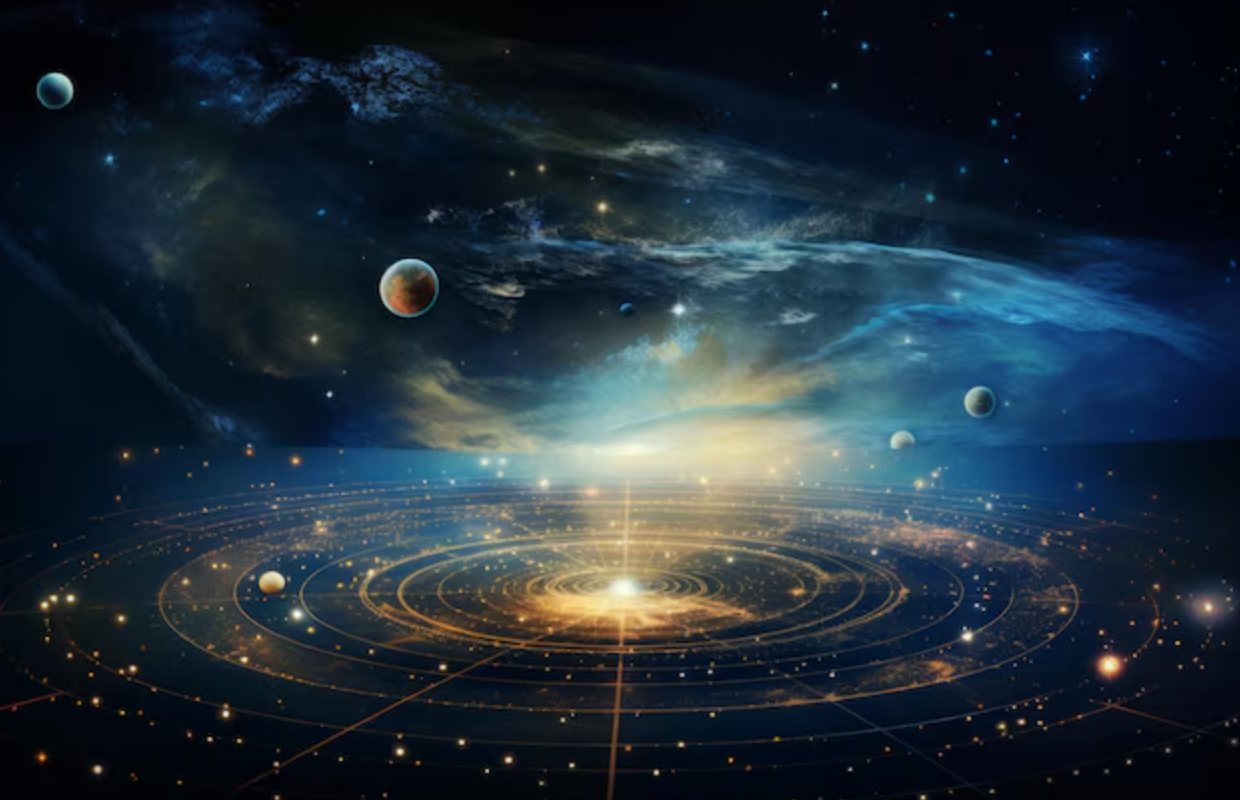
Researchers from the Flatiron Institute and their colleagues have developed a groundbreaking method to estimate the universe's cosmological parameters with remarkable precision using artificial intelligence (AI).
Their new approach, called the Simulation-Based Inference of Galaxies (SimBIG), uncovers information hidden in the distribution of galaxies, significantly improving previous estimation techniques.
This AI-driven method led to a substantial reduction in the uncertainty of parameters, particularly in describing the universe’s matter clumpiness, achieving less than half the uncertainty compared to conventional approaches. It also aligns closely with estimates from other observations, such as the universe’s oldest light, providing confidence in its accuracy.
The findings, published in Nature Astronomy, promise to enhance our understanding of the universe’s fundamental makeup.
The cosmological parameters are vital in describing the universe's composition and evolution, including the amounts of ordinary matter, dark matter, and dark energy. They also help explain the conditions shortly after the Big Bang, like the opacity of the early universe and the distribution of mass.
Related Stories
These parameters "are essentially the ‘settings’ of the universe that determine how it operates on the largest scales," explains Liam Parker, a research analyst at the Flatiron Institute’s Center for Computational Astrophysics (CCA) and a co-author of the study.
To derive these parameters, scientists often study the clustering of galaxies, but previous methods were limited to analyzing only large-scale galaxy distributions. ChangHoon Hahn, an associate research scholar at Princeton University and lead author of the study, explains, “We haven’t been able to go down to small scales... there’s additional information there; we just didn’t have a good way of extracting it.”
Hahn’s solution was to harness AI to tap into this untapped small-scale data. His two-phase plan first involved training an AI model to learn the values of cosmological parameters from simulated universes. The next step was applying this trained model to actual galaxy distribution data.
For training, the researchers fed the model 2,000 simulations from the CCA’s Quijote suite, each created with different cosmological parameter values. These simulated universes mimicked real-world data from galaxy surveys, including imperfections caused by the atmosphere and telescopes. “That’s a large number of simulations, but it’s a manageable amount,” says Hahn. Without AI, he adds, they would have needed hundreds of thousands of simulations to achieve the same results.
Through these simulations, the AI learned to correlate the values of the cosmological parameters with small-scale differences in galaxy clustering. For example, it learned to analyze the distance between individual galaxies and extract additional information by looking at groups of three or more galaxies and the geometric shapes they form.
Once trained, the AI model was tested using actual data from 109,636 galaxies measured by the Baryon Oscillation Spectroscopic Survey. As hoped, the AI successfully used both small-scale and large-scale galaxy details to significantly improve the precision of cosmological parameter estimates. Remarkably, this precision was equivalent to that of a traditional analysis using roughly four times as many galaxies.
This is particularly important because the universe contains a limited number of galaxies. According to Shirley Ho, a group leader at CCA and a co-author of the study, “By getting higher precision with less data, SimBIG can push the limits of what’s possible.”
Ho also emphasized the practical significance of these cosmological parameters, given the enormous cost of the galaxy surveys. “Each of these surveys costs hundreds of millions to billions of dollars,” she says. “You want the best analysis you can to extract as much knowledge out of these surveys as possible and push the boundaries of our understanding of the universe.”
One of the key future applications of SimBIG’s precision is addressing the Hubble tension. This cosmological issue refers to the discrepancy in estimates of the Hubble constant, which describes the rate at which the universe is expanding.
Estimates based on the spacing of fluctuations in the universe’s oldest light differ by about 10% from those based on the distance to supernovae in distant galaxies. This mismatch has sparked debate and suggests that a deeper understanding or even new physics might be needed.
As new surveys of the cosmos come online over the next few years, SimBIG’s AI-powered approach will allow researchers to better explore the Hubble tension and determine whether the mismatch in estimates can be resolved. If not, it could indicate that the universe operates under physical laws we don’t yet fully understand.
“If we measure the quantities very precisely and can firmly say that there is a tension, that could reveal new physics about dark energy and the expansion of the universe,” Hahn says.
The Nature Astronomy study represents a major step forward in cosmology. Their innovative use of AI to extract hidden data and improve precision offers new opportunities to unravel the mysteries of the universe and advance our knowledge of dark matter, dark energy, and the forces shaping the cosmos.
Note: Materials provided above by The Brighter Side of News. Content may be edited for style and length.
Like these kind of feel good stories? Get The Brighter Side of News' newsletter.
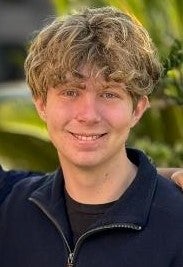