Groundbreaking AI technology revolutionizes clean water production
Researchers developed an AI-driven random forest model that accurately predicts ion concentrations in desalination processes, revolutionizing water quality monitoring.
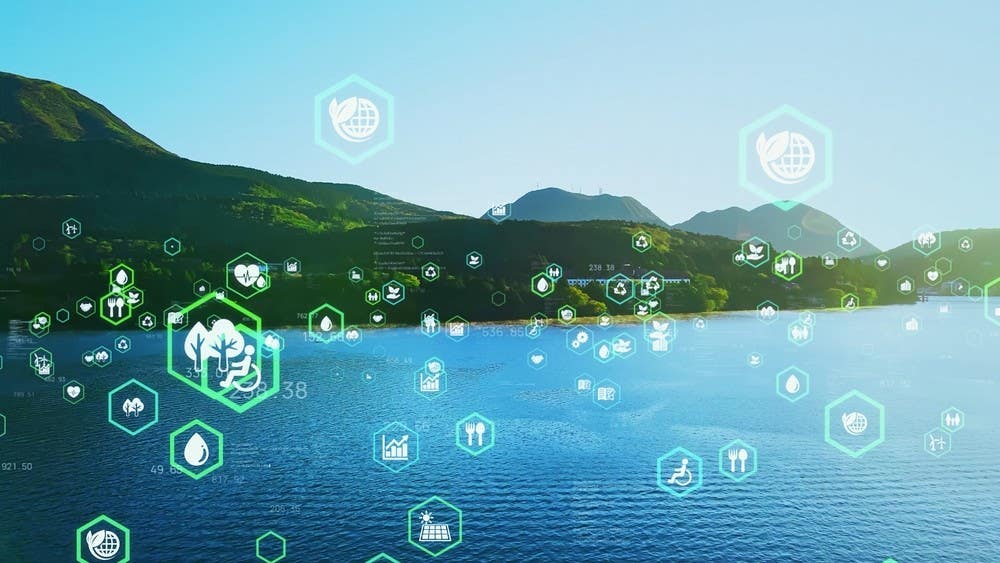
These findings represent a leap forward in the ability to monitor water quality during desalination processes. (CREDIT: CC BY-SA 3.0)
Effluent conductivity is commonly used to evaluate desalination performance because it’s a simple way to assess how well processes like capacitive deionization (CDI) and battery electrode deionization (BDI) remove ions. Typically, conductivity is converted into ion concentration, but this method is often inaccurate.
To improve precision, researchers have been exploring more reliable techniques for measuring ion concentrations in real time. In a recent study, a team developed a random forest (RF)-based artificial intelligence (AI) model that significantly enhances the accuracy of these measurements.
The RF model was validated to predict effluent conductivity for both CDI and BDI processes, achieving an impressive R² of 0.86 for CDI and 0.95 for BDI. The AI model didn’t just stop at conductivity predictions; it also accurately estimated concentrations of specific ions like sodium (Na⁺), potassium (K⁺), calcium (Ca²⁺), and chloride (Cl⁻). In fact, its predictions for ion concentrations were even more accurate than those for conductivity, due to a strong linear correlation in the dataset.
This suggests that the AI model captures complex interactions that traditional methods often overlook. Additionally, the study examined how different sampling intervals impacted prediction accuracy. The results showed that intervals under 80 seconds did not significantly affect the model’s accuracy, meaning the AI can reliably predict ion concentrations even with less frequent data collection.
These findings represent a leap forward in the ability to monitor water quality during desalination processes. Random forest models like the one developed in this study have the potential to become crucial tools in decentralized water production systems, which are increasingly in demand.
Related Stories
Globally, more than 2.2 billion people—over a quarter of the population—lack access to safe drinking water. Additionally, half the world’s population faces severe water shortages at least once a year. To address these challenges, governments are investing in costly water management methods, such as sewer irrigation, rainwater reuse, and seawater desalination.
Centralized water distribution systems, while effective, have their limitations. They struggle to quickly adapt to changing water demands, which can exacerbate water scarcity. This has fueled interest in decentralized water production technologies that offer greater flexibility. CDI and BDI are two promising electrochemical-based methods in this field. They are easier to adopt and manage compared to other technologies.
However, one major drawback is the lack of sensors capable of tracking individual ions in the water. Current sensors rely on conductivity to estimate overall water quality, which doesn't provide the granularity needed for precise ion monitoring.
This is where the new AI-driven model becomes vital. Dr. Son Moon’s team from the Korea Institute of Science and Technology (KIST) collaborated with Professor Baek Sang-Soo's team at Yeongnam University to develop a solution that enhances the accuracy of ion monitoring in electrochemical water treatment processes.
The random forest model they created was specifically designed to predict the concentrations of ions in treated water. It excelled in predicting electrical conductivity and the concentrations of ions such as Na⁺, K⁺, Ca²⁺, and Cl⁻, with R² values approaching 0.9 for ion predictions.
The practical benefits of this model extend beyond its accuracy. Unlike deep learning models, which require substantial computational resources, random forest models are more efficient. This efficiency makes them cost-effective for widespread use, especially in national water quality monitoring systems.
In fact, the AI model developed in this study requires 100 times fewer computational resources to train compared to more complex machine learning models. Additionally, the research highlighted the importance of frequent updates—data needs to be updated every 20 to 80 seconds to ensure prediction accuracy. This frequent updating is necessary if the technology is to be integrated into national water quality networks.
Dr. Son Moon emphasized the importance of this research, stating, "The significance of this research is not only in developing a new AI model but also in its application to the national water quality management system. With this technology, the concentration of individual ions can be monitored more precisely, contributing to the improvement of social water welfare."
The ability to monitor ion concentrations in real-time has the potential to revolutionize how we manage water quality, especially in regions facing acute water shortages.
By leveraging AI models like the one developed by the KIST team, it becomes possible to implement smarter, more responsive water management systems that meet the needs of an ever-growing global population.
Note: Materials provided above by The Brighter Side of News. Content may be edited for style and length.
Like these kind of feel good stories? Get The Brighter Side of News' newsletter.
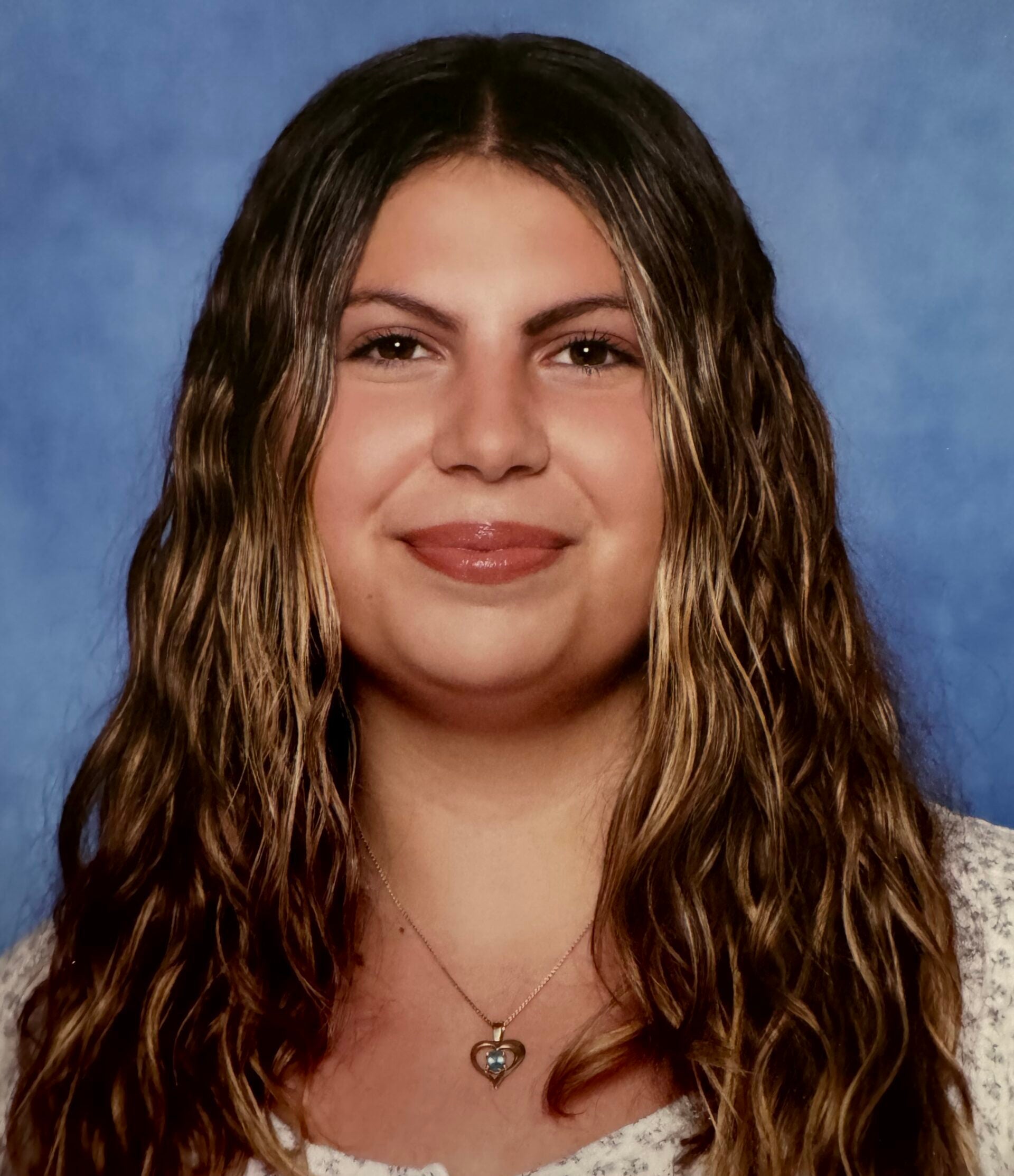
Rebecca Shavit
Science & Technology Journalist | Innovation Storyteller
Based in Los Angeles, Rebecca Shavit is a dedicated science and technology journalist who writes for The Brighter Side of News, an online publication committed to highlighting positive and transformative stories from around the world. With a passion for uncovering groundbreaking discoveries and innovations, she brings to light the scientific advancements shaping a better future. Her reporting spans a wide range of topics, from cutting-edge medical breakthroughs and artificial intelligence to green technology and space exploration. With a keen ability to translate complex concepts into engaging and accessible stories, she makes science and innovation relatable to a broad audience.