Groundbreaking AI can accurately decode emotions from multiple animal species
Scientists used artificial intelligence to analyze animal vocalizations, achieving 89.49% accuracy in detecting emotions across species.
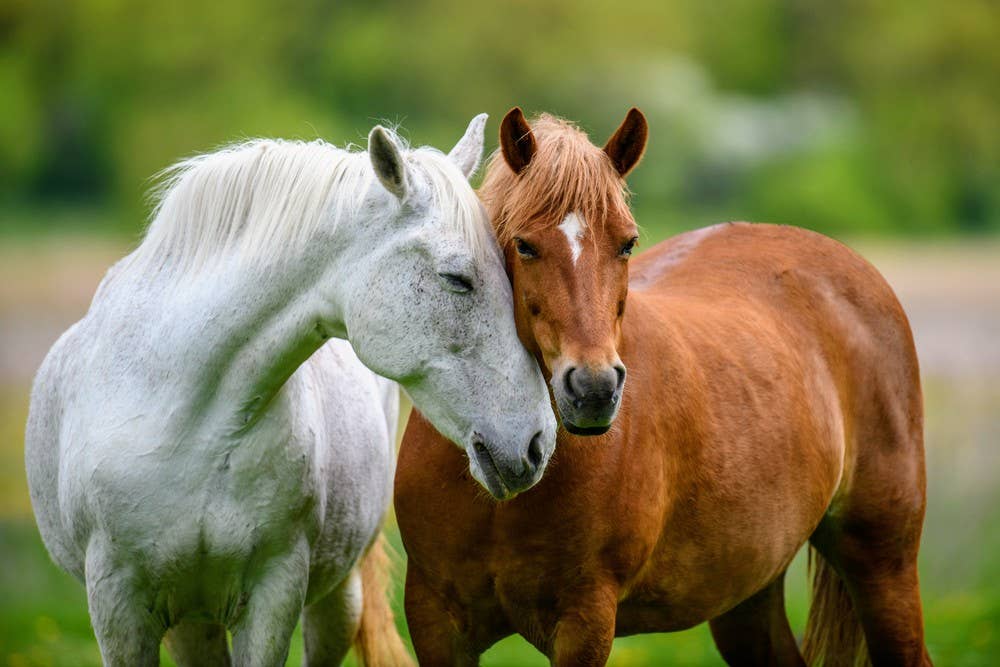
A new study shows AI can decode animal emotions by analyzing vocal patterns with nearly 90% accuracy. (CREDIT: CC BY-SA 4.0)
For years, scientists have tried to understand how animals express emotions. Unlike humans, they cannot describe their feelings, leaving researchers to rely on behavioral and physiological cues. However, recent advances in artificial intelligence (AI) and machine learning offer a new way to decode emotional states—through vocalizations.
A groundbreaking study led by researchers at the University of Copenhagen has taken a major step forward in this field. Using a machine-learning algorithm, scientists analyzed vocalizations from seven different ungulate species, including cows, pigs, and wild boars.
Their findings, published in the journal iScience, reveal that AI can accurately distinguish between positive and negative emotions based on vocal patterns. With an accuracy rate of 89.49%, this marks the first time an AI model has successfully classified emotional valence across multiple species.
The Science Behind Animal Emotions
Emotions trigger changes in both the autonomic and somatic nervous systems. They can be categorized along two key dimensions: arousal (how activated an animal is) and valence (whether an emotion is positive or negative). While arousal is relatively easy to measure using indicators like heart rate or body movement, valence is far more challenging to assess.
Vocalizations, however, provide valuable insight. When an animal produces a sound, its emotional state influences the tension and action of the muscles controlling vocal production. This alters acoustic features such as pitch, duration, and amplitude.
Past studies have shown that vocal indicators of arousal are consistent across species. Even distantly related animals, like crocodiles, can recognize distress in human baby cries. However, evidence for universal vocal markers of valence has been limited.
To address this, the research team applied machine learning to analyze a large dataset of vocal recordings. They used an algorithm called eXtreme Gradient Boosting (XGBoost) to identify patterns that distinguish positive and negative emotional states.
The results suggest that certain vocal characteristics—such as energy distribution, fundamental frequency, and amplitude modulation—are conserved across species and reliably indicate emotional valence.
Related Stories
AI as a Universal Translator of Animal Feelings
The AI model's ability to detect emotional valence across different species represents a major breakthrough. It demonstrates that animals share common vocal markers of emotion, a finding with deep evolutionary significance.
"This breakthrough provides solid evidence that AI can decode emotions across multiple species based on vocal patterns," says Élodie F. Briefer, an associate professor at the University of Copenhagen and the study’s senior author. "It has the potential to revolutionize animal welfare, livestock management, and conservation, allowing us to monitor animals’ emotions in real time."
The study analyzed thousands of vocalizations recorded in different emotional contexts. By identifying key acoustic features, the model determined whether an emotion was positive or negative with remarkable accuracy. This opens the door to developing real-time emotion monitoring tools for use in farming, wildlife conservation, and veterinary care.
A New Era for Animal Welfare and Conservation
These findings could transform how humans care for animals. If AI can accurately detect stress, discomfort, or even happiness, it could lead to major improvements in animal well-being.
"Understanding how animals express emotions can help us improve their well-being," Briefer explains. "If we can detect stress or discomfort early, we can intervene before it escalates. Equally important, we could also promote positive emotions. This would be a game-changer for animal welfare."
In livestock management, such technology could help farmers identify signs of distress, leading to better living conditions and healthier animals. In wildlife conservation, AI-driven monitoring systems could provide insights into how animals respond to environmental changes, improving conservation strategies. The technology may even help scientists study the evolutionary origins of emotional communication, shedding light on the development of human speech.
Key Findings and Future Research
The study's main findings highlight the power of AI in animal emotion research:
- High Accuracy: The AI model classified emotional valence with an overall accuracy of 89.49%.
- Universal Patterns: Key acoustic features predicting emotional valence were consistent across species.
- New Insights Into Evolution: The findings suggest an ancient and shared system of vocal emotional expression among mammals.
To advance research in this field, the team has made their database of labeled animal vocalizations publicly available. By sharing this resource, they hope to accelerate the development of AI tools for monitoring animal emotions.
"We want this to be a resource for other scientists," Briefer says. "By making the data open access, we hope to accelerate research into how AI can help us better understand animals and improve their welfare."
This pioneering study marks a turning point in the field of animal communication. With further research, AI-driven emotion recognition could reshape how humans interact with animals, offering new possibilities for science, conservation, and animal care.
Note: Materials provided above by The Brighter Side of News. Content may be edited for style and length.
Like these kind of feel good stories? Get The Brighter Side of News' newsletter.
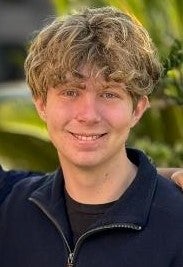