Fast and accurate cancer diagnosis using artificial neurons
AI technology enables learning of the stiffness levels and distributions of the tumor, suggesting the possibility of cancer diagnosis.
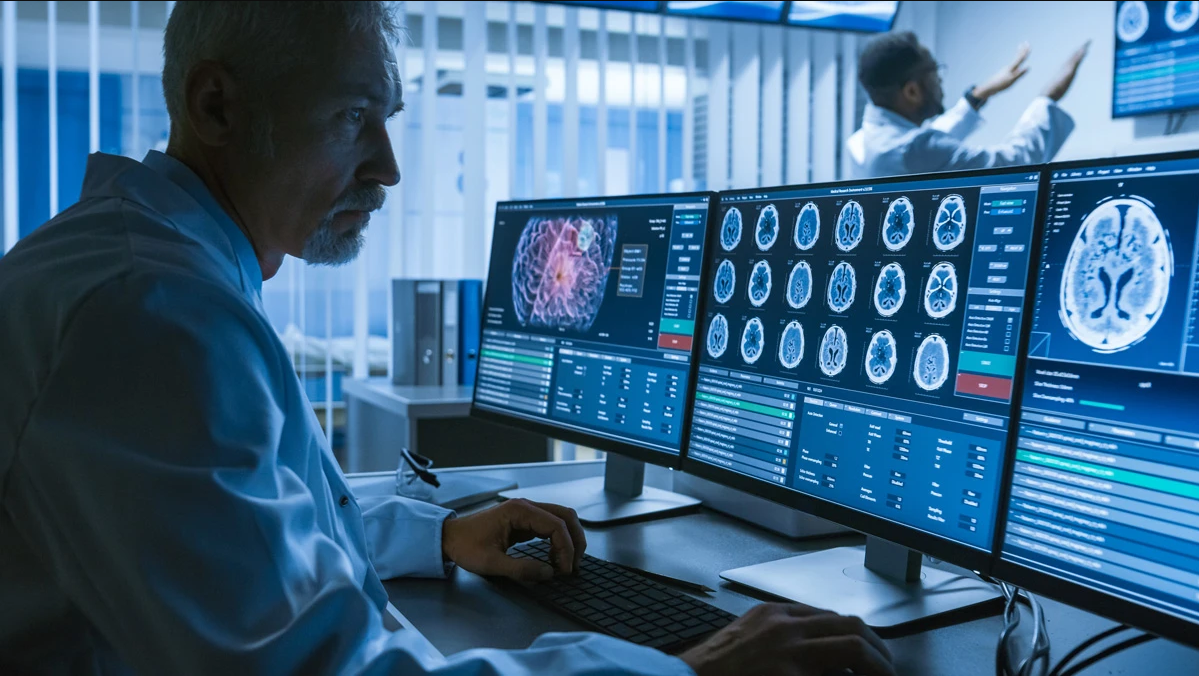
[July 14, 2022: Mira Lee, National Research Council of Science & Technology]
The research results are published as an inside back cover paper in Advanced Materials. (Credit: Korea Institute of Science and Technology (KIST))
An artificial tactile neuron device that quickly and accurately converts the stiffness of a substance. Combining with AI technology enables learning of the stiffness levels and distributions of the tumor, suggesting the possibility of cancer diagnosis.
The stiffness levels and distributions of various biological materials reflect disease-related information, from cells to tissues. For example, malignant breast tumors are usually stiffer and have a more irregular shape than benign breast tumors. Ultrasound elastography can non-invasively determine the degree and shape of the tissue stiffness and is used for diagnosing breast cancer owing to its low cost. However, the opinion of an experienced expert is essential for interpreting ultrasound elastography images, but different experts differ in accuracy.
The president of the Korea Institute of Science and Technology (KIST), Mr. Seok-Jin Yoon, announced that Dr. Hyunjung Yi's team at the spin convergence research center and Suyoun Lee, the director of the Center for Neuromorphic Engineering, had developed a simple but highly accurate disease diagnosis technology by combining tactile neuron devices with artificial neural network learning methods.
Unlike the previously reported artificial tactile neuron devices, this tactile neuron device can determine the stiffness of objects. Neuromorphic technology is a research field that aims to emulate the human brain's information processing method, which is capable of high-level functions while consuming a small amount of energy using electronic circuits.
Related Stories
Neuromorphic technology is gaining attention as a new data processing technology fit for AI, IoT, and autonomous driving, requiring the real-time processing of complex and vast information. Sensory neurons receive external stimuli through sensory receptors and convert them into electrical spike signals. Here, the generated spike pattern varies based on the external stimulus information. For example, higher stimulus intensity causes higher generated spike frequency.
The research team developed an artificial tactile neuron device with a simple structure that combines a pressure sensor and an ovonic threshold switch device to produce such sensory neuron characteristics. Applying pressure to the pressure sensor causes the sensor's resistance to decrease and the connected ovonic switch element's spike frequency to change. The developed artificial tactile neuron device is a high-response, high-sensitivity device that allows the pressing force to generate faster electrical spikes while improving the pressure sensitivity, which focuses on the fact that stiffer materials result in faster pressure sensing when pressed.
The electrical spike duration (or 1/frequency) generated by the developed device is less than 0.00001 s, which is more than 100,000 times faster than the several seconds it usually takes to press an object.
A schematic of spiking representation of stiffness and disease diagnosis using an artificial tactile neuron based on an OTS and a PRS. a) Components of the artificial tactile neuron based on an OTS and a PRS (bottom) along with the corresponding biological components (top). The PRS was designed to serve as an artificial mechanoreceptor of the OTS-based spiking tactile neuron. Upon pressing the PRS, the resistance of the PRS decreases and the frequency of the generated output spikes increases. b) Schematic illustration of the characteristic I–V curve of an OTS device, where Vth and VH are the threshold voltage and holding voltage, respectively. The arrows indicate the sequence of the I–V curve. c) Schematic of the spikes generated by the OTS+PRS composite device. d) Schematic of the rate coding of static pressure levels, with the spike frequency proportional to the intensity of the loaded pressure level. e) Schematic of the spike evolution patterns at two different levels of elastic stiffness of contacting material. f) Schematic of the classification of malignancy of tumors using the stiffness-encoded spike patterns and the reservoir computing-based SNN learning. (CREDIT: National Research Council of Science & Technology)
Additionally, while the existing devices could detect a low pressure (approximately 20 kPa, similar to a force of light pressing) with a spike frequency change of 20 to 40 Hz, the developed device can detect the low pressure with spike frequency changes of 1.2 MHz. This allows real-time conversion of changes in the pressing force into spikes.
Spiking representation of stiffness of various elastomers using the artificial tactile neuron. a) Various elastic moduli of fabricated elastomers. Mean values ± SD were obtained from three different samples for each group. b) Resistance values of the PRSs during the initial one second upon pressing up to 20 kPa through various materials of different elastic moduli. c) Representative spike evolution patterns generated from artificial tactile neurons upon pressing them through materials of different elastic moduli (see color legend in (b)). The artificial tactile neuron was applied with a voltage pulse train (amplitude = 6 V, base = 0 V, width = 100 μs, leading = 1 μs, falling = 1 μs, period = 40 ms) during pressing through the elastic material. The curves were shifted horizontally for clarity with the time for the spike-onset of each material being zero. d) FFT frequency for the various elastic materials (see color legend in (b)) during the initial two seconds upon pressing up to 20 kPa. The SFER (ψ) is also indicated. (CREDIT: National Research Council of Science & Technology)
To deploy the developed device to actual disease diagnosis, the research team used elastography images of malignant and benign breast tumors and utilized a spiking neural network learning method. Each pixel of the color-coded ultrasound elastography image which is correlated with the stiffness of the imaged material was converted into a spike frequency change value and used for training the AI. As a result, it was possible to determine the malignancy of a breast tumor with up to 95.8% accuracy.
The KIST research team stated, "the developed artificial tactile neuron technology is capable of detecting and learning mechanical properties with a simple structure and method." The team added, "Through follow-up research, it will be possible to solve the noise reflection issue, which is a disadvantage of ultrasound elastography if artificial tactile neurons can collect an object's elastography image obtainable using ultrasound elastography."
The team also expects the device to be helpful in low-power and high-accuracy disease diagnosis and applications such as robotic surgery where a surgical site needs to be quickly determined in an environment humans cannot directly contact."
For more science and technology news stories check out our New Discoveries section at The Brighter Side of News.
Note: Materials provided above by National Research Council of Science & Technology. Content may be edited for style and length.
Like these kind of feel good stories? Get the Brighter Side of News' newsletter.
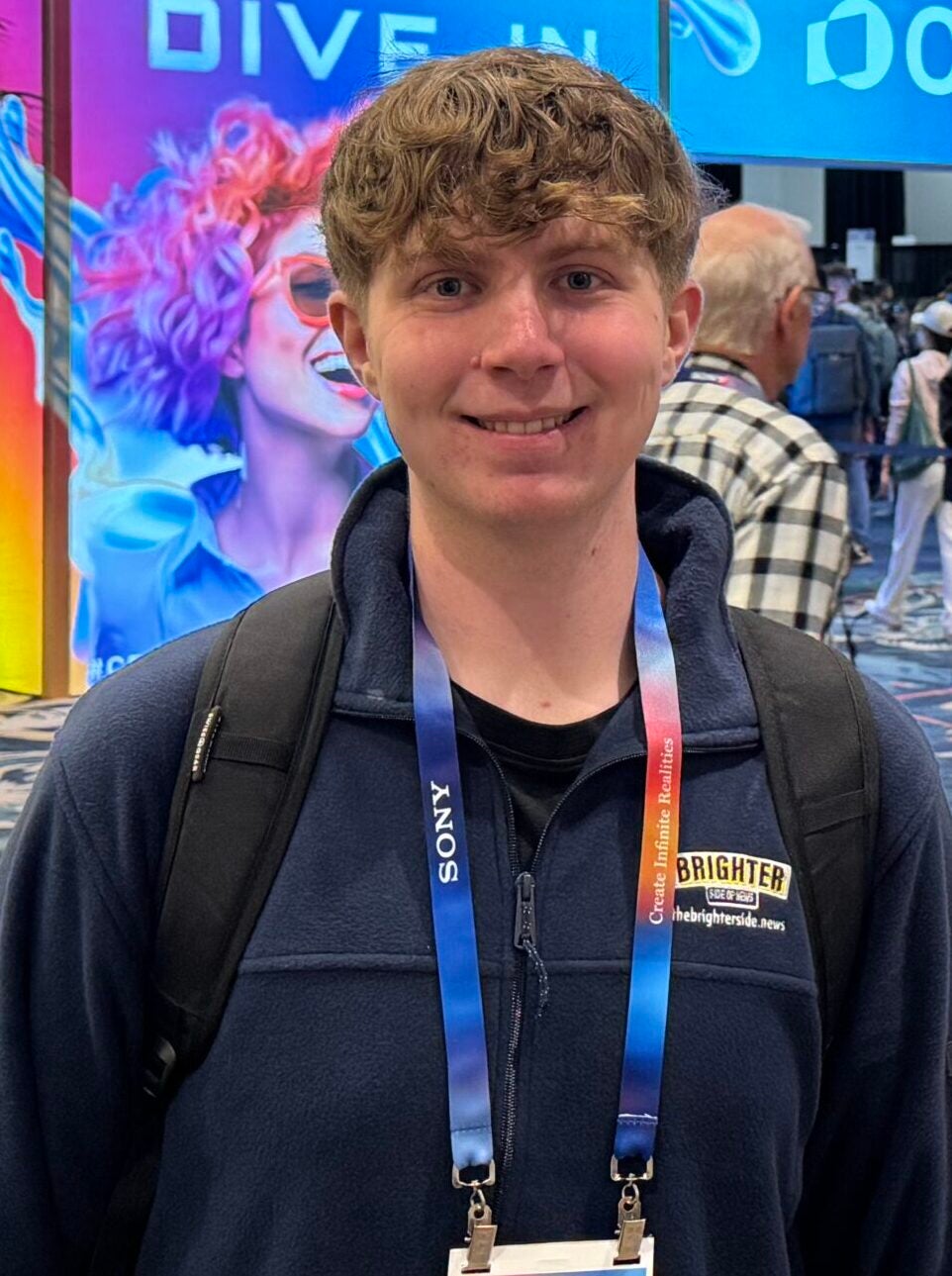
Joshua Shavit
Science & Technology Writer | AI and Robotics Reporter
Joshua Shavit is a Los Angeles-based science and technology writer with a passion for exploring the breakthroughs shaping the future. As a contributor to The Brighter Side of News, he focuses on positive and transformative advancements in AI, technology, physics, engineering, robotics and space science. Joshua is currently working towards a Bachelor of Science in Business Administration at the University of California, Berkeley. He combines his academic background with a talent for storytelling, making complex scientific discoveries engaging and accessible. His work highlights the innovators behind the ideas, bringing readers closer to the people driving progress.