Eco-driving reduces urban carbon emissions by up to 22%, MIT study finds
Eco-driving could reduce urban carbon emissions by up to 22%, delivering a promising path for sustainable traffic without compromising safety
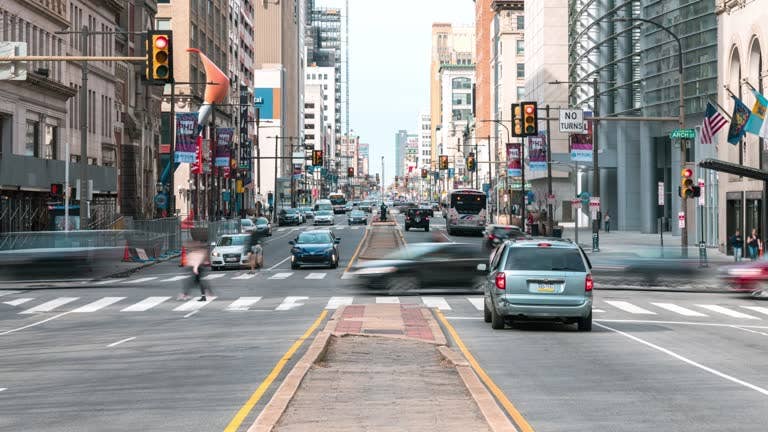
By programming vehicles to reduce stop-and-go traffic using intelligent speed adjustments, significant reductions in emissions can be achieved. (CREDIT: iStock)
The transportation sector poses a formidable challenge for decarbonization due to its sheer diversity and scale. Among the potential solutions, eco-driving through semi-autonomous vehicles offers an emerging opportunity to mitigate carbon emissions.
By programming vehicles to reduce stop-and-go traffic using intelligent speed adjustments, significant reductions in emissions can be achieved. A comprehensive study explored the effects of eco-driving across a wide variety of traffic scenarios, employing advanced modeling techniques, including large-scale scenario analysis and multi-task deep reinforcement learning.
This study revealed substantial potential for emission reductions at signalized intersections, setting a foundation for a broader shift toward cleaner transportation.
The research focused on simulating eco-driving scenarios at over 6,000 signalized intersections across three major U.S. cities, exploring a million different traffic situations. The results were impressive—optimized vehicle trajectories led to reductions of 11-22% in intersection carbon emissions.
This reduction level is equivalent to the national emissions of countries like Israel or Nigeria. Importantly, these benefits were achieved without negatively impacting traffic flow or safety, underscoring the effectiveness of this approach.
Interestingly, the study found that only 10% adoption of eco-driving technology yielded up to half of the total potential emission reductions, highlighting that early adopters can generate considerable benefits. In fact, 70% of the emission reduction could be attributed to just 20% of the intersections, suggesting a strategic path for a phased implementation.
Related Stories
However, the specific intersections that yield the highest benefits varied with different adoption rates, underscoring the need for careful deployment planning. Key factors like vehicle inflow, lane length, and speed limits played a significant role in determining the emission reduction potential, and these factors varied across different cities.
The method employed in this research involved three key steps: scenario modeling, eco-driving control, and impact assessment. First, the team modeled traffic scenarios to determine factors that influence eco-driving benefits, and pruned them to select a representative subset for training.
Using this subset, they trained a series of eco-driving policies using multi-task deep reinforcement learning. These policies were then deployed through a transfer learning approach to choose the most suitable policy for each scenario. Finally, the researchers conducted an impact assessment based on the learned behaviors across different intersections.
The study demonstrated that eco-driving vehicles learn to glide smoothly as they approach intersections, reducing the need to idle at traffic lights. This behavior significantly cuts emissions compared to typical human-driven vehicles that tend to stop and idle. For instance, a two-lane intersection scenario showed that human drivers usually stop at a red signal, while vehicles equipped with eco-driving systems glide and pass through without halting, minimizing wasted fuel and emissions.
In addition to emission reductions, the study also evaluated the safety of eco-driving behaviors. It compared the road safety metrics of eco-driving with those of conventional human driving using five key safety indicators: time to collision (TTC), post-encroachment time (PET), maximum speed, speed change, and deceleration rate.
Results indicated that the eco-driving behaviors were as safe as human driving due to the smoother maneuvers. However, since the behaviors of eco-driving vehicles differ from those of human drivers, additional studies are recommended to understand potential human-driver responses and avoid unintended consequences.
Factors such as speed limits, road grade, vehicle inflow, and lane length played a crucial role in influencing emission reductions. The study employed Pearson correlation analysis to identify these key factors, which revealed significant differences based on local traffic conditions.
For example, cities like Atlanta, with higher speed limits and longer lanes, saw greater benefits compared to cities like San Francisco, which have lower limits and shorter approaches. The findings also suggested that as eco-driving adoption grows, the factors influencing emissions evolve.
At lower adoption rates, features like signal timing ratios and lane count were more critical, while at higher adoption rates, vehicle speed and lane length became more significant. This dynamic relationship highlights the need for adaptive city planning and frequent updates to traffic signal timing guidelines.
The study also examined how to efficiently implement eco-driving by focusing on specific intersections. In all three cities analyzed, at least 70% of the emission benefits could be achieved by applying eco-driving at just 20% of the intersections. However, these intersections did not consistently overlap between different levels of eco-driving adoption, suggesting that infrastructure investments must be carefully planned.
For instance, which intersections should receive roadside units (RSUs) for communication with vehicles depends on current and future eco-driving adoption levels. The selection of these locations will have significant long-term value for eco-driving effectiveness, especially considering the budgetary constraints of transportation agencies.
Furthermore, the potential benefits of eco-driving were assessed alongside the growing adoption of hybrid and electric vehicles. While vehicle electrification is rapidly advancing, the energy savings from eco-driving behaviors contribute to emission reductions even for electric vehicles, since the electricity grid itself is not fully carbon-free.
Therefore, the combination of eco-driving with hybrid and electric vehicles presents a compelling strategy for further reducing carbon footprints, especially when coupled with other improvements like infrastructure optimization and better traffic signal coordination.
Eco-driving, especially in urban environments, has shown promise not just in reducing emissions but also in reshaping how cities plan their transportation infrastructure. With consistent emission benefits observed across various weather conditions and seasons, eco-driving offers a reliable year-round solution for emissions reduction. It also promotes a more efficient use of existing infrastructure by reducing fuel consumption without compromising safety or traffic throughput.
This study paves the way for large-scale analyses of other traffic externalities, such as time efficiency, road safety, and air quality. The use of multi-task deep reinforcement learning for optimizing eco-driving policies demonstrates the potential of artificial intelligence in solving complex urban challenges.
As cities strive to become more sustainable, such advanced solutions will play a critical role in reducing carbon emissions, enhancing air quality, and improving overall urban living conditions.
Note: Materials provided above by The Brighter Side of News. Content may be edited for style and length.
Like these kind of feel good stories? Get The Brighter Side of News' newsletter.