Cutting-edge AI model can determine your true age by looking at your chest
A chest radiograph, paired with the magic of advanced artificial intelligence (AI), might just redefine what it means to “look your age.”
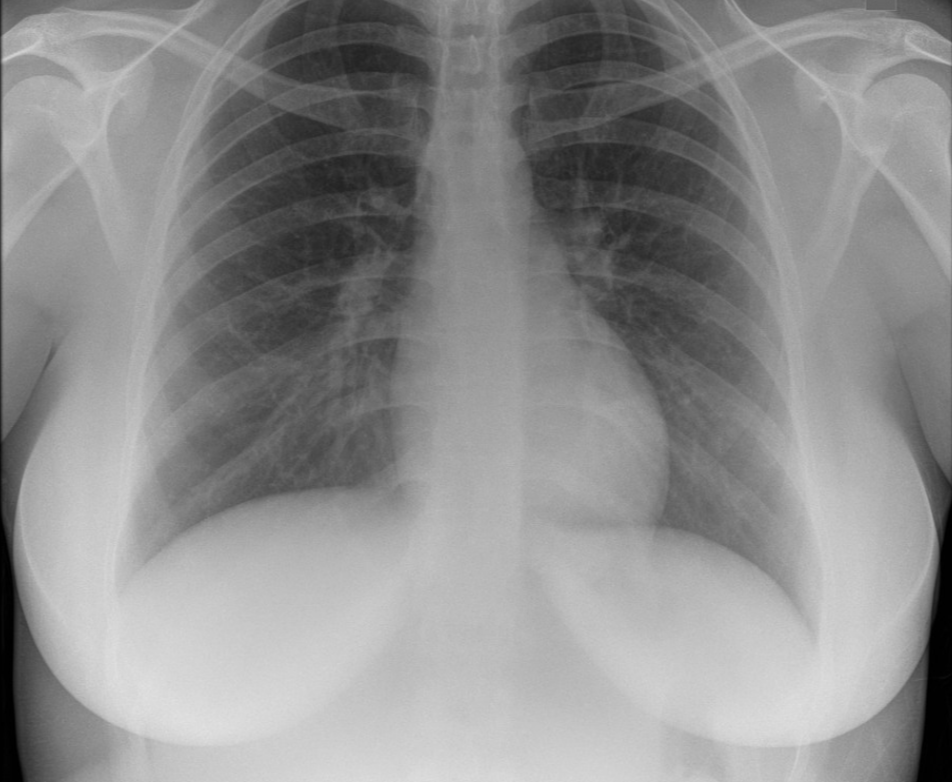
[Aug. 18, 2023: Staff Writer, The Brighter Side of News]
AniFaceDrawing system: Generating High-Quality Anime Portraits using AI. (CREDIT: Haoran Xie from JAIST)
In an age where every wrinkle and fine line on our faces is often scrutinized, scientists from Osaka Metropolitan University are steering the conversation towards a different direction: our chests.
According to their groundbreaking study, one's chronological age, commonly deduced from facial features, might not be the only—or even the most accurate—indicator of their overall health. Instead, a chest radiograph, paired with the magic of advanced artificial intelligence (AI), might just redefine what it means to "look your age."
The Next Leap in Medical Imaging
This innovative AI model, developed at Osaka Metropolitan University, uses chest radiographs not just to provide an estimate of an individual’s age but also to detect disparities that might hint at the presence of chronic diseases.
Such advancements are paving a revolutionary path for early disease detection and subsequent interventions. This groundbreaking study is set to make waves in the medical community with its impending publication in The Lancet Healthy Longevity.
Related Stories:
The forerunners of this research were graduate student Yasuhito Mitsuyama and Dr. Daiju Ueda from the Department of Diagnostic and Interventional Radiology at the Graduate School of Medicine, Osaka Metropolitan University.
The duo, alongside their team, began by developing a deep learning-based AI model that estimates age using chest radiographs of healthy individuals. Their next step was to test this model's accuracy on radiographs of patients with known diseases, delving deep into the correlation between AI-estimated age and specific diseases.
Aware of the challenges of training AI on a single dataset, especially the risk of overfitting, the team adopted a comprehensive approach, sourcing data from a myriad of institutions.
The upper images are the chest radiographs of patients from 21 to 40 years old and from 81 to 100 years old chronologically and the lower images are a visualization of the AI’s focus (both after averaging). Red indicates the points most useful for age determination. (Credit: Yasuhito Mitsuyama, Osaka Metropolitan University)
Data Collection and Impressive Results
The robust research process involved obtaining 67,099 chest radiographs between 2008 and 2021, sourced from 36,051 healthy individuals who had undergone health check-ups at three distinct facilities. The results? Astounding.
The AI's estimated age showcased a remarkable correlation coefficient of 0.95 when juxtaposed with the chronological age. For the uninitiated, a correlation coefficient above 0.9 is generally considered phenomenally strong, underscoring the reliability of this novel method.
Scatterplots (each including a regression line) of ground truth and predicted values of the artificial intelligence model for the internal dataset from institution A. (Credit: Yasuhito Mitsuyama, Osaka Metropolitan University)
Yet, the team wasn't done. They went further, amassing an additional 34,197 chest radiographs from as many patients with known diseases across two institutions. Their discoveries reaffirmed their hypotheses. Discrepancies between the AI-estimated age and an individual’s actual chronological age signaled a higher likelihood of several chronic ailments, notably hypertension, hyperuricemia, and chronic obstructive pulmonary disease.
A New Outlook on Age and Health
Mr. Mitsuyama reflected on the implications of their research, stating, “Chronological age is one of the most critical factors in medicine. Our results suggest that chest radiography-based apparent age may accurately reflect health conditions beyond chronological age."
Average saliency images of each 20-year chronological age group in the external test dataset from institution C. The top panels show averaged chest radiographs for all participants in the group and the bottom panels show averaged saliency maps. Hot areas in the saliency maps indicate characteristics of increasing age in chest radiographs; cold areas indicate characteristics of decreasing age. (Credit: Yasuhito Mitsuyama, Osaka Metropolitan University)
He further elucidated the team's ambitions, highlighting their aspiration "to further develop this research and apply it to estimate the severity of chronic diseases, predict life expectancy, and even forecast possible surgical complications."
Osaka Metropolitan University's research is a testament to the strides AI is making in the realm of healthcare. By harnessing the untapped potential of chest radiographs, we might soon be shifting the paradigm on aging and health, spotlighting the chest as an equally (if not more) significant barometer of our overall well-being than our faces. As technology and healthcare continue to intertwine, the adage "don't judge a book by its cover" might soon be applicable to how we perceive age and health.
Note: Materials provided above by The Brighter Side of News. Content may be edited for style and length.
Like these kind of feel good stories? Get the Brighter Side of News' newsletter.
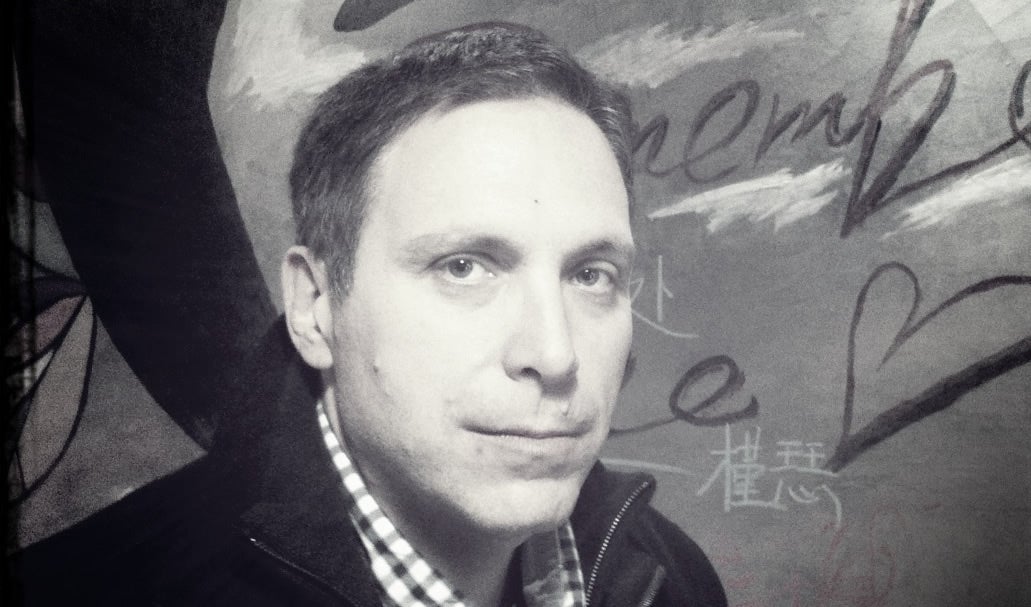