Cutting-edge AI is transforming deadly skin cancer detection
AI is revolutionizing skin cancer diagnosis with 94% accuracy, improving early detection, reducing costs, and saving lives worldwide.
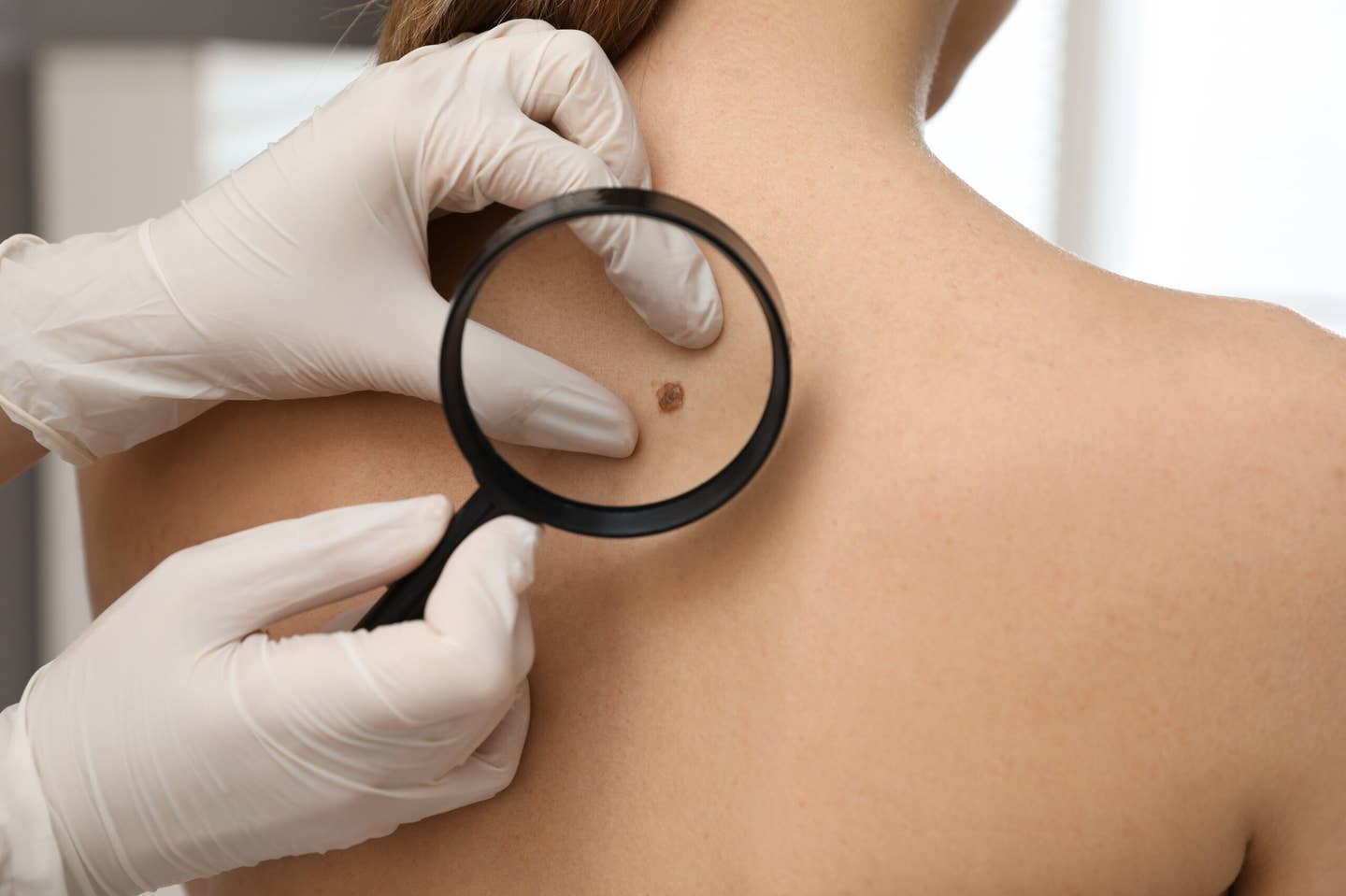
AI-driven innovations in dermatology enhance skin cancer detection with groundbreaking accuracy, promising earlier diagnoses, reduced costs, and improved patient care. (CREDIT: Shutterstock)
Skin cancer, the most common cancer worldwide, presents a unique challenge due to its varied manifestations. Each year, millions of cases are diagnosed, with the disease impacting people across diverse regions and demographics.
While nonmelanoma skin cancers (NMSCs) are prevalent, melanoma, though less common, is significantly more lethal due to its aggressive nature and tendency to metastasize rapidly.
In 2022, nearly 20 million new cancer cases were reported globally, with 9.7 million cancer-related deaths. Skin cancer alone accounted for a significant portion, with melanoma responsible for approximately 55,500 annual deaths. Despite accounting for only 1% of skin cancer cases, melanoma causes the majority of fatalities.
One in five individuals will develop some form of cancer in their lifetime, and skin cancer is particularly common among Caucasians, where both melanoma and NMSCs pose significant health risks.
The primary risk factor for skin cancer is prolonged exposure to ultraviolet (UV) rays, with research showing that 86% of melanoma cases are linked to excessive UV exposure. While darker skin tones offer some protection due to higher melanin levels, no one is entirely immune.
Early detection remains crucial, as the five-year survival rate for melanoma can reach 95% if diagnosed early. However, diagnosing skin cancer remains challenging due to the striking similarities between benign and malignant lesions.
Skin cancer’s global impact underscores the need for heightened awareness and improved diagnostic methods. According to the World Health Organization, skin cancer ranks among the top three most common cancers worldwide. Nonmelanoma cases alone contribute to over 2 million diagnoses annually. This staggering number highlights the urgent need for effective strategies to combat the disease.
Traditional diagnostic methods rely heavily on visual inspection and dermoscopy. The ABCDE acronym—asymmetry, border irregularity, color variation, diameter over 6mm, and evolution—helps identify suspicious lesions. However, even experienced dermatologists face difficulties.
Without dermoscopic training, diagnostic accuracy averages 60%, improving to 75-84% with experience and the use of dermoscopes. Yet, the process is time-consuming and prone to errors.
Studies have shown that dermatologists with over a decade of experience achieve 80% diagnostic accuracy, compared to 62% for those with 3-5 years of experience. This variability underscores the need for improved diagnostic tools. Misdiagnosis can lead to unnecessary biopsies or delayed treatment, emphasizing the importance of early and accurate detection.
Related Stories
Clinical evaluations often combine visual inspections with tools like dermoscopy and, in some cases, histopathological examinations. These methods, while effective, require significant training and experience to achieve consistent accuracy.
For example, dermoscopy—a technique that examines skin lesions with magnification—has become a critical tool. Yet even this advanced method has limitations, as it relies heavily on the clinician’s skill.
Published in the journal, Data Science and Management, recent advances in artificial intelligence (AI) have revolutionized dermatology. AI systems, particularly those using convolutional neural networks (CNNs), offer promising solutions for skin cancer detection.
By analyzing dermoscopic images, CNNs can classify skin lesions with accuracy comparable to, or better than, experienced dermatologists. In seven out of 11 studies, AI demonstrated superior diagnostic performance.
A notable study compared a CNN model with 145 dermatologists across 12 German hospitals. The CNN achieved a sensitivity of 92.8% and specificity of 61.1%, outperforming most participants. This highlights the potential of AI to enhance diagnostic accuracy and reduce the burden on medical professionals.
Deep learning models, particularly transfer learning (TL), have further advanced AI’s capabilities. TL leverages pre-trained models to improve performance on new tasks, even with limited data. By applying techniques like test-time augmentation (TTA), which enhances datasets through random image modifications,
AI systems become more robust and versatile. These innovations enable models to generalize across diverse lesion types, improving accuracy and reliability.
AI’s ability to process vast amounts of data efficiently makes it particularly suited for dermatology. Unlike traditional methods, AI systems can analyze thousands of images in minutes, identifying patterns and anomalies that might escape human observation. This speed and precision are invaluable in clinical settings, where early detection can significantly impact patient outcomes.
A pioneering study by researchers at Ahmadu Bello University introduced an ensemble AI model combining five state-of-the-art transfer learning architectures: MobileNet, EfficientNetV2B2, Xception, ResNext101, and DenseNet201. Trained on the HAM10000 dataset of over 10,000 dermoscopic images, the model achieved 94.49% accuracy in classifying skin lesions into seven categories, including melanoma and basal cell carcinoma.
The study’s innovative approach weighted each model based on performance, ensuring superior accuracy during ensemble predictions. TTA further improved outcomes by increasing the dataset’s diversity, allowing the AI system to better identify subtle differences between benign and malignant lesions. Metrics such as precision (95.07%) and recall (94.49%) underscore the model’s reliability and potential clinical utility.
“The integration of deep learning in dermatology is not just an advancement; it’s a necessity,” said lead researcher Aliyu Tetengi Ibrahim. “Our model’s high accuracy rate can reduce unnecessary biopsies and promote earlier detection, ultimately saving lives by helping dermatologists make more informed decisions.”
The model’s ability to classify lesions into specific categories marks a significant leap forward. By identifying conditions such as actinic keratoses, benign keratosis, and vascular skin lesions, it provides a comprehensive diagnostic tool. This capability ensures that patients receive accurate diagnoses, reducing the risk of misclassification and inappropriate treatments.
AI-driven diagnostic tools offer immense potential to revolutionize dermatology. By streamlining diagnosis, reducing healthcare costs, and improving accuracy, these technologies address critical gaps in skin cancer detection. Telemedicine platforms integrating AI systems can democratize access to advanced diagnostics, especially in underserved regions lacking dermatological expertise.
The benefits extend beyond diagnosis. AI can aid in monitoring treatment responses, predicting patient outcomes, and guiding personalized therapies. By alleviating the burden on healthcare systems, AI paves the way for more efficient, accessible, and affordable care. As the prevalence of skin cancer rises, embracing innovative technologies becomes imperative to save lives and enhance global health.
AI’s role in healthcare is expanding rapidly. Beyond dermatology, its applications span various medical fields, including radiology, oncology, and cardiology. These advancements highlight AI’s transformative potential, not just as a diagnostic tool but as a cornerstone of modern medicine. By integrating AI into routine clinical practice, healthcare providers can offer more precise, timely, and effective care.
In conclusion, the fight against skin cancer has entered a new era, with AI leading the charge. Its ability to improve diagnostic accuracy, reduce costs, and enhance patient care represents a significant step forward.
As research continues to evolve, the integration of AI into dermatology promises to save lives and redefine the future of cancer detection and treatment.
Note: Materials provided above by The Brighter Side of News. Content may be edited for style and length.
Like these kind of feel good stories? Get The Brighter Side of News' newsletter.
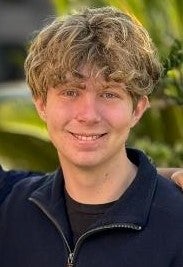