Cutting-edge AI finds 44 Earth-like worlds that may support life
Scientists trained an AI to predict where Earth-like planets might exist, helping speed up the search for habitable worlds.
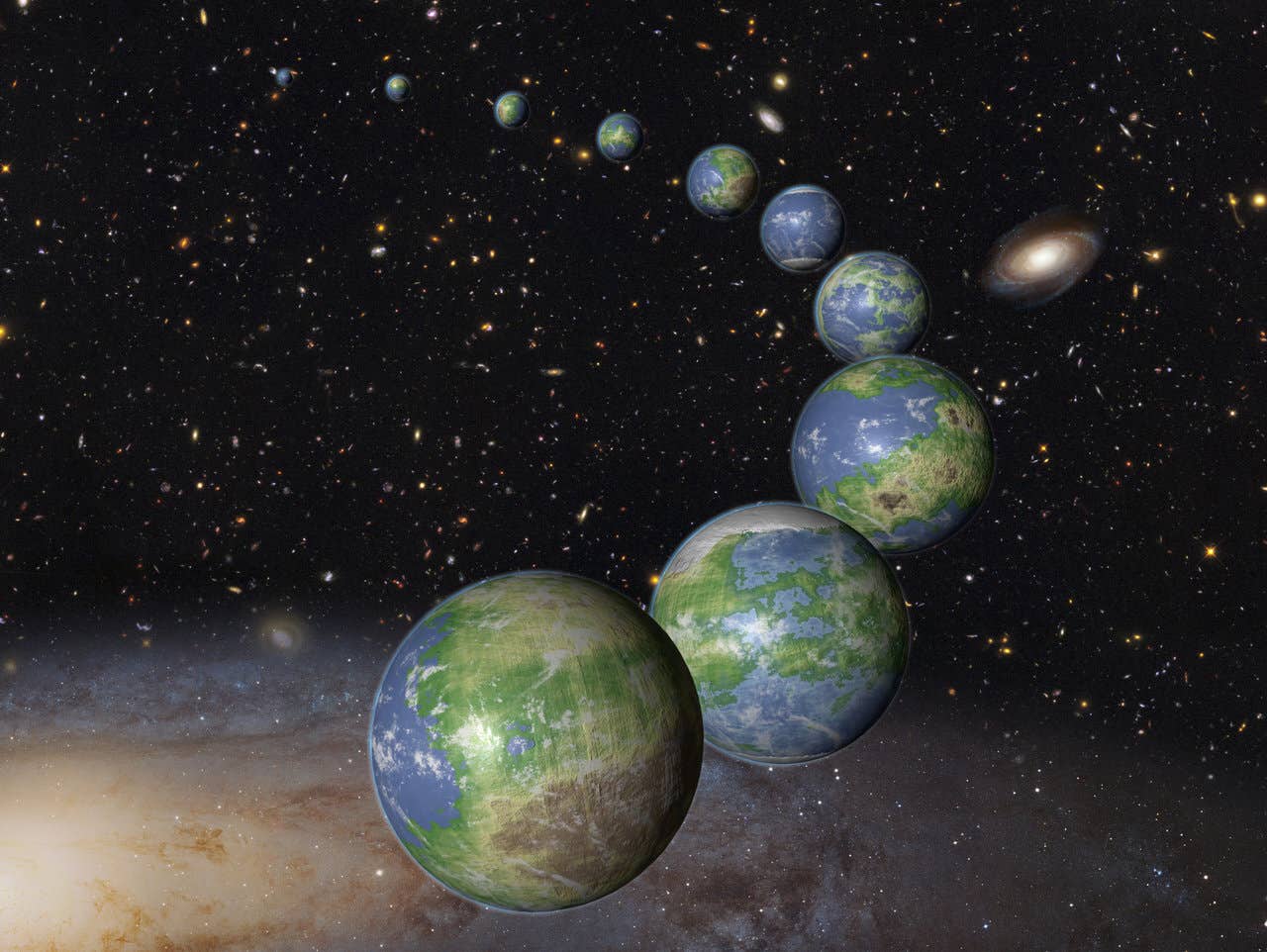
AI model predicts where Earth-like planets may be hiding, boosting search for life beyond our solar system. (CREDIT: NASA)
Astronomers have spent decades trying to find Earth-like planets beyond our solar system. These worlds are small, cold, and difficult to detect, especially when they lie in the "temperate zone"—the sweet spot around a star where conditions may support life.
New tools like the PLATO mission and proposed LIFE mission promise to aid the search, but they take time. A full year of observations might be needed to detect just one planet. So, to speed things up, researchers have started thinking differently: what if machines could help point telescopes in the right direction?
A team based at the University of Bern and the National Centre of Competence in Research PlanetS has trained an artificial intelligence system to do just that. The model doesn’t look at the night sky—it studies the structure of known planetary systems to make smart guesses about where undiscovered Earth-like planets might be hiding.
The results are promising. In tests, this machine learning algorithm correctly identified Earth-like planets with 99% accuracy, offering a major leap forward in the hunt for habitable worlds.
What planetary architecture can reveal
The search for planets outside our solar system—exoplanets—has revealed that many stars host multiple planets. These planetary systems aren’t arranged randomly. Instead, patterns often emerge, such as the “peas-in-a-pod” structure, where planets of similar size and spacing form together. Studies like those by Lissauer, Millholland, Weiss, and others show that a planet’s size, mass, and position often depend on its neighbors.
Other researchers have even found that the presence of a small rocky planet close to a star may hint at a larger gas giant further out.
In contrast, the presence of a "hot Jupiter"—a massive planet close to its star—seems to break up those regular planet patterns. These clues suggest that when you spot one planet, you might be able to predict others that are harder to detect.
Related Stories
Teaching machines to see what we can’t
Previous efforts to predict hidden planets relied on trends in observed data, like orbital spacing laws or statistical averages. But these methods come with limitations. For one, the catalog of known planetary systems is still small—fewer than 5,000 by mid-2024. And even among those, we have only spotted Earth-like planets in about two dozen systems. That’s just 0.5% of all known systems.
Because Earth-like planets are small and cold, they don’t block much starlight or tug strongly on their stars—making them almost invisible to current methods. Machine learning requires huge amounts of training data, which is a problem when only a few of these planets are confirmed. So the Bern team turned to simulations.
They used the Bern Model of Planet Formation and Evolution, a well-established computer model that simulates how planets form and change over time in their early environments. The model includes many of the physical processes involved in forming planetary systems, allowing scientists to create thousands of synthetic systems where every planet is known.
Dr. Jeanne Davoult, now at the German Aerospace Center in Berlin, built the machine learning algorithm as part of her PhD at the University of Bern. The team trained the model on synthetic systems generated by the Bern Model. These fake systems are designed to closely resemble real ones in structure and behavior.
Yann Alibert, co-director of the university’s Centre for Space and Habitability, explains, “The Bern Model is one of the only models worldwide that offers such a wealth of interrelated physical processes and enables a study like the current one to be carried out.”
Learning from the innermost planet
Once trained, the model looked at over 1,600 observed star systems, each of which already had at least one known planet. The algorithm was especially interested in the innermost detectable planet, or IDP, in each system. It found that the mass and orbit of this planet gave strong clues about whether an Earth-like planet might also be present further out.
From this analysis, the model flagged 44 star systems that it believes likely contain an Earth-like planet we haven’t found yet. These systems orbit stars of types G, K, or M—G being like our Sun, with K and M types being smaller and cooler.
The researchers didn’t stop there. They ran more detailed simulations and confirmed that the predicted planets could, in theory, exist within those systems. While this doesn’t prove the planets are real, it provides a valuable roadmap for future missions to follow.
Dr. Davoult says, “The model identified 44 systems that are highly likely to harbor undetected Earth-like planets. A further study confirmed the theoretical possibility for these systems to host an Earth-like planet.”
A faster route to finding life
If the model’s predictions hold up in real observations, it could transform how astronomers look for habitable worlds. Instead of scanning the sky blindly, they could focus on the systems flagged by the machine learning model, saving precious time and telescope resources.
This approach could be a game-changer for upcoming missions like PLATO and future concepts like LIFE, both of which aim to find and study small, cold planets. These missions are incredibly sensitive but also slow. Even one year of observing time can result in just a few promising discoveries. Targeting the most likely systems first makes the search much more efficient.
Romain Eltschinger, a PhD student and co-author of the study, helped expand the model’s reach. He says, “These results are important for the scientific community, and particularly for future space missions.”
So far, most confirmed exoplanets are large and close to their stars, simply because they’re easier to detect. Earth-like planets are often too faint and too far out. But with smarter tools like this AI model, researchers may soon be able to uncover many more planets like our own.
Dr. Alibert adds, “This is a significant step in the search for planets with conditions favorable to life and, ultimately, for the search for life in the universe.”
Model limitations and what’s next
While the model is powerful, it’s not perfect. For instance, it doesn’t yet fully reproduce certain patterns observed in real star systems. For example, many Sun-like stars have both Super-Earths and Cold Jupiters—giant planets orbiting far from their star. The synthetic data doesn’t always capture this.
Also, the fake systems tend to produce planets that are closer to their stars than those we’ve actually observed. So, the model’s predictions may need adjusting as real-world data improves.
Still, this study shows the value of combining computer simulations with machine learning. Instead of guessing where Earth-like planets might be, researchers can now make educated predictions backed by data.
That’s a big deal. The universe is vast. Telescopes can’t look everywhere at once. But with AI-powered tools, we can finally start narrowing down the list of places that just might be another Earth.
Research findings are available online in the journal Astronomy & Astrophysics.
Note: The article above provided above by The Brighter Side of News.
Like these kind of feel good stories? Get The Brighter Side of News' newsletter.
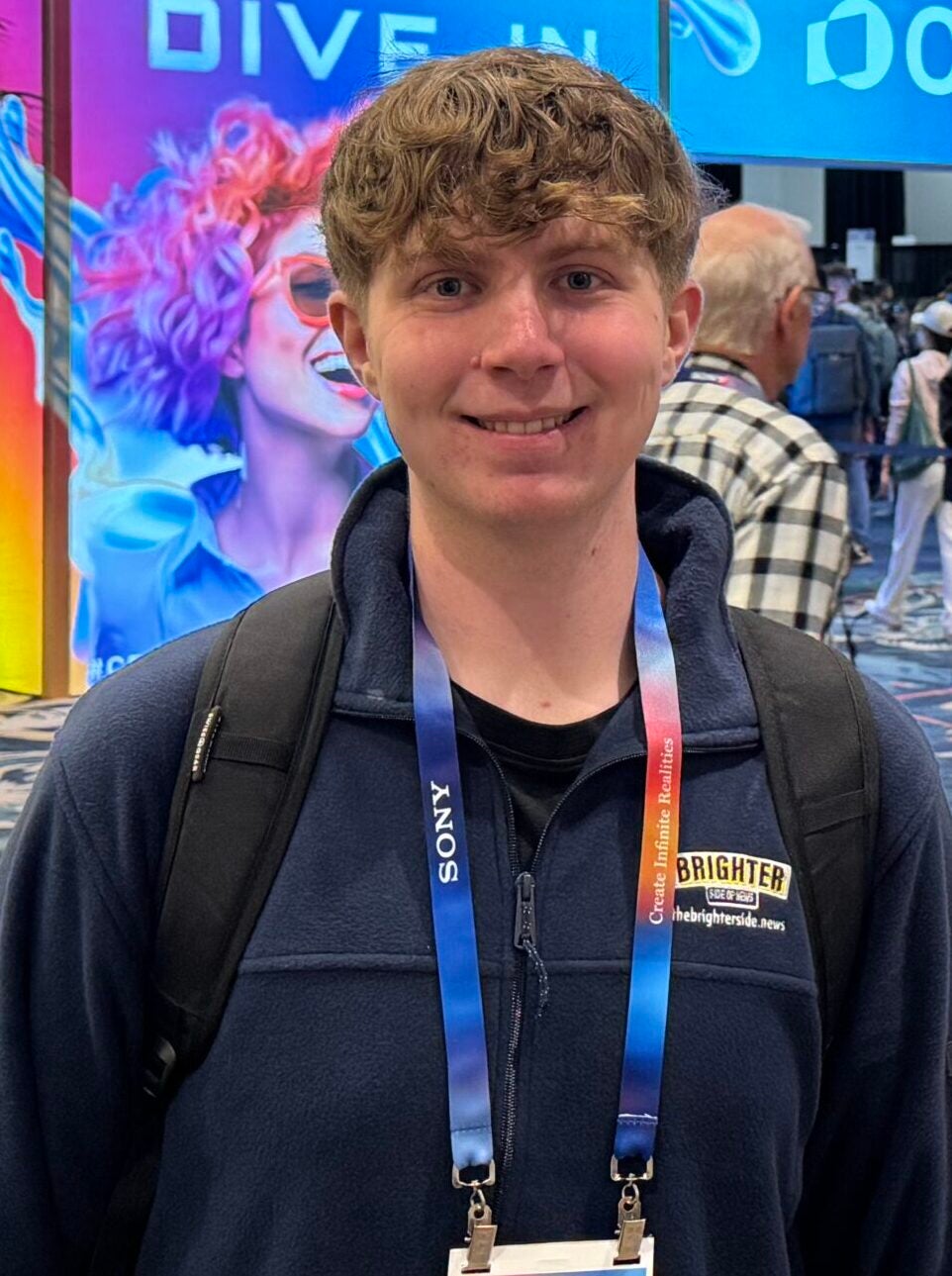
Joshua Shavit
Science & Technology Writer | AI and Robotics Reporter
Joshua Shavit is a Los Angeles-based science and technology writer with a passion for exploring the breakthroughs shaping the future. As a contributor to The Brighter Side of News, he focuses on positive and transformative advancements in AI, technology, physics, engineering, robotics and space science. Joshua is currently working towards a Bachelor of Science in Business Administration at the University of California, Berkeley. He combines his academic background with a talent for storytelling, making complex scientific discoveries engaging and accessible. His work highlights the innovators behind the ideas, bringing readers closer to the people driving progress.