Cutting-edge AI digs through old maps to find lost oil and gas wells
AI and historical maps are uncovering undocumented orphaned oil wells, addressing climate risks and improving methane emission mitigation strategies.
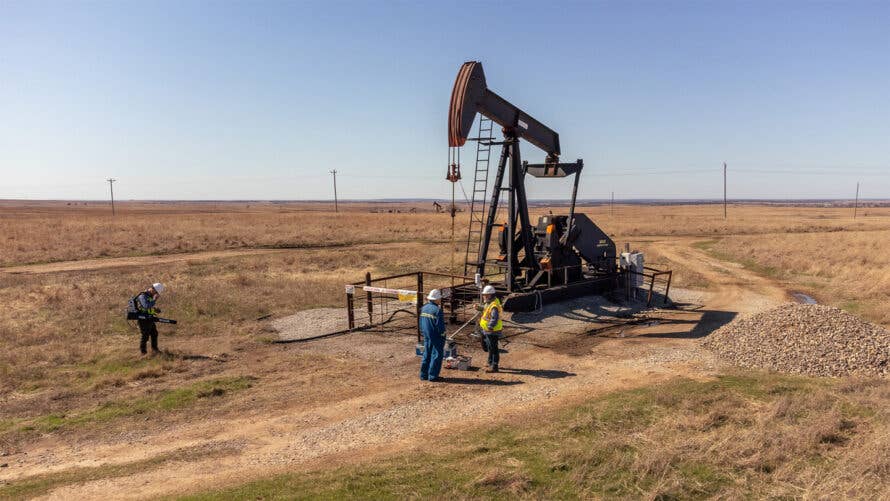
Members of CATALOG partner with industry to collect methane samples at a known well site in Oklahoma. (CREDIT: Jeremy Snyder / Berkeley Lab)
The United States is home to a staggering number of abandoned oil and gas wells, remnants of nearly two centuries of hydrocarbon extraction. Many of these wells, classified as undocumented orphaned wells (UOWs), are neither recorded in official databases nor tied to a responsible operator.
These hidden relics of the nation’s energy history pose significant environmental and public health risks, including methane emissions and water contamination.
Methane, a potent greenhouse gas, traps heat about 28 times more effectively than carbon dioxide over a century. Leaks from orphaned wells can accelerate climate change while releasing toxic substances like hydrogen sulfide into the air and contaminating nearby water supplies.
Addressing these risks begins with locating the wells—a daunting task, given the estimated 310,000 to 800,000 undocumented orphaned wells scattered across the U.S.
A Historical Map Meets Artificial Intelligence
To tackle this problem, researchers have turned to an unlikely ally: historical maps. Since 2011, the U.S. Geological Survey (USGS) has digitized 190,000 topographic maps dating back to 1884. These georeferenced maps provide critical clues about early oil and gas drilling activities. The challenge, however, is sifting through this vast archive to pinpoint potential UOW locations.
Recent advancements in artificial intelligence (AI) have revolutionized this process. Fabio Ciulla, a postdoctoral fellow at the Lawrence Berkeley National Laboratory, emphasizes the value of combining old data with cutting-edge technology.
“AI enhances our understanding of the past by extracting information from historical data on a scale that was unattainable just a few years ago,” Ciulla explains.
Related Stories
At the core of this effort is the U-Net convolutional neural network, a sophisticated algorithm designed to identify oil and gas well symbols on these maps.
Researchers manually labeled wells on 100 maps to train the AI, teaching it to recognize the distinct hollow black circles used to denote wells. This approach allows the AI to distinguish between actual wells and similar shapes, such as cul-de-sacs or the letter "o."
Mapping the Wells
The AI algorithm was applied to quadrangle maps, a subset of the USGS archive featuring consistent symbols for wells. This analysis focused on four counties with significant oil production histories: Kern and Los Angeles in California and Osage and Oklahoma in Oklahoma. In these regions, the AI identified 1,301 potential UOWs.
Verification of these findings involves a two-step process. First, researchers use satellite images and historical aerial photographs to search for visual evidence, such as pump jacks or storage tanks.
If surface clues are absent, field teams deploy magnetometers, instruments that detect buried metal well casings by measuring disturbances in magnetic fields. In verified cases, the AI proved remarkably accurate, locating wells within an average of 10 meters of their predicted positions.
The AI method represents a significant breakthrough. Traditional approaches, such as manual record searches or ground surveys, are time-intensive and geographically limited. By contrast, this AI-driven framework can analyze vast regions quickly, providing state agencies with a powerful tool to locate undocumented wells and prioritize them for plugging.
Charuleka Varadharajan, a senior scientist at Berkeley Lab, highlights the conservative nature of the approach. “We intentionally chose to have more false negatives than false positives,” she says. “This ensures careful identification of individual well locations.” While the current estimates are likely undercounts, refining the AI could uncover additional wells.
Beyond Maps: Drones, Sensors, and Citizen Science
The AI mapping effort is just one component of the Consortium Advancing Technology for Assessment of Lost Oil & Gas Wells (CATALOG), a nationwide initiative to address the orphaned well crisis. This collaborative project involves multiple national laboratories and local stakeholders, including Native American tribes and environmental agencies.
CATALOG is developing diverse tools to detect and measure methane emissions, assess well conditions, and streamline plugging efforts.
Drones equipped with methane sensors or magnetometers can survey large areas autonomously, identifying leaks and buried wells. Other methods, like hyperspectral imaging and thermal cameras, provide additional layers of data to locate wells and assess emissions.
In one innovative approach, researchers are exploring how smartphone magnetometers can help citizens contribute to the search for orphaned wells. This crowdsourced effort complements professional surveys, expanding the reach of detection technologies.
Methane detection and mitigation are central to the project. High-tech sensors, while effective, are often prohibitively expensive. Researchers are developing lower-cost alternatives to quickly categorize leaks, enabling agencies to prioritize high-emission wells for immediate action.
“We don’t need exact measurements,” explains Berkeley Lab scientist Sebastien Biraud. “We need to know if a well is leaking and whether it’s a small or significant amount.”
Quantifying emissions before and after plugging wells is crucial for verifying the effectiveness of these efforts. Properly plugging a well involves filling its borehole with cement to prevent oil, gas, or chemicals from escaping into the environment. This step not only reduces local hazards but also contributes to global climate mitigation strategies.
Collaboration with local stakeholders is vital for the program’s success. In the Osage Nation, where oil drilling dates back to the early 20th century, tribal officials are working closely with researchers to refine detection techniques and address data gaps.
“Utilizing AI and state-of-the-art equipment has streamlined various processes within our orphan well program,” says Craig Walker, director of Osage Nation Natural Resources.
The techniques pioneered by CATALOG are scalable and adaptable, making them applicable to other regions and well types. By integrating historical data with modern technology, the project represents a unique convergence of old and new.
As Ciulla notes, “Maps, something that seems so old-fashioned and static, can give us so much useful information when used correctly with the help of current technology.”
A Path to a Cleaner Future
While much work remains, the progress made by CATALOG offers hope for addressing the environmental and health risks posed by undocumented orphaned wells. By combining AI, advanced sensors, and community partnerships, researchers are not only uncovering the hidden legacy of America’s oil boom but also paving the way for a cleaner, safer future.
“We, as a society, really like energy,” Biraud says. “But we need to find solutions that limit our emissions. This is one way we can have an impact.”
The United States is home to a staggering number of abandoned oil and gas wells, remnants of nearly two centuries of hydrocarbon extraction. Many of these wells, classified as undocumented orphaned wells (UOWs), are neither recorded in official databases nor tied to a responsible operator.
These hidden relics of the nation’s energy history pose significant environmental and public health risks, including methane emissions and water contamination.
Methane, a potent greenhouse gas, traps heat about 28 times more effectively than carbon dioxide over a century. Leaks from orphaned wells can accelerate climate change while releasing toxic substances like hydrogen sulfide into the air and contaminating nearby water supplies.
Addressing these risks begins with locating the wells—a daunting task, given the estimated 310,000 to 800,000 undocumented orphaned wells scattered across the U.S.
Note: Materials provided above by The Brighter Side of News. Content may be edited for style and length.
Like these kind of feel good stories? Get The Brighter Side of News' newsletter.
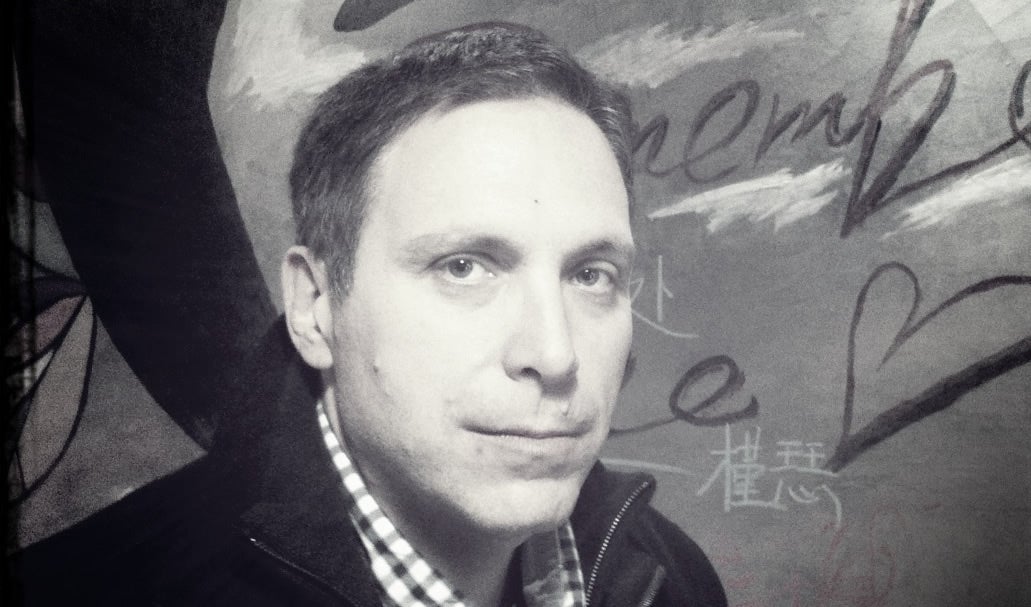