Cutting-edge AI can detect dark matter hidden within the cosmic noise of space
A breakthrough in AI has allowed scientists to separate dark matter self-interactions from AGN feedback, offering a new tool for uncovering dark matter’s mysteries.
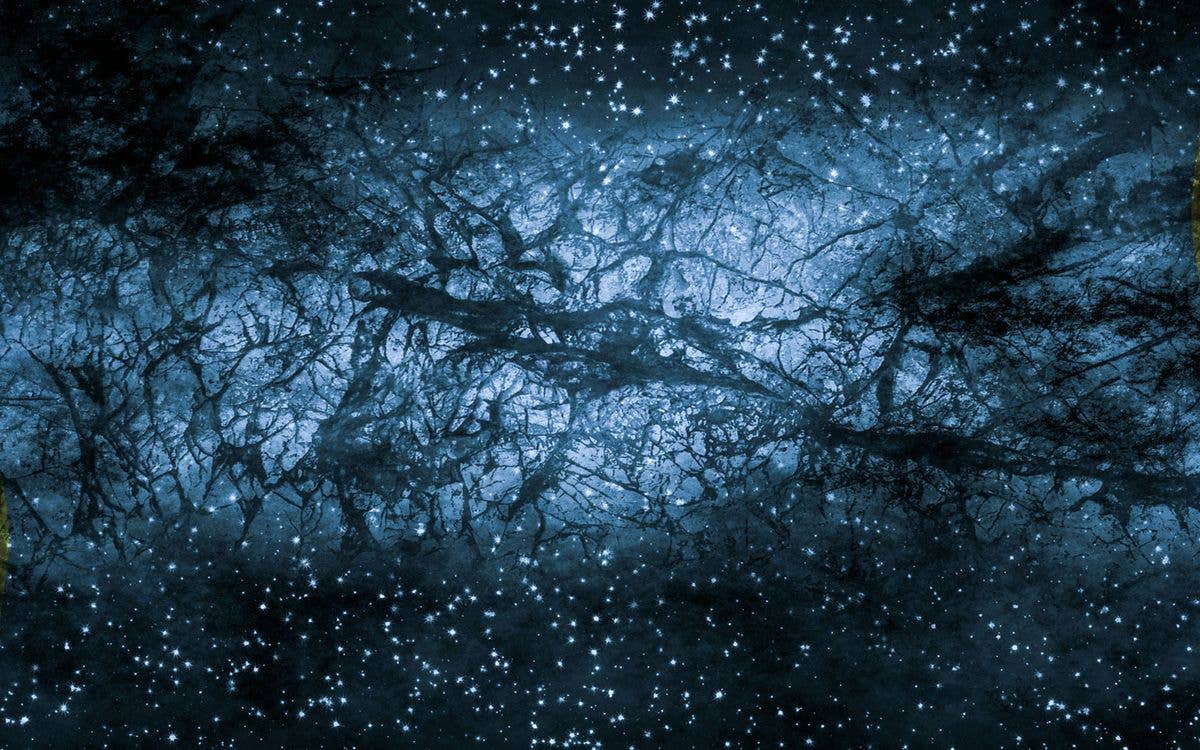
Dark matter, an invisible force thought to hold the universe together, is believed to make up about 85% of all matter. (CREDIT: CC BY-SA 3.0)
Dark matter, an invisible force thought to hold the universe together, is believed to make up about 85% of all matter and around 27% of the universe's total contents.
Even though dark matter has such a large presence, it remains undetectable by direct observation. Instead, scientists study its gravitational influence on galaxies and other cosmic structures. Despite decades of research, its true nature remains one of the most intriguing mysteries in physics.
One leading theory suggests that dark matter consists of particles that hardly interact with ordinary matter, apart from gravity. However, some scientists propose that these particles might occasionally interact with each other—a process called self-interaction.
Proving this theory would provide critical insight into dark matter’s properties and behavior. Yet, detecting these interactions presents a major challenge.
The challenge lies in distinguishing the subtle effects of dark matter self-interactions from other cosmic phenomena. Active galactic nuclei (AGN), for example, are supermassive black holes located at the centers of galaxies. AGNs can release tremendous energy that pushes matter around, mimicking the effects of dark matter. This overlap makes it extremely difficult for scientists to separate the two, leaving the nature of dark matter an ongoing enigma.
Related Stories
In an exciting development, astronomer David Harvey from EPFL’s Laboratory of Astrophysics has pioneered an innovative method to solve this problem. Using a deep-learning algorithm, Harvey’s approach is designed to separate the signals of dark matter self-interactions from those caused by AGN feedback.
The key tool here is an artificial intelligence (AI) model that analyzes images of galaxy clusters—vast collections of galaxies bound by gravity. This cutting-edge method could significantly improve the precision of dark matter research.
Harvey trained a Convolutional Neural Network (CNN), a type of AI known for recognizing patterns in visual data, to analyze images from the BAHAMAS-SIDM project. This project simulates galaxy clusters under different dark matter and AGN feedback conditions. By processing thousands of these simulated images, the CNN learned to distinguish between signals resulting from dark matter self-interactions and those from AGN feedback.
Among the various AI models tested, one known as "Inception" stood out as the most effective. This model was trained using two main dark matter scenarios that featured different levels of self-interaction. After training, the algorithm was validated with additional, more complex models, including one involving velocity-dependent dark matter, which varies in behavior based on its speed.
Under ideal conditions, the Inception model achieved an impressive 80% accuracy in identifying whether galaxy clusters were influenced by self-interacting dark matter or AGN feedback. Remarkably, the AI maintained its high performance even when realistic observational noise—simulating the data expected from future telescopes like Euclid—was introduced into the testing environment. This suggests that the algorithm can handle real-world data, making it a reliable tool for upcoming dark matter research.
What makes this breakthrough particularly exciting is the AI's potential to process vast amounts of data efficiently. As new telescopes such as Euclid and the James Webb Space Telescope collect unprecedented volumes of information, scientists will need advanced methods to sift through it all. AI-driven techniques, like Harvey’s Inception model, could become essential tools for interpreting the data, helping researchers zero in on the hidden clues about dark matter’s true nature.
As astronomers gather more data, AI could dramatically shape how we study dark matter. The ability of deep-learning models to recognize patterns within complex, noisy datasets will enable scientists to explore this invisible force in greater detail. If successful, this approach could finally answer some of the long-standing questions about dark matter and its role in the universe.
While dark matter may remain unseen, innovations like these bring us closer than ever to understanding what it is and how it interacts with the universe. The AI-powered leap forward offers a powerful tool for astronomers who continue to unravel the mysteries of the cosmos. The true nature of dark matter may be elusive now, but thanks to advances like Harvey's, science is inching closer to a breakthrough that could change our understanding of the universe forever.
Note: Materials provided above by The Brighter Side of News. Content may be edited for style and length.
Like these kind of feel good stories? Get The Brighter Side of News' newsletter.
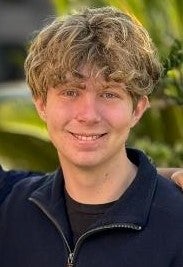