Breakthrough technology looks to revolutionize how we grow food
RhizoNet, uses AI to transform how we study plant roots, offering new insights into root behavior under various environmental conditions
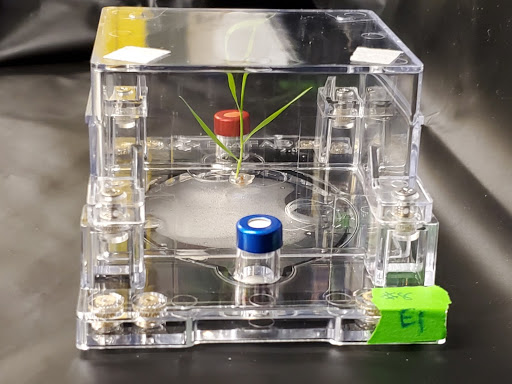
In a world striving for sustainability, understanding plant roots is essential. Roots are not just an anchor; they are a dynamic interface between the plant and soil, critical for water uptake, nutrient absorption, and, ultimately, the survival of the plant.
To boost agricultural yields and develop climate-resilient crops, scientists from Lawrence Berkeley National Laboratory (Berkeley Lab) have made a significant breakthrough. Their latest innovation, RhizoNet, uses artificial intelligence (AI) to transform how we study plant roots, offering new insights into root behavior under various environmental conditions.
Transforming Root Analysis with AI
Detailed in a study published in Scientific Reports, RhizoNet revolutionizes root image analysis by automating the process with exceptional accuracy. Traditional methods are labor-intensive and prone to errors, especially with complex root systems. It utilizes a state-of-the-art deep learning approach, enables precise tracking of root growth and biomass. It also uses an advanced convolutional neural network to segment plant roots, significantly enhancing how laboratories analyze root systems and advancing the goal of self-driving labs.
“The capability of RhizoNet to standardize root segmentation and phenotyping represents a substantial advancement in the systematic and accelerated analysis of thousands of images,” said Daniela Ushizima, lead investigator of the AI-driven software. “This innovation is instrumental in our ongoing efforts to enhance precision in capturing root growth dynamics under diverse plant conditions.”
Overcoming Traditional Challenges
Root analysis traditionally relies on flatbed scanners and manual segmentation, which are time-consuming and error-prone. Natural phenomena like bubbles, droplets, reflections, and shadows complicate automated analysis. These challenges are particularly acute at smaller spatial scales, where fine structures can be as narrow as a pixel, making manual annotation extremely challenging.
The latest version of EcoFAB, a novel hydroponic device introduced by Berkeley Lab’s Environmental Genomics and Systems Biology (EGSB) Division, addresses these challenges. EcoFAB facilitates in-situ plant imaging, offering a detailed view of root systems.
Related Stories
Developed in collaboration with the DOE Joint Genome Institute (JGI) and the Climate & Ecosystem Sciences division at Berkeley Lab, EcoFAB enhances data reproducibility in fabricated ecosystem experiments.
RhizoNet processes color scans of plants grown in EcoFABs under specific nutritional treatments. It employs a sophisticated Residual U-Net architecture to deliver root segmentation tailored for EcoFAB conditions, significantly improving prediction accuracy. The system integrates a convexification procedure that encapsulates identified roots from time series, aiding in the accurate monitoring of root biomass and growth over time.
Practical Applications and Future Potential
The study demonstrates how researchers used EcoFAB and RhizoNet to process root scans of Brachypodium distachyon, a small grass species, under different nutrient deprivation conditions over five weeks. These images, taken every three to seven days, provide vital data that help scientists understand how roots adapt to varying environments. The high-throughput nature of EcoBOT, the new image acquisition system for EcoFABs, allows for systematic experimental monitoring.
“We’ve made a lot of progress in reducing the manual work involved in plant cultivation experiments with EcoBOT, and now RhizoNet is reducing the manual work involved in analyzing the data generated,” said Peter Andeer, a research scientist in EGSB. “This increases our throughput and moves us toward the goal of self-driving labs.”
During model tuning, the findings indicated that using smaller image patches significantly enhances performance. These patches allow the model to capture fine details more effectively, enriching the latent space with diverse feature vectors. This approach not only improves the model's ability to generalize to unseen EcoFAB images but also increases its robustness, enabling it to focus on thin objects and capture intricate patterns despite various visual artifacts.
Smaller patches also help prevent class imbalance by excluding sparsely labeled patches, predominantly background. The team’s results show high accuracy, precision, recall, and Intersection over Union (IoU) for smaller patch sizes, demonstrating the model's improved ability to distinguish roots from other objects or artifacts.
The study validates RhizoNet's performance by comparing predicted root biomass to actual measurements. Linear regression analysis revealed a significant correlation, underscoring the precision of automated segmentation over manual annotations. This comparison highlights the challenge human annotators face and showcases the advanced capabilities of RhizoNet, particularly when trained on smaller patch sizes.
This research lays the groundwork for future innovations in sustainable energy solutions and carbon-sequestration technology using plants and microbes. “Our next steps involve refining RhizoNet’s capabilities to further improve the detection and branching patterns of plant roots,” said Ushizima.
"We also see potential in adapting and applying these deep-learning algorithms for roots in soil as well as new materials science investigations. We're exploring iterative training protocols, hyperparameter optimization, and leveraging multiple GPUs. These computational tools are designed to assist science teams in analyzing diverse experiments captured as images, and have applicability in multiple areas,” he continued.
RhizoNet represents a significant leap forward in our ability to study and understand plant roots, paving the way for advancements in agriculture and sustainability.
Note: Materials provided above by The Brighter Side of News. Content may be edited for style and length.
Like these kind of feel good stories? Get the Brighter Side of News' newsletter.
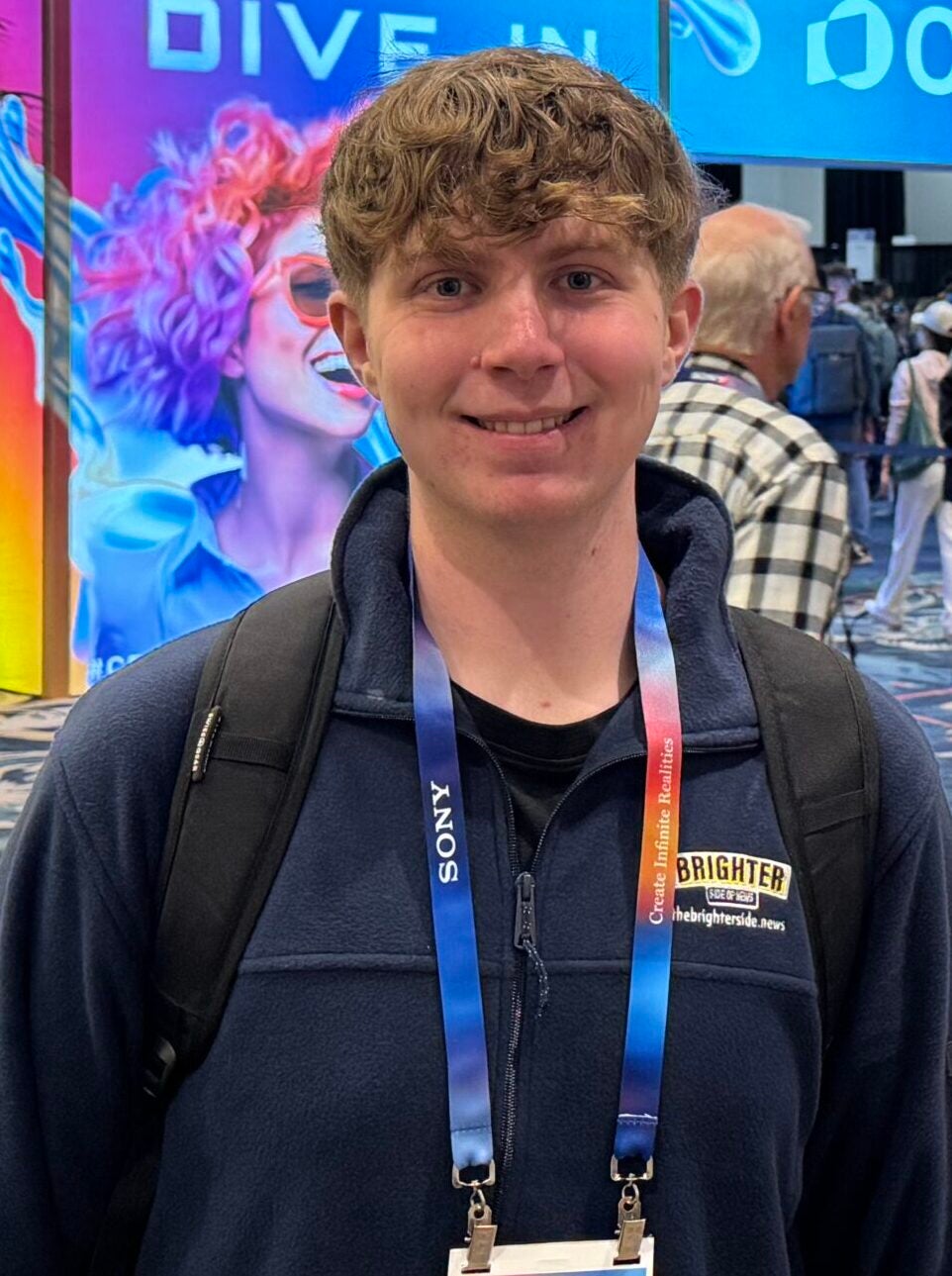
Joshua Shavit
Science & Technology Writer | AI and Robotics Reporter
Joshua Shavit is a Los Angeles-based science and technology writer with a passion for exploring the breakthroughs shaping the future. As a contributor to The Brighter Side of News, he focuses on positive and transformative advancements in AI, technology, physics, engineering, robotics and space science. Joshua is currently working towards a Bachelor of Science in Business Administration at the University of California, Berkeley. He combines his academic background with a talent for storytelling, making complex scientific discoveries engaging and accessible. His work highlights the innovators behind the ideas, bringing readers closer to the people driving progress.