AI tackles one of the most difficult challenges in quantum chemistry
New AI research shows how neural networks can model excited molecular states, advancing sustainable tech like solar cells and photocatalysts.
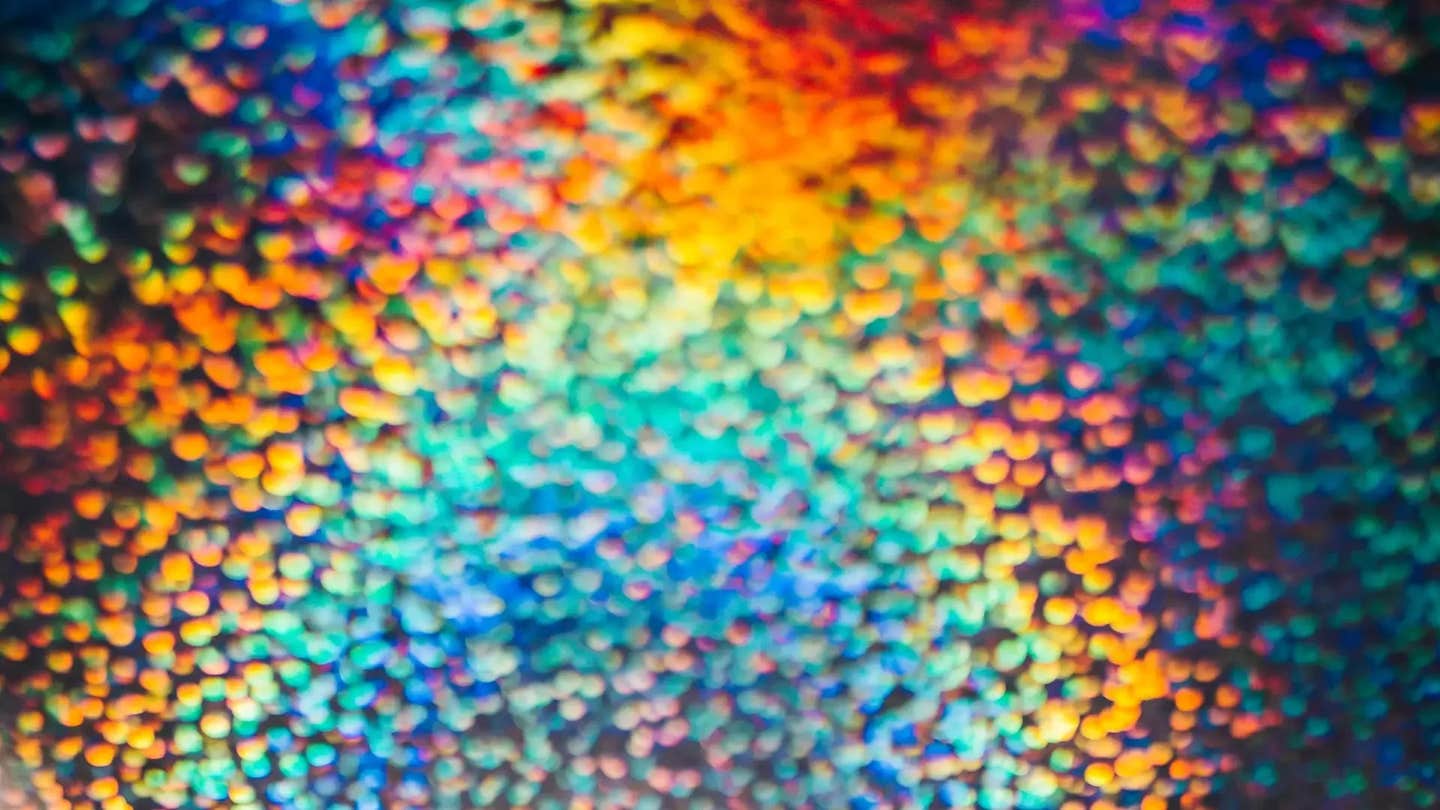
FermiNet is the first deep learning applications that could compute the energy of atoms and molecules from fundamental principles with an accuracy sufficient for practical use. (CREDIT: Imperial College London.)
New research in artificial intelligence (AI) has introduced a promising method for modeling complex molecular systems, potentially revolutionizing material science.
The study, a collaboration between Imperial College London and Google DeepMind, outlines how neural networks—AI models inspired by the structure of the human brain—can offer a novel approach to understanding the behavior of excited molecules.
Published in the journal Science, this research could significantly impact future innovations in material design and chemical synthesis.
The study’s focus lies in modeling the quantum behavior of molecules in "excited states." An excited state occurs when a molecule or material absorbs a significant amount of energy, causing its electrons to move into a temporary, more energized configuration.
This phenomenon can be triggered by exposure to light or high temperatures, creating a unique energetic fingerprint that is characteristic of each molecule. Understanding these energy transitions is crucial for technologies like solar panels, light-emitting diodes (LEDs), semiconductors, and photocatalysts. Moreover, these processes are fundamental in natural phenomena such as photosynthesis and human vision.
Despite their importance, the complexity of excited states has made them challenging to model accurately. The challenge stems from the quantum nature of electrons. Their positions within a molecule can't be pinpointed; instead, they can only be described in terms of probabilities. This uncertainty makes it exceedingly difficult to predict how molecules will behave when they absorb and release energy.
Dr. David Pfau, the lead researcher from Google DeepMind and the Department of Physics at Imperial College London, highlighted the difficulty of capturing quantum systems. "Representing the state of a quantum system is extremely challenging," he explained.
Related Stories
"A probability has to be assigned to every possible configuration of electron positions. The space of all possible configurations is enormous—if you tried to represent it as a grid with 100 points along each dimension, then the number of possible electron configurations for the silicon atom would be larger than the number of atoms in the universe. This is exactly where we thought deep neural networks could help," he continued.
The neural network the researchers used is called FermiNet, short for Fermionic Neural Network. FermiNet stands out as a key tool because it was one of the first deep learning applications that could compute the energy of atoms and molecules from fundamental principles with an accuracy sufficient for practical use. The approach they developed uses a new mathematical framework combined with this AI model, offering a fresh way to solve the fundamental equations that describe the states of molecules.
The results were promising, especially in handling challenging molecular systems. For example, the team tested their approach on carbon dimer, a small but complex molecule. They achieved a mean absolute error (MAE) of just 4 millielectronvolts (meV)—an impressively small energy unit.
This result was five times more precise compared to previous gold-standard methods, which had an error of 20 meV. This means the new method brings the predictions much closer to the experimental results, enhancing the reliability of simulations involving excited states.
Moreover, the researchers tested their neural network approach on computationally difficult scenarios, where two electrons were excited simultaneously. The model's results were within about 0.1 electronvolts (eV) of the most complex calculations available today. This level of accuracy in predicting the energy states of molecules represents a substantial advancement in computational chemistry.
According to Dr. Pfau, the research is about pushing boundaries and inspiring the scientific community to further explore these fundamental phenomena. "We tested our method on some of the most challenging systems in computational chemistry, where two electrons are excited simultaneously, and found we were within around 0.1 eV of the most demanding, complex calculations done to date," Pfau noted. "Today, we’re making our latest work open source, and hope the research community will build upon our methods to explore the unexpected ways matter interacts with light."
Making the work open source is particularly significant. By sharing these methods publicly, the team hopes to encourage collaboration and innovation across the global scientific community. This open approach will enable other researchers to use these powerful computational tools, potentially discovering new interactions and phenomena that could have wide-reaching implications. For example, it could lead to new ways to prototype materials and chemicals using computer simulations before attempting to synthesize them in the lab, saving both time and resources.
The implications of this research extend to the development of sustainable energy solutions, efficient lighting technologies, and even novel medical applications where understanding molecular interactions is key. Technologies like solar cells and photocatalysts depend heavily on understanding how molecules behave when excited by light, and this method offers a more precise way to predict those behaviors.
By leveraging AI to tackle one of the most difficult problems in physical chemistry, the research sets the stage for more effective simulations in material science and beyond. Instead of relying purely on experimental methods—which can be time-consuming and costly—scientists now have a more accurate computational tool that brings theoretical models closer to real-world scenarios.
As research continues, the integration of deep learning into chemistry could pave the way for accelerated discoveries and technological advancements that benefit a variety of industries.
Note: Materials provided above by The Brighter Side of News. Content may be edited for style and length.
Like these kind of feel good stories? Get The Brighter Side of News' newsletter.
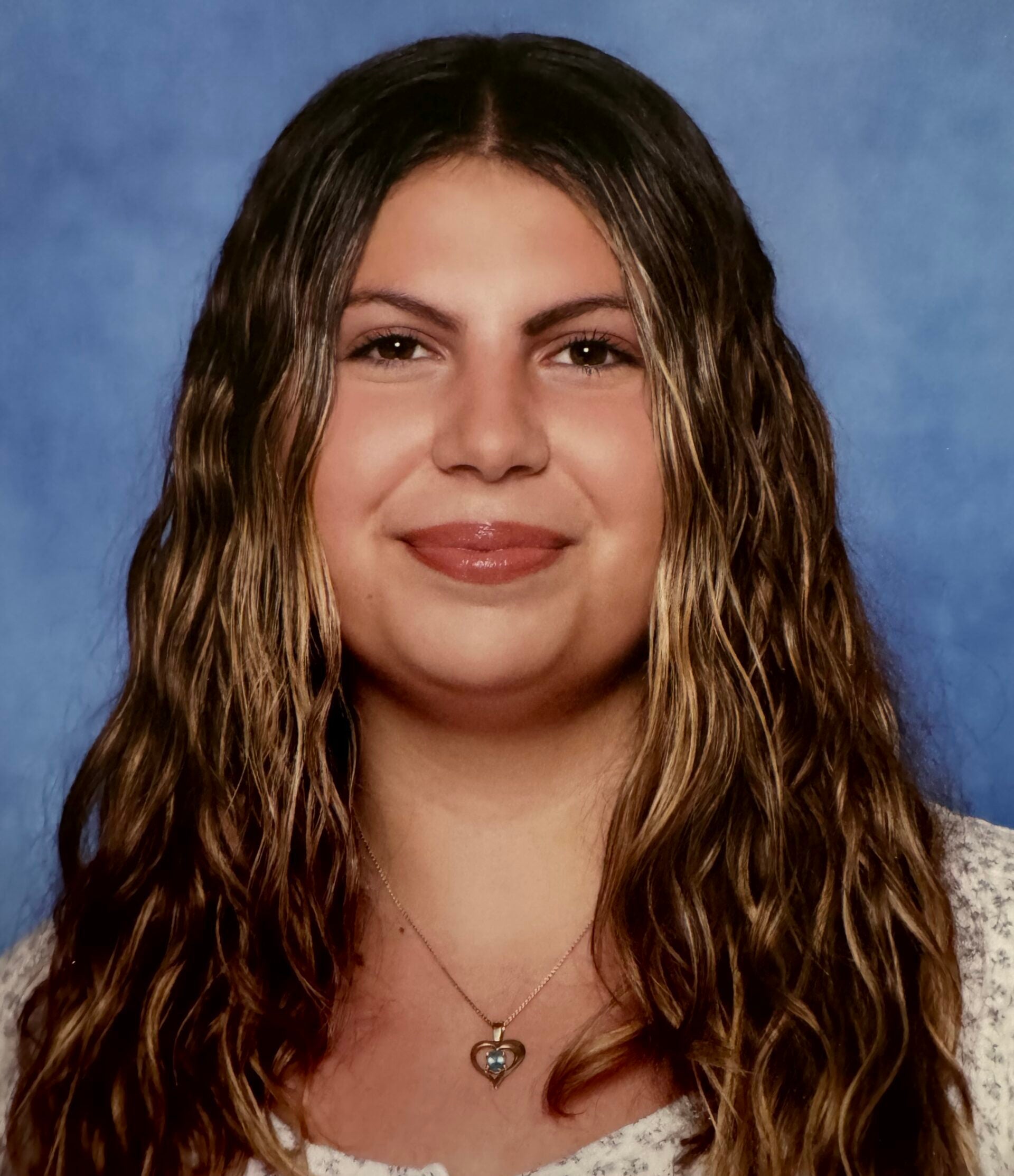
Rebecca Shavit
Science & Technology Journalist | Innovation Storyteller
Based in Los Angeles, Rebecca Shavit is a dedicated science and technology journalist who writes for The Brighter Side of News, an online publication committed to highlighting positive and transformative stories from around the world. With a passion for uncovering groundbreaking discoveries and innovations, she brings to light the scientific advancements shaping a better future. Her reporting spans a wide range of topics, from cutting-edge medical breakthroughs and artificial intelligence to green technology and space exploration. With a keen ability to translate complex concepts into engaging and accessible stories, she makes science and innovation relatable to a broad audience.