AI powers major leap in understanding Alzheimer’s and Parkinson’s Diseases
Researchers at Rice University and the Changping Laboratory have developed a new artificial intelligence tool called RibbonFold.
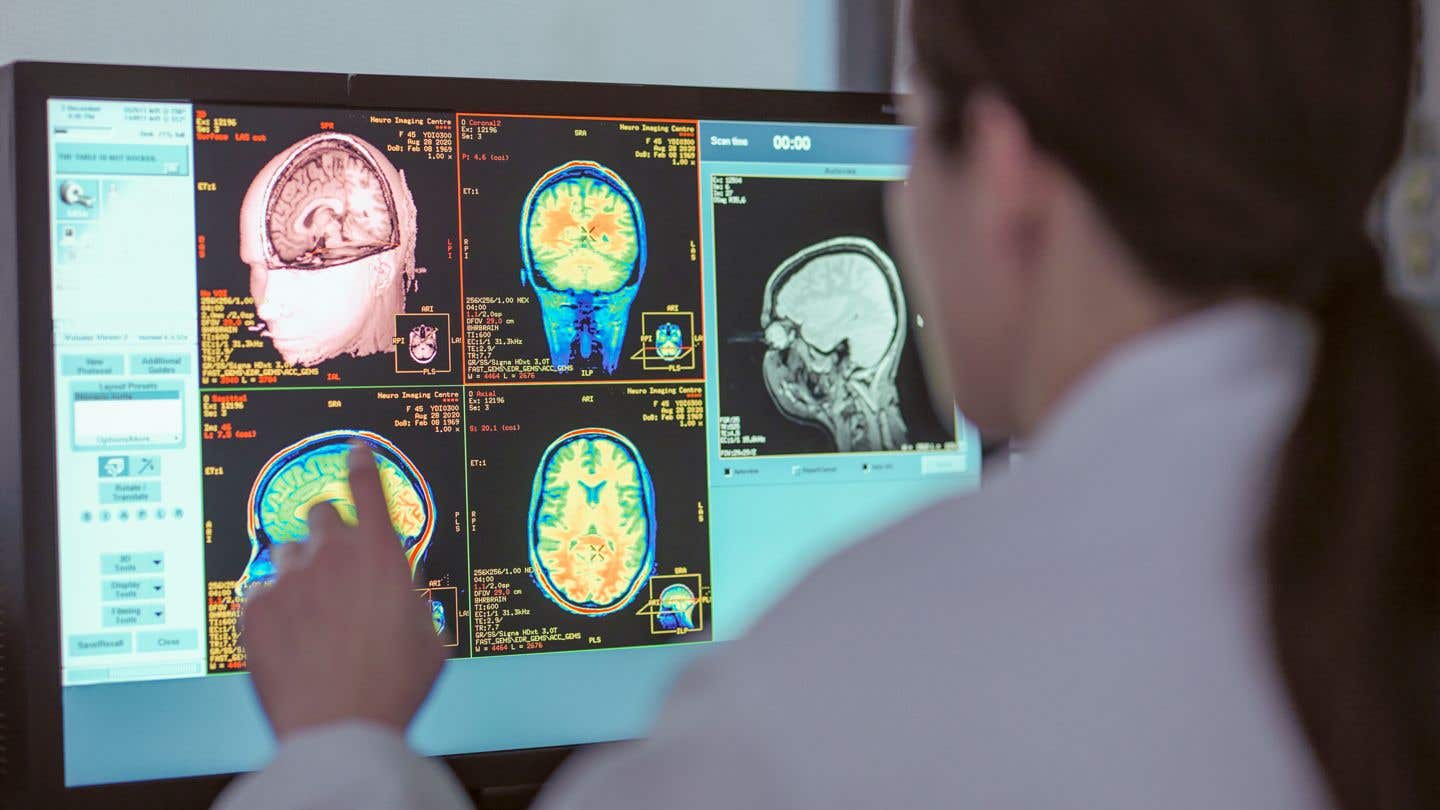
New AI tool predicts toxic protein shapes tied to Alzheimer’s and Parkinson’s, opening doors for targeted treatments. (CREDIT: Getty Images)
In the fight against diseases like Alzheimer’s and Parkinson’s, scientists have long struggled to predict how certain proteins misfold. These misfolded proteins, known as amyloids, clump together in the brain, forming long fibers that interfere with normal brain function. Despite major advances in protein structure prediction, the unique and variable shapes of amyloids have remained elusive—until now.
Researchers at Rice University and the Changping Laboratory have developed a new artificial intelligence tool called RibbonFold. This breakthrough method can predict the structure of amyloid fibrils with far more accuracy than existing tools, offering a clearer picture of how these misfolded proteins evolve and lead to disease. Led by Mingchen Chen and Peter Wolynes, the study was published in Proceedings of the National Academy of Sciences.
Folding patterns and a deeper mystery
Proteins are usually designed by nature to fold into a specific shape to carry out their functions. For most proteins, these shapes are well-defined and can be predicted using tools like AlphaFold2 and AlphaFold3. These tools rely on known evolutionary patterns and the physical forces that govern how a protein chain twists and folds. But amyloids don’t follow those same rules.
Instead of folding into a single functional shape, amyloids can form many different structures. All of them share a core feature—long, beta-sheet structures held together by hydrogen bonds—but their outer shapes vary depending on conditions like pH, temperature, and even small changes in sequence. This variability, called polymorphism, has made amyloids hard to predict and study.
Wolynes, co-director of Rice’s Center for Theoretical Biological Physics, explains the problem. “Misfolded proteins can take on many different structures,” he said. “Our method shows that stable polymorphs will likely win out over time by being more insoluble than other forms, explaining the late onset of symptoms.”
Why amyloids matter
Amyloids are best known for their role in devastating brain diseases. Prion diseases like Kuru and Scrapie, which first brought amyloids to scientists' attention, are now part of a much larger group of disorders tied to protein misfolding. Alzheimer’s, Parkinson’s, and even Type II diabetes involve harmful protein aggregates.
Related Stories
What makes these fibers so dangerous is their ability to grow. They start small—just a few misfolded proteins clumped together—and gradually recruit more proteins to join the chain. Over time, they form tough, insoluble structures that interfere with cell function. The process can take years, which is why many neurodegenerative diseases develop slowly.
Amyloids aren’t always harmful, though. Some play a role in memory formation or perform useful tasks in yeast cells. Researchers have even shown they can act as weak catalysts, though they’re still not as efficient as enzymes. Still, when things go wrong, amyloids can destroy tissue and cause lasting damage.
Understanding how amyloids form—and predicting their shapes—has been one of biology’s biggest challenges. The number of experimentally known amyloid structures remains small, especially compared to the more than 200,000 well-folded proteins listed in global structure databases.
How RibbonFold changes the game
RibbonFold takes a new approach to solving this mystery. While AlphaFold2 was designed to predict the neat, functional folds of single protein chains, RibbonFold focuses on the stacked, ribbon-like structures formed by amyloid fibrils.
The team modified AlphaFold2’s framework to account for these stacking patterns and trained their model using known amyloid structures. They also introduced a special loss function to reflect the polymorphic nature of amyloids, allowing the tool to better capture their complex behavior.
“RibbonFold outperforms other AI-based prediction tools like AlphaFold, which were trained only to predict correctly folded globular protein structures,” said Wolynes. In tests, RibbonFold produced more accurate results and predicted previously unknown fibril shapes that were later confirmed using experimental data.
The model scored an average TM-score of 0.5 on independent test sets—well above the results seen with previous tools. Even more important, it could map the full range of polymorphs for key disease-linked sequences, revealing how certain structures evolve to become more stable and less soluble over time. These more insoluble forms likely play a key role in disease progression.
The physics behind the fold
Evolution has shaped many proteins to fold quickly and reliably into useful shapes. But not all proteins follow the same rules. Some remain disordered or flexible, adopting many shapes depending on their environment. Others can stick together in ways that don’t reflect their original design.
One common way this happens is through domain swapping, where parts of proteins interact with each other to form larger structures. This process still follows the original folding patterns. But amyloids break away from that logic. Their folding isn’t guided by evolution but rather by basic physical forces that favor beta-sheet stacking.
At high enough concentrations, almost any protein or peptide can form aggregates. But not all of them turn into amyloids. Coarse-grained energy analysis shows that only about half of all protein sequences have segments capable of forming strong, amyloid-like associations.
As they grow, these fibers may also “backtrack,” pulling parts of themselves apart to keep adding new proteins. This behavior reveals a rugged energy landscape, filled with peaks and valleys that slow down the folding process. These same challenges make direct molecular simulations almost impossible, which is why machine learning methods like RibbonFold are so valuable.
A two-dimensional perspective
The team behind RibbonFold drew on earlier work that suggested amyloid growth can be viewed as a two-dimensional folding problem. Rather than folding into complex three-dimensional shapes like globular proteins, amyloids form ribbons, where the folding happens mostly in a plane. These ribbons are made up of repeating stacks of peptides aligned in the same direction.
By limiting the folding to this ribbon-like structure, the researchers reduced the complexity of the problem. It also allowed them to apply the same physical principles that guide normal protein folding, but in a simplified two-dimensional framework. Previous versions of this approach using molecular dynamics had already shown promise in predicting amyloid shapes, particularly for the Aβ protein linked to Alzheimer’s.
Now, with AI taking over the prediction process, the method is faster, more accurate, and can scale to many different sequences.
What comes next
RibbonFold’s ability to predict the structure of harmful protein aggregates could revolutionize how drugs are developed for neurodegenerative diseases. Instead of targeting general protein behavior, drugmakers could design molecules that bind to specific polymorphs—the exact structures that cause the most damage.
“This work not only explains a long-standing problem but also equips us with the tools to systematically study and intervene in one of life’s most destructive processes,” said Chen, co-corresponding author of the study.
The insights from RibbonFold may also inform the design of new biomaterials. Understanding how proteins self-assemble could lead to new synthetic structures, inspired by the way nature builds strong and stable fibrils. The tool might even help scientists explore how harmless proteins suddenly become dangerous over time—a question that has puzzled researchers for decades.
Most important of all, RibbonFold brings us closer to solving one of medicine’s greatest mysteries. If scientists can predict which fibril forms are likely to appear, and when, they may be able to catch diseases before symptoms begin.
The project received support from the National Science Foundation, the Welch Foundation, and the Changping Laboratory. Co-first authors of the paper included Liangyue Guo and Qilin Yu, along with Di Wang and Xiaoyu Wu.
As Wolynes noted, “The ability to predict amyloid polymorphs efficiently may guide future breakthroughs in preventing harmful protein aggregation, a crucial step toward tackling some of the world’s most pressing neurodegenerative challenges.”
Note: The article above provided above by The Brighter Side of News.
Like these kind of feel good stories? Get The Brighter Side of News' newsletter.
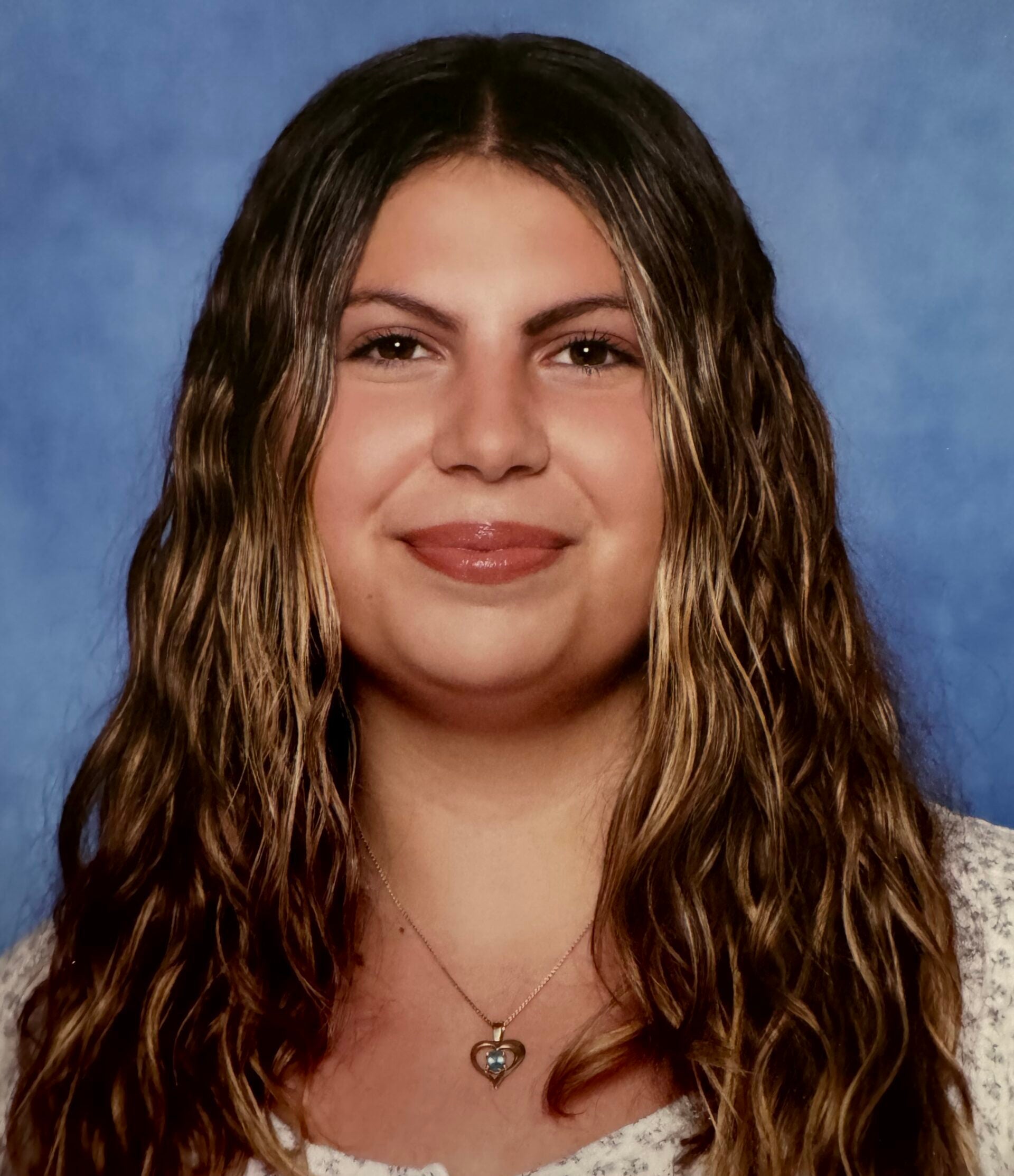
Rebecca Shavit
Science & Technology Journalist | Innovation Storyteller
Based in Los Angeles, Rebecca Shavit is a dedicated science and technology journalist who writes for The Brighter Side of News, an online publication committed to highlighting positive and transformative stories from around the world. With a passion for uncovering groundbreaking discoveries and innovations, she brings to light the scientific advancements shaping a better future. Her reporting spans a wide range of topics, from cutting-edge medical breakthroughs and artificial intelligence to green technology and space exploration. With a keen ability to translate complex concepts into engaging and accessible stories, she makes science and innovation relatable to a broad audience.