AI-powered test boasts an impressive 93% accuracy rate at diagnosing ovarian cancer
This test boasts an impressive 93 percent accuracy rate, a significant leap forward in the quest to detect ovarian cancer
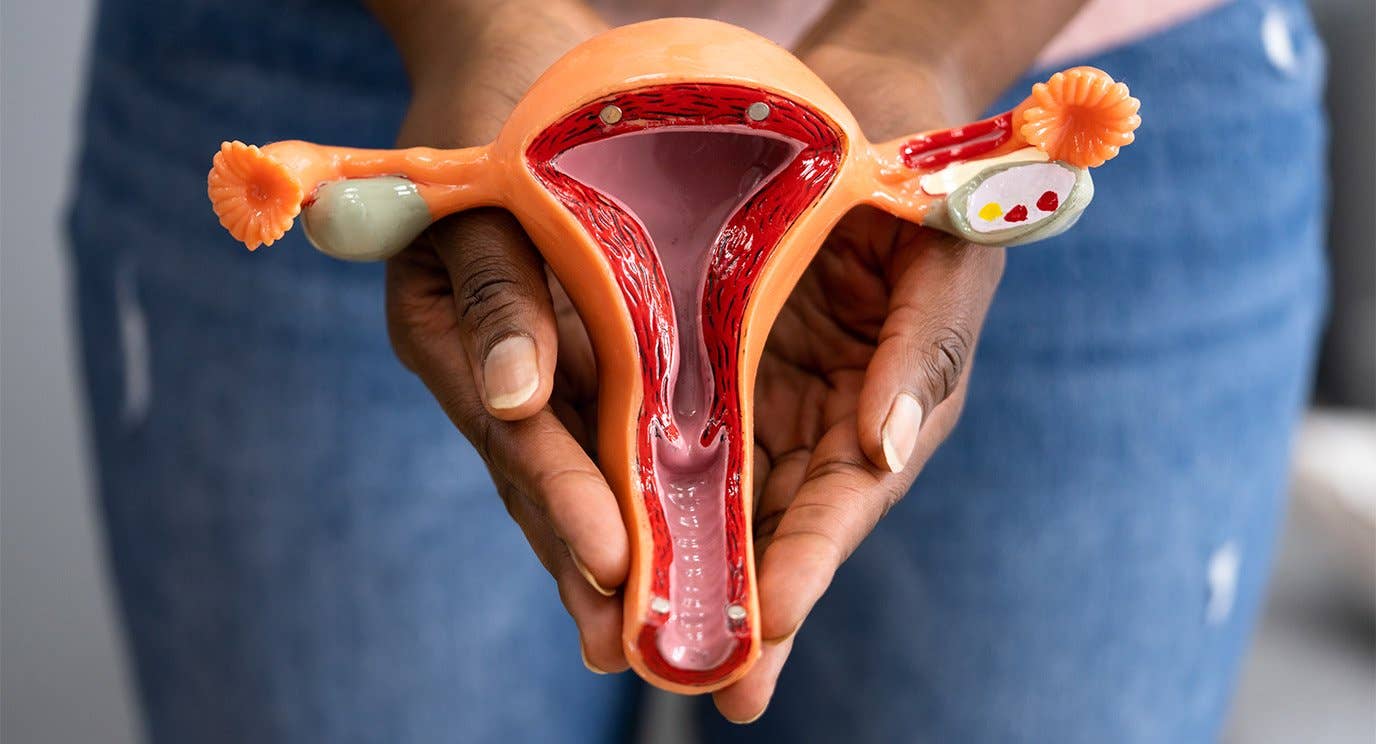
This test boasts an impressive 93 percent accuracy rate, a significant leap forward in the quest to detect ovarian cancer. (CREDIT: Creative Commons)
For decades, the medical community has been striving to develop an accurate early diagnostic test for ovarian cancer, a disease often dubbed the "silent killer" due to its asymptomatic nature in the early stages.
However, a breakthrough has finally emerged from the Georgia Tech Integrated Cancer Research Center (ICRC), where scientists have harnessed the power of machine learning and blood metabolites to create a groundbreaking diagnostic tool. This test boasts an impressive 93 percent accuracy rate, a significant leap forward in the quest to detect ovarian cancer at its earliest and most treatable stages.
The driving force behind this innovative diagnostic test is Professor John McDonald, a stalwart in the field of biological sciences and the founding director of ICRC. He spearheaded the study and serves as its corresponding author. McDonald explains that their new test outperforms existing methods, particularly in detecting early-stage ovarian cancer among women who are clinically classified as normal.
The team's findings and methodologies are comprehensively detailed in their recently published paper, "A Personalized Probabilistic Approach to Ovarian Cancer Diagnostics," which graced the pages of the prestigious medical journal, Gynecologic Oncology.
Related Stories
McDonald and his colleagues have crafted a more clinically relevant approach to ovarian cancer diagnosis, one that takes into account a patient's individual metabolic profile, providing a more accurate probability of the disease's presence or absence.
In McDonald's own words, "This personalized, probabilistic approach to cancer diagnostics is more clinically informative and accurate than traditional binary (yes/no) tests. It represents a promising new direction in the early detection of ovarian cancer, and perhaps other cancers as well."
The study's co-authors are an impressive lineup of scientists and physicians, including Dongjo Ban, a Bioinformatics Ph.D. student, Research Scientists Stephen N. Housley, Lilya V. Matyunina, and L.DeEtte (Walker) McDonald, as well as Regents' Professor Jeffrey Skolnick, a renowned figure in computational systems biology.
Micrograph of a mucinous ovarian tumor. (CREDIT: NIH)
Two collaborating physicians, University of North Carolina Professor Victoria L. Bae-Jump and Ovarian Cancer Institute of Atlanta Founder and CEO Benedict B. Benigno, have also lent their expertise to this groundbreaking research. Notably, the team is taking steps to commercialize their technology, with plans to undergo requisite trials and seek FDA approval for the test.
Ovarian cancer, often referred to as the "silent killer," poses a formidable challenge to the medical community because it typically remains asymptomatic until it reaches advanced stages, making treatment significantly more difficult. McDonald underscores the critical need for an accurate early diagnostic test, given that the five-year survival rate for late-stage ovarian cancer patients, even after treatment, hovers around a dismal 31 percent. In contrast, when ovarian cancer is detected and treated early, the average five-year survival rate soars to over 90 percent.
The frequency and Gini importance values of features in each dataset. The x-axis and y-axis correspond to feature frequency and importance value, respectively. (CREDIT: Gynecologic Oncology)
The elusiveness of early, accurate diagnostic tests for ovarian cancer can be attributed to the intricate molecular nature of cancer itself. According to McDonald, there are numerous molecular pathways that can lead to the development of ovarian cancer. The high degree of molecular heterogeneity among patients makes it impossible to identify a universal diagnostic biomarker for the disease. Consequently, the research team turned to artificial intelligence, specifically machine learning, to devise an alternative approach.
Co-author Dongjo Ban, whose thesis research played a significant role in the study, explains their rationale. They decided to use metabolic profiles as the foundation of their analysis because changes in these profiles reflect underlying molecular alterations across multiple levels.
A woman’s risk of getting ovarian cancer during her lifetime is about 1 in 78, according to the American Cancer Society. This cancer mainly develops in older women. (CREDIT: St. John's Cancer Institute)
As Jeffrey Skolnick puts it, "The set of human metabolites is a collective measure of the health of cells," and their approach allowed artificial intelligence to identify the key players in an individual's metabolic profile without preselecting specific metabolites.
Mass spectrometry, a powerful analytical tool, identifies the presence of metabolites in the blood by detecting their mass and charge signatures. Ban highlights that while mass spectrometry can detect the presence of metabolites, it cannot provide precise chemical compositions for the majority of metabolites in human blood.
Comparison of consensus classifier performance. A) The performance characteristics of the models were graphically represented through precision-recall (PR) curves. Besides the HP dataset, the models for the remaining datasets showed similar levels of performance. (CREDIT: Gynecologic Oncology)
Nevertheless, the team realized that the mere detection of different metabolites in individuals' blood, even without knowing their exact chemical makeup, could be incorporated into machine learning-based predictive models.
Ban explains, "Thousands of metabolites are known to be circulating in the human bloodstream, and they can be readily and accurately detected by mass spectrometry and combined with machine learning to establish an accurate ovarian cancer diagnostic."
The researchers crafted their groundbreaking approach by merging metabolomic profiles with machine learning-based classifiers, ultimately achieving a remarkable 93 percent accuracy rate when tested on a cohort of 564 women from various regions. Out of these women, 431 had active ovarian cancer, while the remaining 133 were cancer-free. The team is now conducting further studies to assess the test's ability to detect very early-stage disease in asymptomatic women.
McDonald envisions a future where individuals with metabolic profiles indicating a low likelihood of cancer would only require yearly monitoring. Conversely, those with metabolic profiles falling within a range where a majority have previously been diagnosed with ovarian cancer may undergo more frequent monitoring or immediate referral for advanced screening.
The development of this personalized, probabilistic diagnostic test marks a pivotal moment in the fight against ovarian cancer. As researchers continue to refine and validate their approach, there is newfound hope that this silent killer may no longer claim so many lives in the shadows.
Note: Materials provided above by The Brighter Side of News. Content may be edited for style and length.
Like these kind of feel good stories? Get the Brighter Side of News' newsletter.
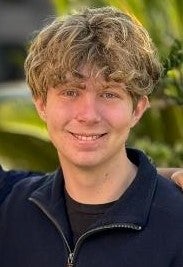