AI outperforms experts in diagnosing ovarian cancer, study finds
AI models outperform experts in diagnosing ovarian cancer, enhancing accuracy and reducing healthcare burdens in a groundbreaking international study.
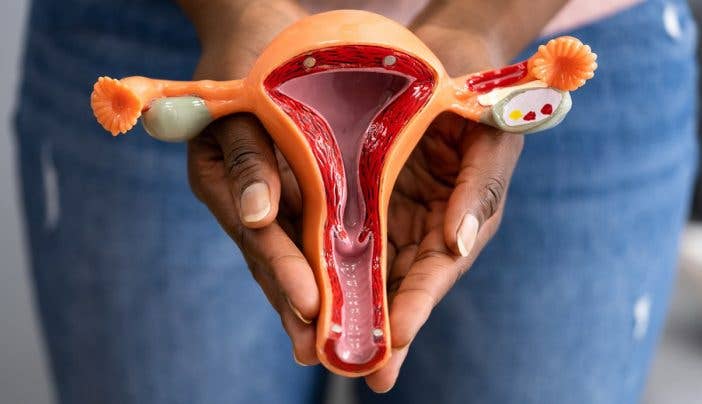
Discover how AI models surpass human experts in ovarian cancer diagnosis, improving accuracy and streamlining healthcare workflows in a global study. (CREDIT: CC BY-SA 4.0)
Ovarian tumors, often detected incidentally, pose a diagnostic challenge that requires careful management. Decisions depend on assessing the malignancy risk and addressing patient symptoms. For benign cases, conservative monitoring or minimally invasive surgery preserves fertility and reduces costs. However, suspected malignancies demand referral to specialists, as gynecologic oncologists significantly improve survival rates.
Transvaginal ultrasound remains the primary diagnostic tool, offering high accuracy in skilled hands. Unfortunately, many healthcare systems lack sufficient expert ultrasound examiners, even in high-income countries. This shortage leads to delayed diagnoses and unnecessary treatments, placing a significant burden on healthcare systems.
In some regions, patients face prolonged wait times for specialist consultations, further exacerbating the problem. Misdiagnoses not only delay necessary treatments but also increase patient anxiety and healthcare costs.
Artificial intelligence (AI) has emerged as a promising solution. Neural networks, particularly convolutional neural networks (CNNs), have demonstrated potential in distinguishing benign from malignant ovarian lesions. However, AI models often face challenges adapting to diverse clinical settings due to "domain shift," where new data differs from training datasets.
This variability can limit AI performance in real-world applications. For example, differences in imaging protocols, patient demographics, and equipment can significantly impact diagnostic accuracy.
A recent international study sought to address these limitations. Researchers from Karolinska Institutet and collaborators conducted a multicenter analysis, known as the Ovarian Tumor Machine Learning Collaboration - Retrospective Study (OMLC-RS).
Using a dataset of 17,119 ultrasound images from 3,652 patients across 20 centers in eight countries, the study evaluated transformer-based AI models. These models, a state-of-the-art alternative to CNNs, were trained to generalize across diverse clinical environments.
To test AI's diagnostic potential, researchers implemented a leave-one-center-out validation strategy. This approach trained models on data from 19 centers while reserving the 20th for testing. This rigorous method ensured the AI could adapt to unseen data from new centers. The study also included comparisons with human examiners, encompassing 33 experts and 33 less experienced practitioners.
The results were striking. The AI models achieved an F1 score—a metric balancing precision and recall—of 83.5%, surpassing experts at 79.5% and non-experts at 74.1%. Accuracy rates also highlighted AI's superiority, with models reaching 86.3% compared to 82.6% for experts and 77.7% for non-experts. These metrics underscore AI's potential to support and enhance diagnostic accuracy, particularly in challenging cases.
Related Stories
AI's advantages extended beyond accuracy. The models demonstrated reduced false negative and false positive rates compared to human examiners. For example, the AI's false negative rate was 15.12%, significantly lower than the 17.6% observed among experts. Similarly, the AI achieved a false positive rate of 12.7%, outperforming both experts and non-experts. These improvements could lead to earlier cancer detection and fewer unnecessary interventions.
The study, published in Nature Medicine, also highlighted AI’s ability to handle variability in clinical data. By training on diverse datasets, the models learned to adapt to different patient populations, imaging devices, and protocols. This robustness is crucial for real-world applications, where standardization across centers is often lacking.
One of AI's most compelling benefits lies in streamlining healthcare workflows. In a simulated triage scenario, AI assistance reduced the need for expert referrals by 63%. This efficiency could alleviate the burden on overextended specialists, enabling them to focus on complex cases. Additionally, the AI reduced diagnostic errors by 18%, enhancing patient care and outcomes.
Professor Elisabeth Epstein of Karolinska Institutet emphasized the significance of these findings. "Ovarian tumors are often detected by chance, and there’s a serious shortage of ultrasound experts worldwide," she noted. "AI can complement human expertise, especially in settings with limited resources."
The study’s simulation further demonstrated how AI could streamline patient management. By identifying cases that required immediate expert intervention, the AI ensured that critical resources were allocated effectively. This approach not only improved diagnostic accuracy but also reduced patient wait times and associated healthcare costs.
Despite these promising results, researchers acknowledged the need for further validation. Prospective clinical studies are underway to assess AI's safety and effectiveness in routine practice. Future research will include randomized multicenter trials to evaluate the impact on patient management and healthcare costs. These trials aim to provide comprehensive data on how AI integration could revolutionize ovarian cancer diagnosis globally.
While AI demonstrates immense potential, its integration into clinical practice is not without hurdles. The study highlighted the importance of addressing domain shift and ensuring models generalize across diverse populations and imaging protocols. This requires comprehensive datasets representing varied clinical environments, a goal often constrained by legal and economic factors.
Additionally, the researchers stressed the need for external validation. A meta-analysis revealed that many studies overestimate AI's diagnostic accuracy due to insufficient testing on external datasets. To build trust and confidence, models must undergo rigorous evaluation in real-world scenarios.
Ethical considerations also play a crucial role in AI deployment. Ensuring patient data privacy and addressing biases in training datasets are essential for creating equitable and reliable diagnostic tools. Collaboration between clinicians, researchers, and policymakers is necessary to establish guidelines that facilitate the ethical and effective use of AI in healthcare.
Collaborative efforts played a pivotal role in the OMLC-RS study. Researchers from institutions like the KTH Royal Institute of Technology contributed to developing and validating the AI models. Funding from organizations such as the Swedish Research Council and the Swedish Cancer Society supported the research, highlighting the importance of sustained investment in AI innovation.
The success of transformer-based AI models in diagnosing ovarian cancer marks a significant step forward. By outperforming human experts and optimizing resource allocation, these tools could revolutionize cancer diagnostics. However, researchers remain cautious, emphasizing the need for continued development and adaptation.
"With further research, AI can become an integral part of healthcare," said Filip Christiansen, a doctoral student at Karolinska Institutet. "It has the potential to relieve experts and optimize hospital resources, but we must ensure it meets diverse clinical needs."
As AI continues to evolve, its role in addressing global healthcare challenges will expand. From reducing diagnostic delays to improving patient outcomes, AI-driven solutions offer a glimpse into a more efficient and equitable future.
The potential applications of AI extend beyond ovarian cancer. Similar technologies could be adapted for diagnosing other complex conditions, such as breast cancer or cardiovascular diseases. By leveraging the strengths of AI, healthcare systems can achieve greater accuracy and efficiency, ultimately enhancing patient care.
However, the journey toward widespread AI adoption requires collaboration and transparency. Ongoing education and training for clinicians will be vital to ensure they can effectively integrate AI tools into their workflows.
Additionally, fostering public trust through clear communication about AI’s capabilities and limitations will be crucial for its acceptance.
Note: Materials provided above by The Brighter Side of News. Content may be edited for style and length.
Like these kind of feel good stories? Get The Brighter Side of News' newsletter.
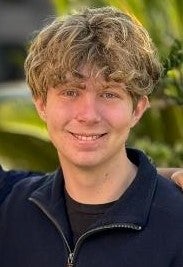