AI is substantially more energy-efficient thanks to groundbreaking new device
Researchers have demonstrated a state-of-the-art hardware device that could reduce energy consumption for artificial intelligent (AI) computing applications by a factor of at least 1,000.
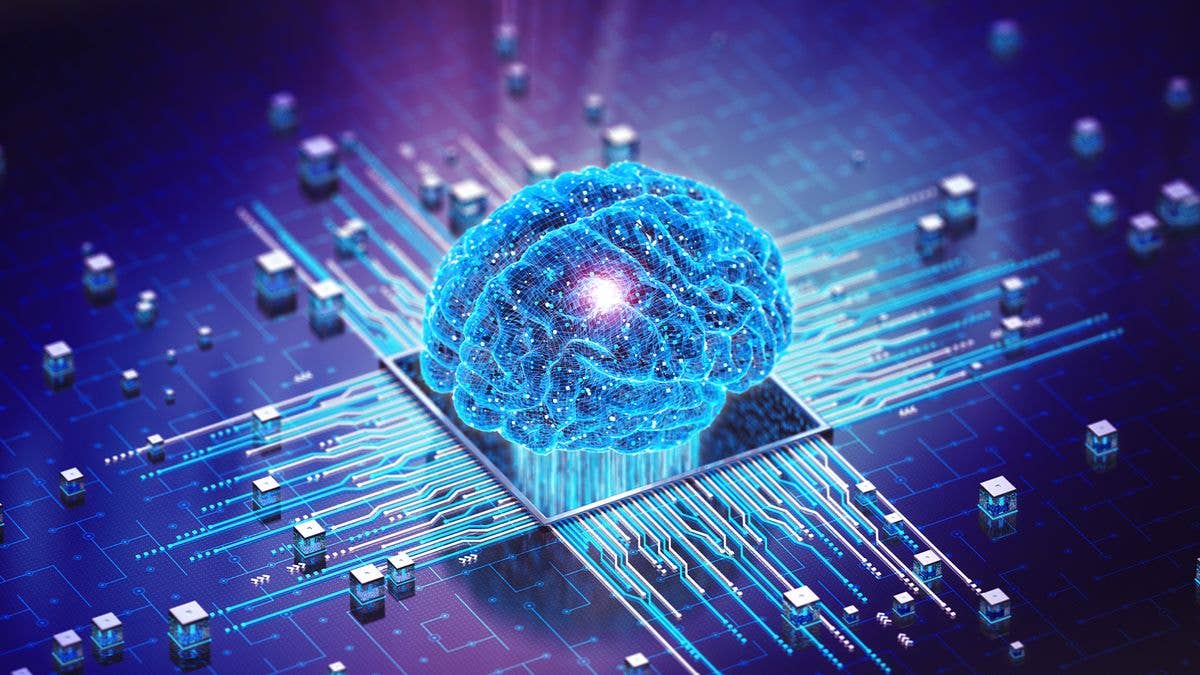
Artificial Intelligence (CREDIT: Live Science)
Researchers at the University of Minnesota Twin Cities have developed a groundbreaking hardware device that could drastically reduce energy consumption for AI computing by at least 1,000 times.
This innovation, detailed in npj Unconventional Computing, marks a significant leap forward in making AI applications more energy-efficient while maintaining high performance and low costs.
Traditional AI processes consume large amounts of energy by transferring data between logic (where information is processed) and memory (where data is stored). To address this, researchers from the University of Minnesota's College of Science and Engineering have introduced a novel model where data remains within the memory. This new approach, known as computational random-access memory (CRAM), eliminates the need for data to leave the memory array.
“This work is the first experimental demonstration of CRAM, where the data can be processed entirely within the memory array without the need to leave the grid where a computer stores information,” explained Yang Lv, a postdoctoral researcher in the Department of Electrical and Computer Engineering and first author of the paper.
The global energy demand for AI is projected to double from 460 terawatt-hours (TWh) in 2022 to 1,000 TWh in 2026, according to the International Energy Agency (IEA). This anticipated increase underscores the urgency of developing more energy-efficient AI technologies.
The CRAM-based machine learning inference accelerator proposed by the University of Minnesota team could achieve improvements up to 1,000 times. In some instances, energy savings were shown to be 2,500 and 1,700 times greater compared to traditional methods.
The journey to this breakthrough spans over two decades. “Our initial concept to use memory cells directly for computing 20 years ago was considered crazy,” said Jian-Ping Wang, the senior author of the paper and a Distinguished McKnight Professor in the Department of Electrical and Computer Engineering.
Related Stories
Wang credited a collaborative interdisciplinary team—comprising experts in physics, materials science, computer science, modeling, and hardware creation—for the successful development and demonstration of this technology.
This research builds on Wang's and his team's earlier work on Magnetic Tunnel Junctions (MTJs), which are nanostructured devices that enhance hard drives, sensors, and other microelectronic systems. These devices also play a crucial role in Magnetic Random Access Memory (MRAM), used in microcontrollers and smartwatches.
The CRAM architecture represents a significant advancement by enabling true computation within memory, thereby eliminating the traditional bottleneck between computation and memory in von Neumann architecture, which underpins most modern computers.
“CRAM is an extremely energy-efficient digital-based in-memory computing substrate,” said Ulya Karpuzcu, an associate professor in the Department of Electrical and Computer Engineering and co-author of the paper. “It is very flexible in that computation can be performed in any location in the memory array.
Accordingly, we can reconfigure CRAM to best match the performance needs of a diverse set of AI algorithms. It is more energy-efficient than traditional building blocks for today’s AI systems.”
Karpuzcu further explained that CRAM performs computations directly within memory cells, utilizing the array structure efficiently and eliminating the need for slow, energy-intensive data transfers. This efficiency is achieved using spintronic devices, which leverage the spin of electrons rather than electrical charge to store data, providing a more efficient alternative to traditional transistor-based chips.
The team plans to collaborate with semiconductor industry leaders, including those based in Minnesota, to conduct large-scale demonstrations and produce the hardware needed to advance AI functionality. This collaboration aims to bring this innovative technology to market, offering a sustainable solution to the growing energy demands of AI applications.
By addressing the critical challenge of energy consumption in AI, the University of Minnesota's research not only paves the way for more efficient AI technologies but also contributes to the broader goal of sustainable technological development.
Note: Materials provided above by The Brighter Side of News. Content may be edited for style and length.
Like these kind of feel good stories? Get The Brighter Side of News' newsletter.
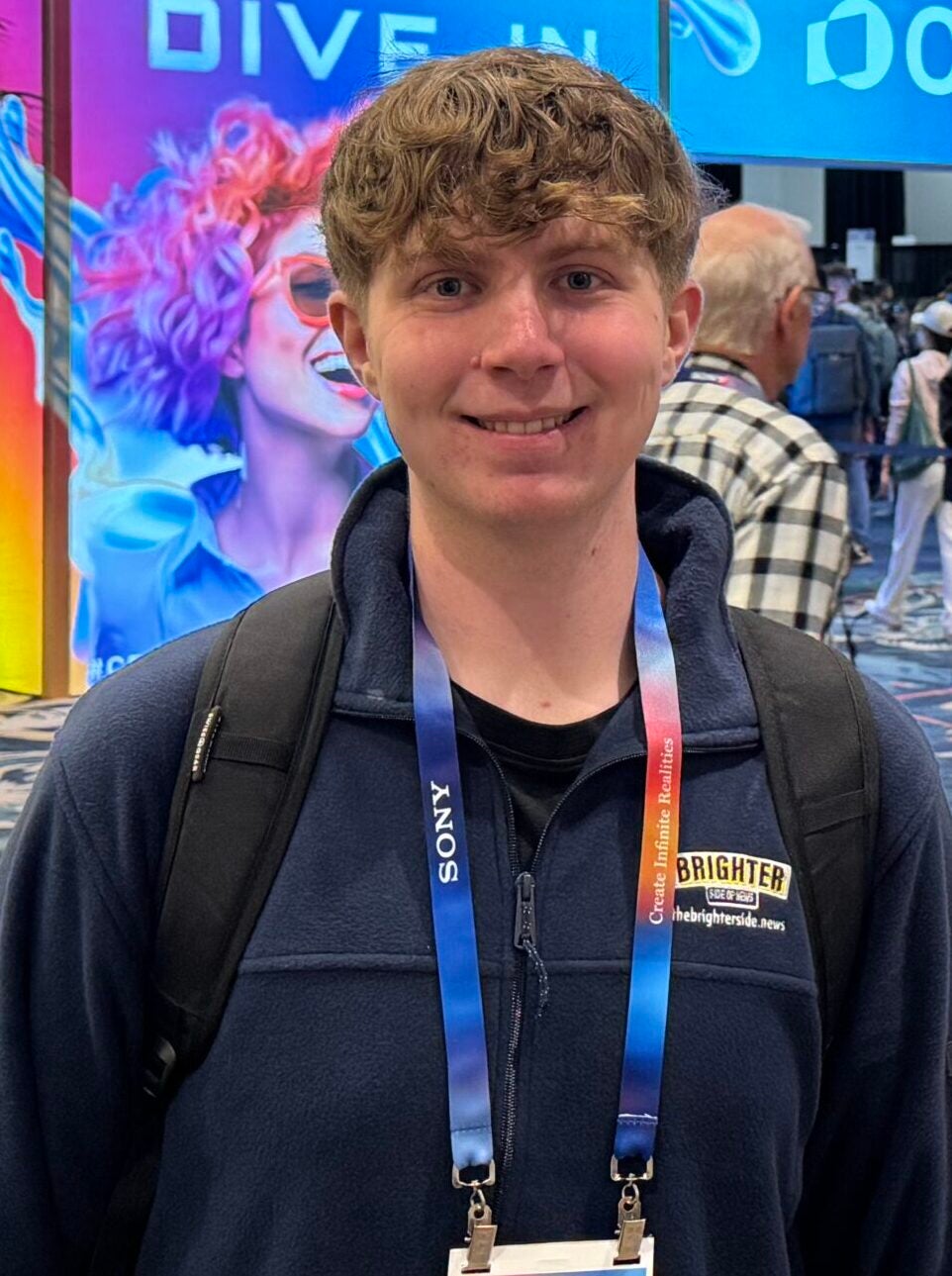
Joshua Shavit
Science & Technology Writer | AI and Robotics Reporter
Joshua Shavit is a Los Angeles-based science and technology writer with a passion for exploring the breakthroughs shaping the future. As a contributor to The Brighter Side of News, he focuses on positive and transformative advancements in AI, technology, physics, engineering, robotics and space science. Joshua is currently working towards a Bachelor of Science in Business Administration at the University of California, Berkeley. He combines his academic background with a talent for storytelling, making complex scientific discoveries engaging and accessible. His work highlights the innovators behind the ideas, bringing readers closer to the people driving progress.