AI can’t think like we do – here’s what that means for the future
A new study finds GPT models lack robust reasoning, failing simple analogy tests when problems are slightly changed.
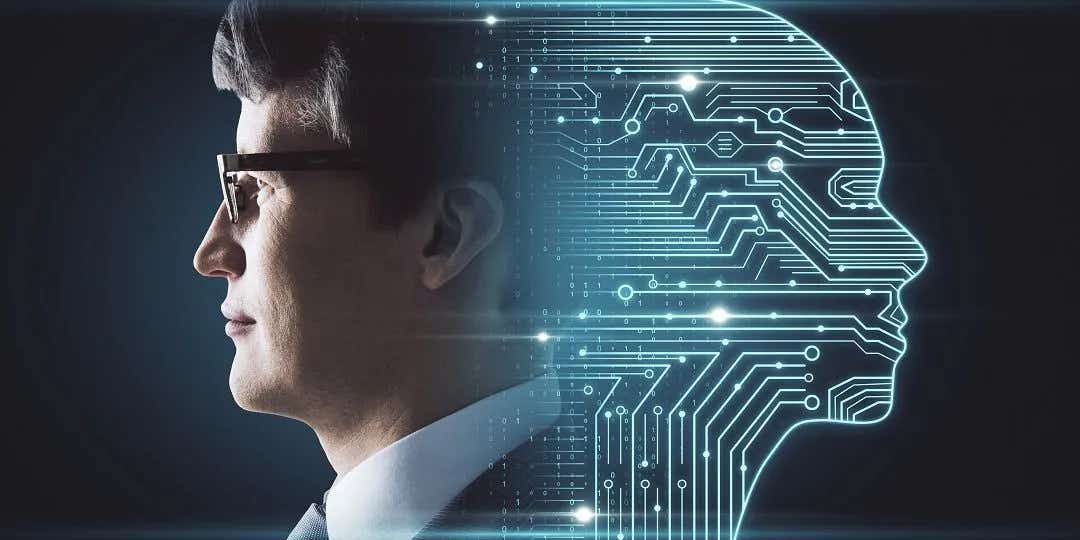
GPT models struggle with analogical reasoning, revealing key limits in AI’s ability to think like humans. (CREDIT: CC BY-SA 4.0)
When artificial intelligence solves a puzzle, it can look like real thinking. Large language models such as GPT-4 have shown impressive results on tests that measure reasoning. But a new study, published in the journal Transactions on Machine Learning Research, suggests that these AI models may not be thinking the way people do. Instead of forming deep connections and abstract ideas, they may just be spotting patterns from their training data.
This difference matters. AI is increasingly being used in areas like education, medicine, and law, where real understanding—not just pattern-matching—is crucial.
Researchers Martha Lewis, from the University of Amsterdam, and Melanie Mitchell, from the Santa Fe Institute, set out to test how well GPT models handle analogy problems, especially when those problems are changed in subtle ways. Their results reveal key weaknesses in the reasoning skills of these AI systems.
What It Means to Reason by Analogy
Analogical reasoning helps you understand new situations by comparing them to things you already know. For example, if “cup is to coffee” as “bowl is to soup,” you’ve made an analogy by comparing how a container relates to its contents.
These types of problems are important because they test abstract thinking. You’re not just recalling facts—you’re identifying relationships and applying them to new examples. Humans are naturally good at this. But AI? Not so much.
To find out where AI stands, Lewis and Mitchell tested GPT models and humans on three kinds of analogy problems: letter strings, digit matrices, and story analogies.
In each test, the researchers first gave the original version of a problem. Then they introduced a modified version—designed to require the same kind of reasoning but harder to match to anything the AI might have seen during training. A robust thinker should do just as well on the variation. Humans did. AI did not.
Letter Patterns Reveal AI’s Limits
Letter-string problems are often simple, like this: If “abcd” changes to “abce,” what should “ijkl” change to? Most people would say “ijkm,” and so do GPT models. But when the pattern becomes slightly more abstract—like removing repeated letters—the AI starts to fail.
For example, in the problem “abbcd” becomes “abcd,” and the goal is to apply the same rule to “ijkkl.” Most humans figure out the repeated letter is being removed, so they answer “ijkl.” GPT-4, however, often gives the wrong answer.
Related Stories
“These models tend to perform poorly when the patterns differ from those seen in training,” Lewis explained. “They may mimic human answers on familiar problems, but they don’t truly understand the logic behind them.”
Two kinds of variations were tested.
- Researchers changed the order or position of letters.
- They replaced letters with non-letter symbols.
In both cases, humans kept getting the answers right, but GPT models struggled. The problem worsened when the patterns were novel or unfamiliar.
When Numbers Confuse the Machine
The study also looked at digit matrices, where a person—or an AI—must find a missing number based on a pattern in a grid. Think of it like Sudoku but with a twist.
One version tested how the AI would react if the missing number wasn’t always in the bottom-right spot. Humans had no problem adjusting. GPT models, however, showed a steep drop in performance.
In another version, researchers replaced numbers with symbols. This time, neither humans nor the AI had much trouble. But the fact that GPT’s accuracy fell so sharply in the first variation suggests it was relying on fixed expectations—not flexible reasoning.
“These findings show that AI is often tied to specific formats,” Mitchell noted. “If you shift the layout even a little, it falls apart.”
Stories That Don’t Stick
The third type of analogy problem was based on short stories. The test asked both humans and GPT models to read a story and choose the most similar one from two options. This tests more than pattern-matching; it requires understanding how events relate.
Once again, GPT models lagged behind. They were affected by the order in which answers were given—something humans ignored. The models also struggled more when stories were reworded, even if the meaning stayed the same. This suggests a reliance on surface details rather than deeper logic.
“These models tend to favor the first answer given, even when it’s incorrect,” the researchers noted. “They also rely heavily on the phrasing used, showing that they lack a true grasp of the content.”
This matters because real-life decisions aren’t always spelled out the same way every time. In law, for instance, a small change in wording can hide or reveal a critical detail. If an AI system can’t spot that change, it could lead to errors.
Fragile Thinking in a High-Stakes World
One key takeaway from the study is that GPT models lack what’s known as “zero-shot reasoning.” That means they struggle when they’re asked to solve problems they haven’t seen before, even if those problems follow logical rules. Humans, on the other hand, are good at spotting those rules and applying them in new situations.
As Lewis put it, “We can abstract from patterns and apply them broadly. GPT models can’t do that—they’re stuck matching what they’ve already seen.”
This gap between AI and human reasoning isn’t just academic. It affects how AI performs in high-stakes areas like courtrooms or hospitals. For instance, legal systems rely on analogies to interpret laws. If a model fails to recognize how a precedent applies to a new case, the results could be serious.
AI also plays a growing role in education, offering tutoring and feedback. But if it lacks a true understanding of concepts, it might mislead rather than help students. In health care, it could misinterpret patient records or treatment guidelines if the format is unfamiliar.
A Call for Better Testing
The study’s authors stress that high scores on standard benchmarks don’t tell the whole story. AI systems can seem smart on the surface but fail when asked to reason in flexible ways. This means tests need to go beyond accuracy—they must measure robustness.
In one experiment, GPT models chose incorrect answers simply because the format had changed slightly. In another, their performance dropped when unfamiliar symbols replaced familiar ones. These changes shouldn’t matter to a system that truly understands analogies. But they do.
“The ability to generalize is essential for safe and useful AI,” Lewis said. “We need to stop assuming that high benchmark scores mean deep reasoning. They often don’t.”
Pattern-Matching Isn’t Understanding
AI researchers have long known that models work best when trained on large amounts of data. The more examples a system sees, the better it becomes at spotting patterns. But spotting isn’t the same as thinking.
As Lewis pointed out, “It’s less about what’s in the data and more about how the system uses it.”
In the end, this study reminds us that AI can be helpful—but it isn’t a stand-in for human thinking. When problems are new, vague, or complex, people still do better. And that matters in the real world, where no two problems are ever exactly the same.
Note: The article above provided above by The Brighter Side of News.
Like these kind of feel good stories? Get The Brighter Side of News' newsletter.
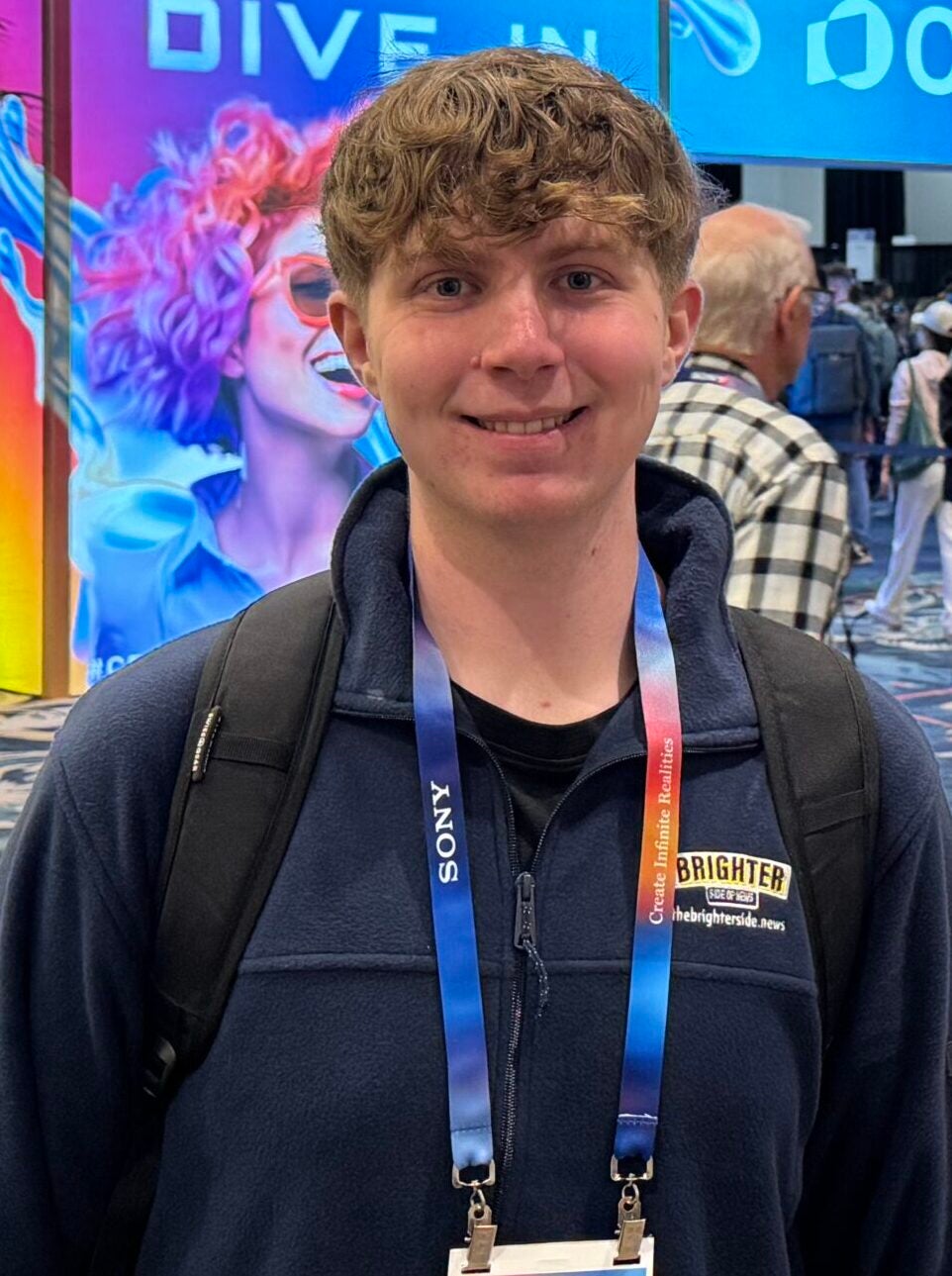
Joshua Shavit
Science & Technology Writer | AI and Robotics Reporter
Joshua Shavit is a Los Angeles-based science and technology writer with a passion for exploring the breakthroughs shaping the future. As a contributor to The Brighter Side of News, he focuses on positive and transformative advancements in AI, technology, physics, engineering, robotics and space science. Joshua is currently working towards a Bachelor of Science in Business Administration at the University of California, Berkeley. He combines his academic background with a talent for storytelling, making complex scientific discoveries engaging and accessible. His work highlights the innovators behind the ideas, bringing readers closer to the people driving progress.