AI can predict binge eating and drinking
A new study uses advanced techniques in machine learning and artificial intelligence to develop prediction models for binge eating and drinking in everyday life.
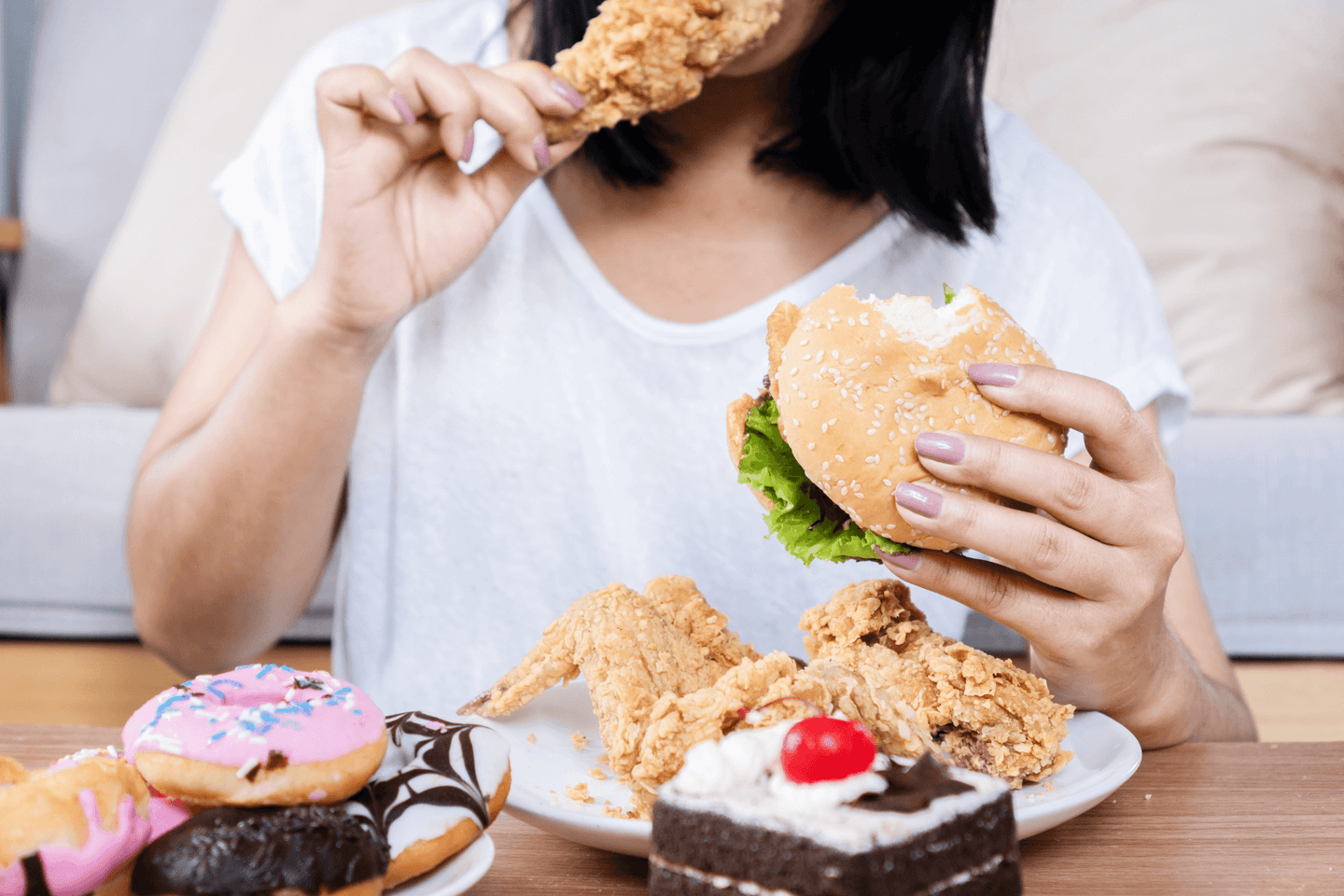
Binge Eating (CREDIT:
Behavioral Health Clinic)
A new study by KU Leuven’s Mind-Body Research Group and the University of California, Berkeley reveals factors driving binge eating and drinking in people with bulimia nervosa and alcohol use disorder. Published in Psychological Medicine, this research uses machine learning and artificial intelligence to create models predicting these behaviors, aiming to make treatments more effective.
For this study, 120 patients were observed over 12 months, tracking their eating and drinking habits along with various emotional, behavioral, and contextual factors. The experience sampling method (ESM) was employed, where participants recorded their actions and feelings eight times a day on selected weekdays.
This extensive data collection allowed the researchers to identify patterns and predictors of problematic eating and drinking behaviors. Using machine learning and artificial intelligence, they developed two types of models: group-level and individual-level.
Generally, the group-level models, which combined data from multiple patients, outperformed the individual-level models. Key predictors for binge eating and drinking included the time of day and the perception of an urge to eat or drink. Social context and emotional factors also played significant roles.
The insights from this study support just-in-time adaptive interventions (JITAI), offering timely assistance when patients need it most. Researcher Nicholas Leenaerts explains, "These insights are an important step towards understanding and addressing complexity in bulimia nervosa and alcohol use disorder. By using artificial intelligence, we can better predict when binge eating and drinking will occur, and step in when needed."
One practical application could involve a smartphone app where patients report their emotions and behaviors. An algorithm would then predict the risk of binge eating or drinking. When the risk is high, the app could send a tailored warning to the patient. This detailed approach could significantly enhance the effectiveness of treatments for bulimia nervosa and alcohol use disorder.
Related Stories
Understanding the specific context of binge eating and drinking is crucial. The study found that social context and emotional state varied significantly between these behaviors. Positive emotions and social situations were more likely to predict binge drinking, while negative emotions were often linked to binge eating.
Leenaerts emphasizes, "Our research shows that it is crucial to understand the context in which the behavior occurs. This knowledge allows us to develop interventions that are not only timed well but are also relevant in the context and take the emotional state of the patient into account."
By recognizing these patterns, you can better comprehend the complexity of bulimia nervosa and alcohol use disorder. The predictive power of artificial intelligence offers a promising avenue for improving patient outcomes. Tailored, real-time interventions could provide critical support exactly when it’s needed, addressing the unique circumstances and emotional states that drive binge behaviors.
This research marks a significant advancement in the treatment of these disorders. The ability to predict and intervene in real-time based on individual data sets a new standard for personalized healthcare. Patients and healthcare providers can leverage these insights to develop more effective, context-aware treatment plans.
The study not only enhances understanding but also opens the door to innovative treatment strategies that could significantly improve the quality of life for those affected by bulimia nervosa and alcohol use disorder.
By focusing on individual needs and the specific contexts of binge behaviors, you can play a pivotal role in the treatment process. This approach underscores the importance of personalized care and the potential of technology to transform healthcare.
As these predictive models become more refined, they hold the promise of making treatments more responsive and effective, ultimately helping patients lead healthier, more stable lives.
Note: Materials provided above by The Brighter Side of News. Content may be edited for style and length.
Like these kind of feel good stories? Get The Brighter Side of News' newsletter.
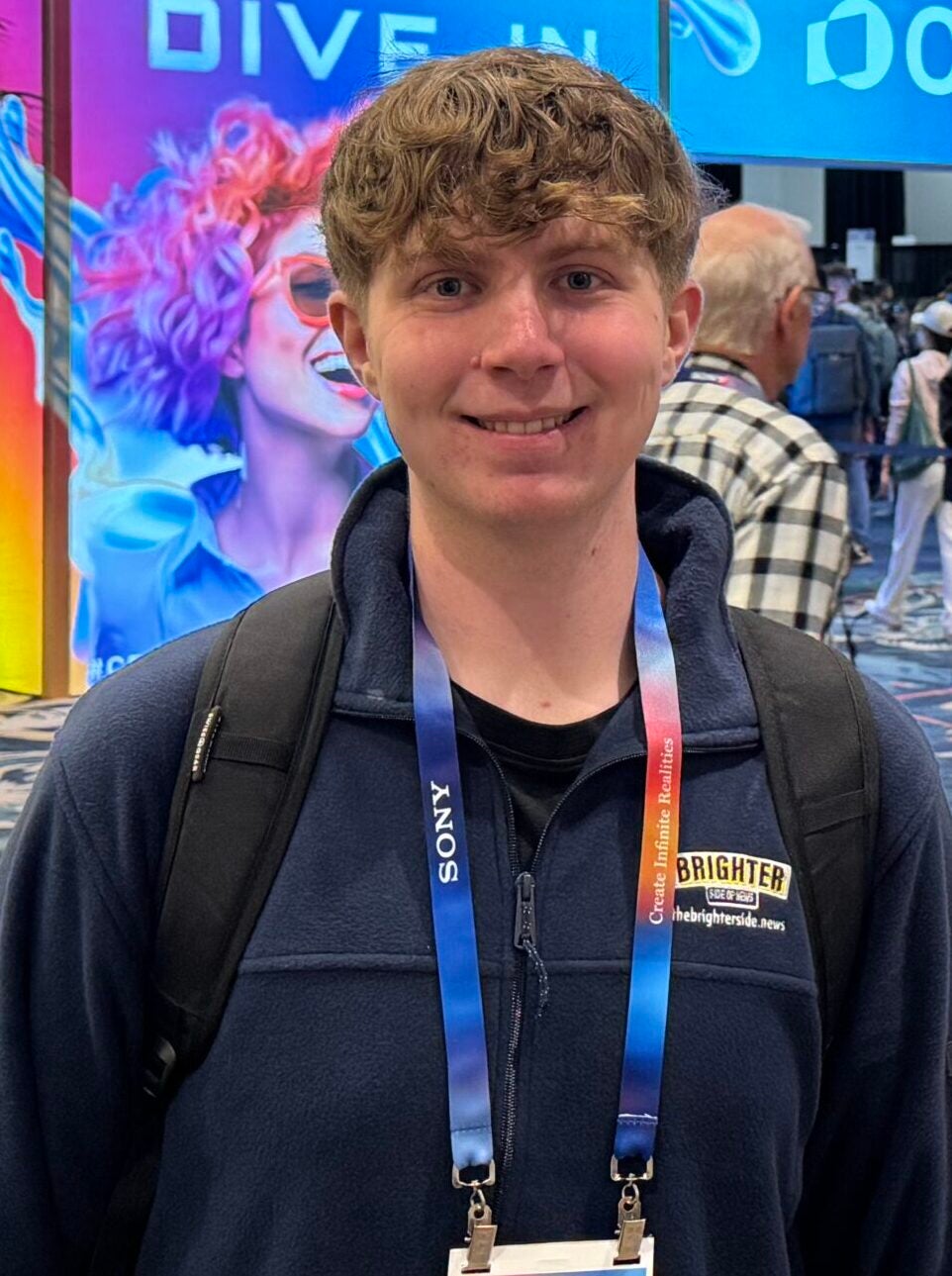
Joshua Shavit
Science & Technology Writer | AI and Robotics Reporter
Joshua Shavit is a Los Angeles-based science and technology writer with a passion for exploring the breakthroughs shaping the future. As a contributor to The Brighter Side of News, he focuses on positive and transformative advancements in AI, technology, physics, engineering, robotics and space science. Joshua is currently working towards a Bachelor of Science in Business Administration at the University of California, Berkeley. He combines his academic background with a talent for storytelling, making complex scientific discoveries engaging and accessible. His work highlights the innovators behind the ideas, bringing readers closer to the people driving progress.