AI breakthrough offers non-invasive brain cancer screening
AI predicts brain cancer spread with 85% accuracy using MRI scans, paving the way for noninvasive diagnostics and personalized treatments.
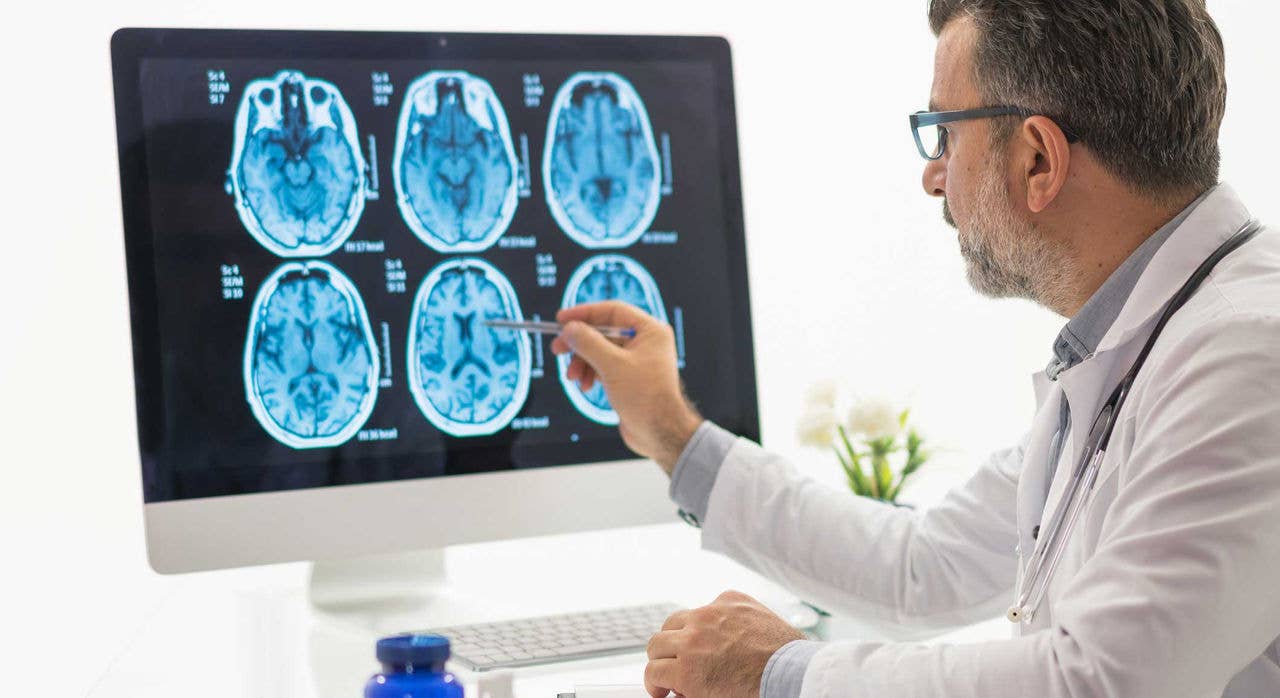
AI-powered MRI analysis predicts brain cancer invasion with 85% accuracy, offering groundbreaking tools for noninvasive diagnosis and improved care. (CREDIT: CC BY-SA 4.0)
The spread of metastatic brain cancer presents one of the most challenging hurdles in oncology, as it often leads to poor outcomes and limited treatment options. Recent advancements in artificial intelligence (AI) and machine learning (ML) are providing a glimpse into a future where noninvasive tools could play a pivotal role in diagnosing and managing these aggressive tumors.
Published in the journal, Neuro-Oncology Advances, researchers have developed a machine learning model that can predict brain metastasis invasion patterns (BMIP) using features extracted from MRI scans. This innovation holds the potential to replace invasive diagnostic procedures with a more patient-friendly alternative.
Brain metastases—cancerous growths originating from other parts of the body—often spread to surrounding healthy brain tissue, making treatment complex and prognosis dire. Approximately 50% to 64% of these metastases are classified as highly invasive, meaning cancer cells infiltrate brain tissue beyond the main tumor site. Such cases are associated with shorter survival and higher recurrence rates.
The new model’s development utilized a retrospective dataset of over 130 patients with surgically resected brain metastases, obtained from the Montreal Neurological Institute-Hospital. Researchers split these cases into training and testing subsets, ensuring no data overlap to maintain the integrity of results. The AI model achieved an impressive 85% accuracy and a 90% F1 score when predicting BMIP.
The model’s success stems from its ability to analyze nuanced features in MRI scans. Preoperative scans were processed using advanced normalization tools to align data from different MRI machines, ensuring consistency. Researchers utilized T2-weighted (T2W) and contrast-enhanced T1-weighted (T1WC+) sequences, key for delineating tumor boundaries and associated edema—a hallmark of tumor invasion.
Manual segmentation of tumors and surrounding edema formed the foundation of the dataset. Expert neuroradiologists refined these initial contours, ensuring precision by removing imaging artifacts and accounting for leptomeningeal invasion. Computational algorithms then generated additional regions of interest, isolating tumor and edema zones. These steps enabled the model to capture subtle features indicative of cancer spread.
Radiomic features—quantitative data derived from medical images—played a pivotal role. Researchers extracted 107 features, such as texture and shape metrics, from segmented regions. To prevent overfitting, the feature set was narrowed down to the ten most predictive attributes using statistical techniques. Image-level standardization and feature-level normalization further enhanced model performance by mitigating variations in scan quality and machine calibration.
This approach not only provided accurate results but also highlighted the importance of co-registering different MRI sequences. Aligning T2W and T1WC+ data ensured that features were consistently extracted across imaging modalities, preserving the integrity of the dataset.
Two complementary approaches, traditional machine learning (TML) and convolutional deep learning (CDL), were employed. TML methods included support vector classifiers, random forests, and multi-layer perceptrons. Meanwhile, CDL utilized EfficientNet, a cutting-edge architecture for image-based learning. Ensemble methods aggregated predictions from both approaches, leveraging their strengths for optimal accuracy.
Related Stories
The predictive model’s utility extends beyond merely identifying invasive patterns. By discerning tumor-associated changes in brain parenchyma, it offers insights that could guide personalized treatment strategies. This capability is particularly vital for patients ineligible for surgery due to tumor location or health constraints.
Training the model required careful handling of data to avoid bias. Researchers employed techniques like cross-validation and grid search to fine-tune model parameters. These methods ensured that the model generalized well across unseen data, reducing the likelihood of overfitting. Moreover, the integration of ensemble methods strengthened predictive accuracy, combining insights from multiple models for robust outcomes.
The model also incorporated hierarchical voting mechanisms, where predictions from individual MRI slices were aggregated at two levels. This multi-tiered approach allowed for more reliable results, accounting for potential inconsistencies in individual slice data. By aggregating predictions at the volume level, the model demonstrated superior performance in distinguishing invasive from non-invasive patterns.
The introduction of this AI model into clinical workflows could revolutionize the management of brain metastases. Current practices rely heavily on histopathological examination of surgical specimens to determine BMIP, a method limited by its invasive nature and dependency on specimen availability. The AI-driven approach bridges this gap, enabling early and accurate detection without the need for surgery.
“Our findings demonstrate the enormous potential of machine learning to improve our understanding of cancer and its treatment,” remarked Dr. Matthew Dankner, a co-lead researcher from McGill University. The AI model’s ability to detect subtle changes often invisible to the human eye highlights its potential to surpass traditional diagnostic methods.
Despite its promise, the technology requires further validation. The study’s dataset, while robust, represents a single institution’s patient population. Expanding the model to incorporate diverse datasets is essential to ensure generalizability. Researchers also aim to refine the model to distinguish between invasive and minimally invasive patterns with even greater precision.
This technology has broader implications for oncology. Noninvasive diagnostics could alleviate the burden on patients who may otherwise require surgery for diagnostic purposes. By identifying BMIP early, clinicians could better tailor treatment strategies, potentially improving outcomes and quality of life for patients with brain metastases.
The model’s efficiency also underscores its scalability. Unlike manual methods, which require significant time and expertise, the AI approach streamlines the diagnostic process. This efficiency could make advanced diagnostics accessible to a wider range of healthcare facilities, including those in resource-limited settings.
Brain metastases are notoriously aggressive and challenging to treat. Advances like this AI model signify a critical shift towards noninvasive diagnostics, offering hope for improved outcomes. “With further development, our AI model could become a part of clinical practice, helping us catch cancer spread within the brain earlier and more accurately,” said Dr. Benjamin Rehany, a radiology resident involved in the project.
Future research will likely focus on integrating this tool with therapeutic decision-making. For instance, identifying BMIP could help stratify patients for clinical trials or tailor treatment plans to individual needs. Additionally, combining AI-driven insights with emerging therapies, such as targeted drugs and immunotherapies, could unlock new avenues in cancer care.
This groundbreaking research was supported by esteemed organizations, including the Canadian Cancer Society and the Canadian Institutes of Health Research. As the study progresses, its implications for noninvasive diagnostics and personalized treatment strategies continue to inspire optimism in the fight against metastatic brain cancer.
Moreover, as AI models become more sophisticated, their applications could extend beyond brain metastases. Similar methodologies might be adapted to other forms of cancer, enabling noninvasive biomarker discovery across diverse oncological conditions. This versatility further underscores the transformative potential of AI in medicine.
Collaboration will be essential to realize the full potential of this technology. Multidisciplinary efforts involving radiologists, oncologists, data scientists, and policymakers will ensure that AI tools are integrated effectively into clinical practice. By addressing challenges such as data standardization, regulatory approval, and ethical considerations, stakeholders can pave the way for widespread adoption.
In summary, the development of an AI-driven model to predict BMIP represents a milestone in cancer diagnostics. By leveraging advanced imaging techniques and machine learning algorithms, researchers have demonstrated that noninvasive tools can achieve remarkable accuracy.
This innovation not only promises to enhance patient care but also sets the stage for future advancements in personalized oncology.
Note: Materials provided above by The Brighter Side of News. Content may be edited for style and length.
Like these kind of feel good stories? Get The Brighter Side of News' newsletter.