AI algorithm detects brain abnormalities to help cure epilepsy
The day is coming – some say has already arrived – when artificial intelligence starts to invent things that its human creators could not.
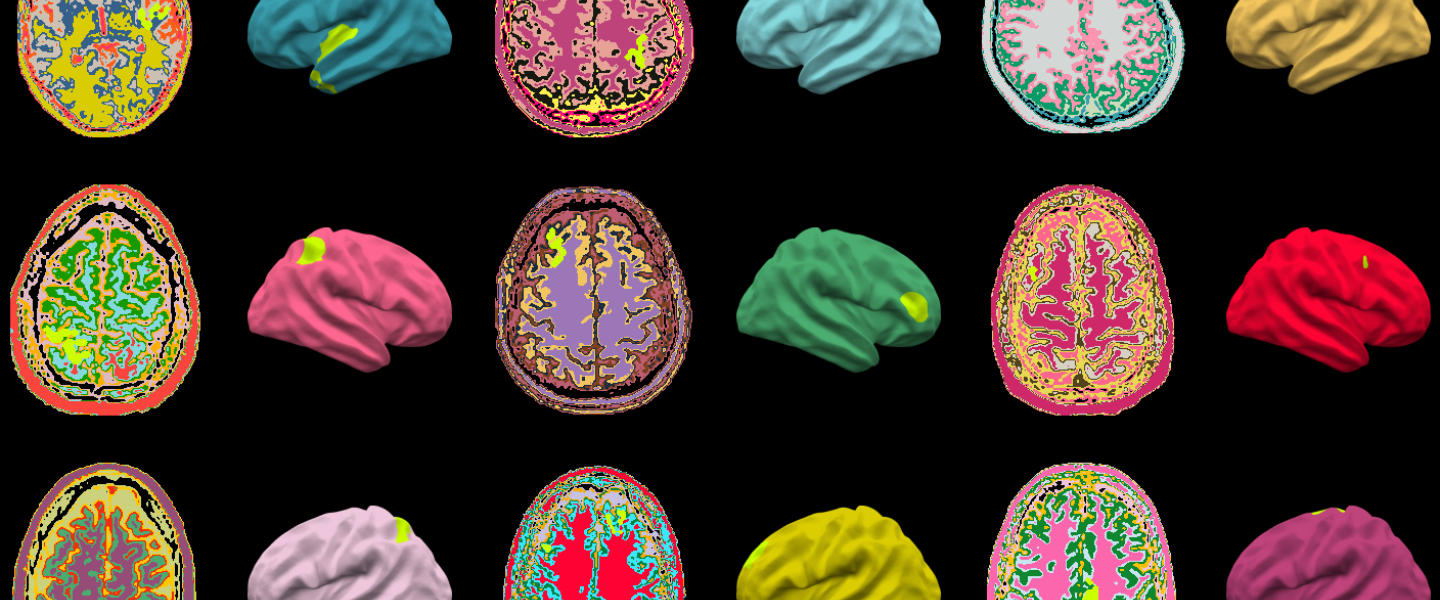
[Aug 16, 2022: Reetta Kälviäinen, University of Eastern Finland]
Brain abnormalities identified by the MELD AI algorithm (highlighted in lime green) on MRI scans of children and adults with epilepsy from around the world. (CREDIT: University of Eastern Finland)
An artificial intelligence (AI) algorithm that can detect subtle brain abnormalities which cause epileptic seizures has been developed by a team of researchers led by University College London. Professor Reetta Kälviäinen’s research group from the University of Eastern Finland and Kuopio University Hospital took part in the study.
The Multicentre Epilepsy Lesion Detection project (MELD) used over 1,000 MRI scans from all over the world to develop the algorithm, which provides reports of where abnormalities are in cases of focal cortical dysplasia (FCD) – a leading cause of epilepsy that is resistant to medication due to unusual cell formations at certain points on the brain.
To do this, the team quantified features from the MRI scans, such as how thick or folded the brain was at around 300,000 locations in each case.
They then trained the AI algorithm on examples labelled by expert radiologists as either being a healthy brain or having FCD – dependant on their patterns and features.
Half of the cohort were used to train the AI and the other half were used to test how well the algorithm performed.
Related Stories
The results, published in Brain, found that in the main cohort of 538 patients the algorithm was able to detect the FCD in 67% of cases.
Previously, 178 of these participants (33%) had been considered MRI negative, which means that radiologists had been unable to find the abnormality – yet the MELD algorithm was able to identify the FCD in 63% of these cases.
This is particularly important, as if doctors can find the abnormality in the brain scan, then surgery to remove it can provide a cure.
Co-first author, Mathilde Ripart (UCL Great Ormond Street Institute of Child Health) said: “We put an emphasis on creating an AI algorithm that was interpretable and could help doctors make decisions. Showing doctors how the MELD algorithm made its predictions was an essential part of that process.”
Co-senior author, Dr Konrad Wagstyl (UCL Queen Square Institute of Neurology) added: "This algorithm could help to find more of these hidden lesions in children and adults with epilepsy, and enable more patients with epilepsy to be considered for brain surgery that could cure the epilepsy and improve their cognitive development. Roughly 440 children per year could benefit from epilepsy surgery in England."
Around 1% of the population have epilepsy and, of these, 20-30% do not respond to medications.
In children who have had surgery to control their epilepsy, FCD is the most common cause, and in adults it is the third most common cause.
Additionally, of patients who have epilepsy that have an abnormality in the brain that cannot be found on MRI scans, FCD is the most common cause.
This study on FCD detection uses the largest MRI cohort of FCDs to date, meaning it is able to detect all types of FCD.
Co-first author, Dr. Hannah Spitzer (Helmholtz Munich) said: “Our algorithm automatically learns to detect lesions from thousands of MRI scans of patients. It can reliably detect lesions of different types, shapes and sizes, and even many of those lesions that were previously missed by radiologists.”
Co-senior author, Sophie Adler (UCL Great Ormond Street Institute of Child Health) added: “We hope that this technology will help to identify epilepsy-causing abnormalities that are currently being missed. Ultimately it could enable more people with epilepsy to have potentially curative brain surgery.”
The MELD FCD classifier tool can be run on any patient with a suspicion of having an FCD who is over the age of 3 years and has an MRI scan.
The MELD project is supported by the Rosetrees Trust.
Study limitations: Different MRI scanners were used at the 22 hospitals involved in the study around the globe, which enables the algorithm to be more robust but might also affect algorithm sensitivity and specificity.
Note: Materials provided above by University of Eastern Finland. Content may be edited for style and length.
Like these kind of feel good stories? Get the Brighter Side of News' newsletter.
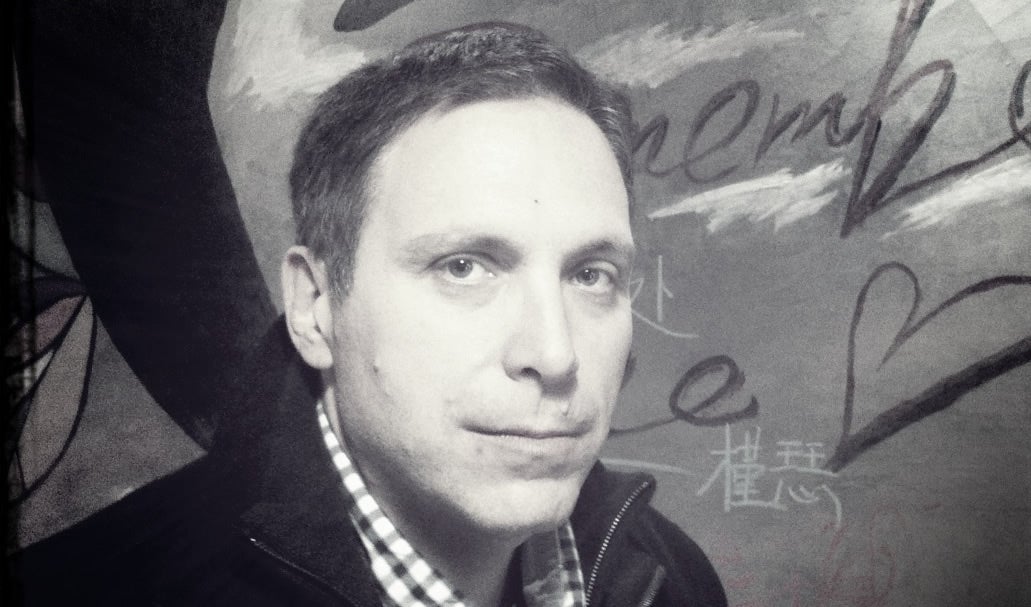
Joseph Shavit
Head Science News Writer | Communicating Innovation & Discovery
Based in Los Angeles, Joseph Shavit is an accomplished science journalist, head science news writer and co-founder at The Brighter Side of News, where he translates cutting-edge discoveries into compelling stories for a broad audience. With a strong background spanning science, business, product management, media leadership, and entrepreneurship, Joseph brings a unique perspective to science communication. His expertise allows him to uncover the intersection of technological advancements and market potential, shedding light on how groundbreaking research evolves into transformative products and industries.