Google’s advanced AI delivers unprecedented accuracy and speed in 15-day weather forecasts
GenCast, an AI model by DeepMind, delivers 15-day forecasts with unparalleled accuracy and speed, aiding preparedness for extreme weather events.
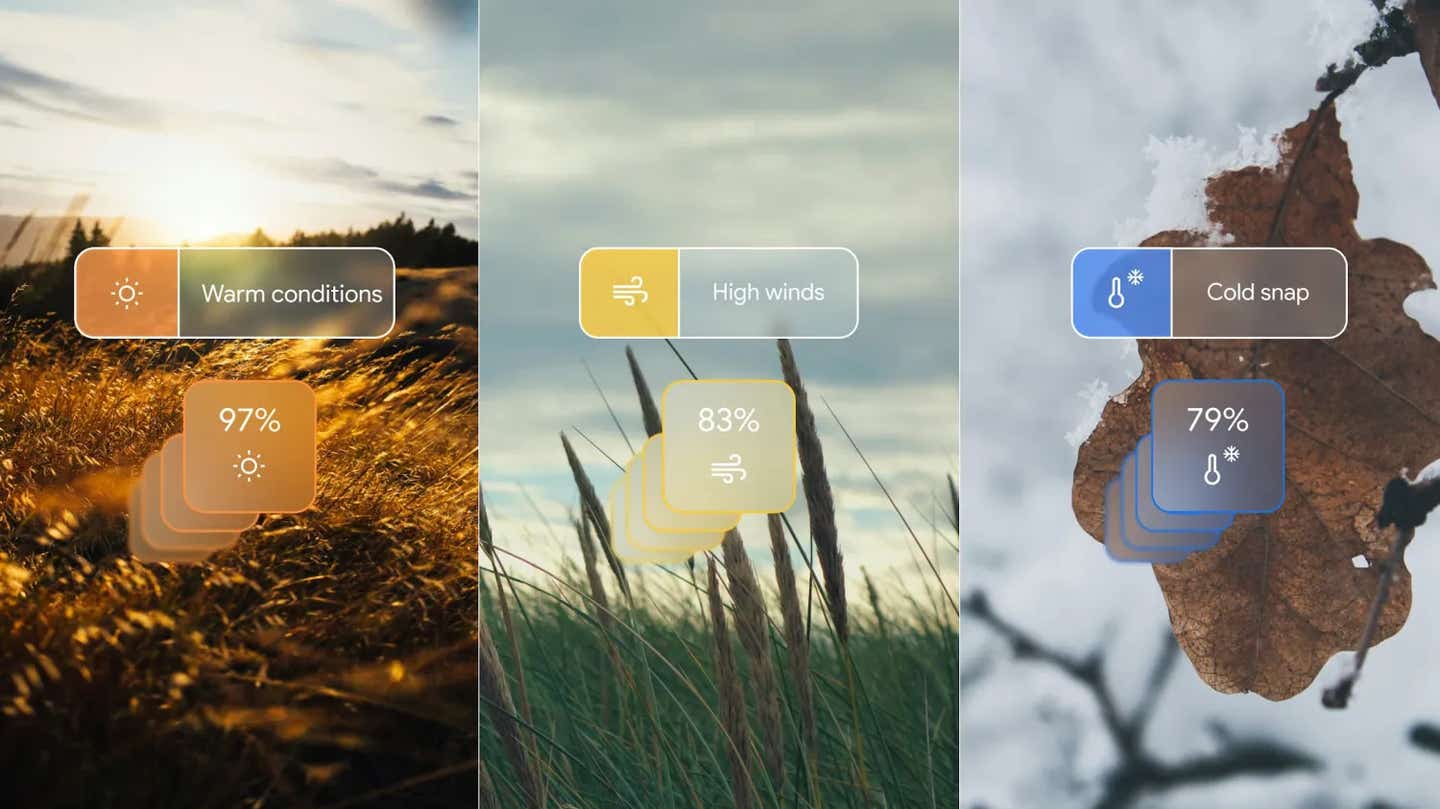
GenCast, an AI-driven weather forecasting model, outperforms traditional systems, offering faster, more accurate predictions and vital insights into extreme weather events. (CREDIT: Google DeepMind)
Weather forecasting shapes critical decisions daily, from carrying an umbrella to preparing for life-threatening cyclones. While forecasts have improved significantly over the years, inherent uncertainties remain due to incomplete weather observations and the complexity of atmospheric physics.
These uncertainties often grow exponentially, making precise long-term predictions challenging. A new artificial intelligence-based weather model, GenCast, may hold the key to unprecedented forecasting accuracy and speed.
Traditional weather forecasting relies on numerical weather prediction (NWP) algorithms, which simulate atmospheric dynamics using mathematical equations.
While NWP methods have served as a global standard for decades, their accuracy is limited by computational complexity and the need for simplifications in modeling. To address this, meteorologists increasingly use ensemble forecasts, which run multiple simulations to provide a range of possible scenarios rather than a single deterministic outcome.
Among these, the European Centre for Medium-Range Weather Forecasting's (ECMWF) Integrated Forecast System (IFS) has long been a global benchmark.
The ECMWF's ensemble forecast system, ENS, exemplifies state-of-the-art probabilistic modeling. Its ensemble members represent sharp, realistic weather trajectories and capture essential spatiotemporal structures, crucial for understanding large-scale phenomena like cyclones.
However, even this advanced system has limitations. Running ENS is computationally intensive, requiring significant time and resources, and it is prone to errors in specific scenarios.
Machine learning (ML) has recently emerged as a promising alternative to traditional weather modeling. ML-based weather prediction (MLWP) methods have demonstrated greater accuracy and efficiency for non-probabilistic forecasts.
Related Stories
These systems are often trained to minimize mean squared error, enabling them to predict the most likely weather outcomes. However, such models typically struggle to represent uncertainty, producing overly generalized forecasts that lack the sharpness of NWP-based methods.
Attempts to integrate MLWP into ensemble forecasts have faced challenges, including unrealistic weather trajectories and lower resolution compared to operational systems like ENS.
GenCast, developed by Google's London-based AI research laboratory DeepMind, represents a breakthrough in weather forecasting.
Described in Nature as the first MLWP method to outperform ENS significantly, GenCast generates realistic weather trajectories that improve marginal and joint forecast distributions over traditional methods. It provides a 15-day forecast with unmatched precision in just eight minutes, compared to hours required by current systems.
Florence Rabier, the ECMWF's director, acknowledged the significance of this advancement, calling it "a leap forward." She emphasized that while GenCast complements existing models, it offers potential for integration into standard forecasting practices.
"We are progressing year by year," Rabier said. "Any new method that can enhance and accelerate this progress is extremely welcome in the context of the extreme societal pressures of climate change."
GenCast's capabilities are especially critical in the context of extreme weather events, which are becoming more frequent and severe due to human-induced climate change.
The model was trained on 40 years of historical data, including temperature, wind speed, and air pressure, enabling it to predict extreme heat, cold, and high winds with remarkable accuracy. In tests against real-world scenarios from 2019, GenCast outperformed ENS in over 97% of cases.
"More accurate forecasts of risks of extreme weather can help officials safeguard more lives, avert damage, and save money," DeepMind stated. These predictions could make a life-saving difference in events like the wildfires in Hawaii in August 2023, which killed approximately 100 people amid criticism over inadequate warnings.
Similarly, GenCast could provide critical lead time in cases like the sudden heat wave in Morocco that claimed 21 lives or Hurricane Helene, which caused 237 fatalities across the southeastern United States.
David Schultz, a professor of synoptic meteorology at Manchester University, expressed optimism about GenCast's future. "I am confident that AI-based weather forecasting systems will continue to incrementally improve, including better prediction of extreme events and their intensity, for which there is a lot of need to improve upon," he said.
However, Schultz noted that these systems remain dependent on existing weather prediction models, such as ECMWF's, for their foundational data.
The implications of GenCast extend beyond emergency preparedness. Its speed and precision could optimize renewable energy management, improve agricultural planning, and enhance everyday decision-making.
By consistently outperforming ENS in predicting both routine and extreme weather events, GenCast sets a new standard in meteorology, offering hope for a future where better forecasts can mitigate the devastating impacts of climate change.
Note: Materials provided above by The Brighter Side of News. Content may be edited for style and length.
Like these kind of feel good stories? Get The Brighter Side of News' newsletter.
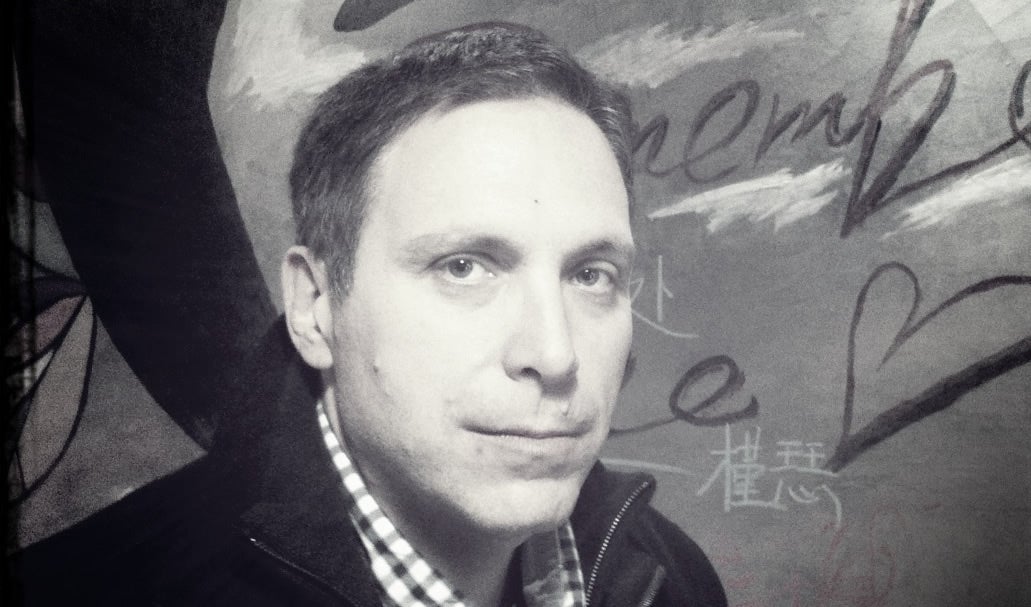
Joseph Shavit
Head Science News Writer | Communicating Innovation & Discovery
Based in Los Angeles, Joseph Shavit is an accomplished science journalist, head science news writer and co-founder at The Brighter Side of News, where he translates cutting-edge discoveries into compelling stories for a broad audience. With a strong background spanning science, business, product management, media leadership, and entrepreneurship, Joseph brings a unique perspective to science communication. His expertise allows him to uncover the intersection of technological advancements and market potential, shedding light on how groundbreaking research evolves into transformative products and industries.